Mutationvalidator: A Computational Method For Variant Cross-Validation In Next-Generation Sequencing Data
CANCER RESEARCH(2014)
摘要
Proceedings: AACR Annual Meeting 2014; April 5-9, 2014; San Diego, CA
Background:
Massively parallel next-generation sequencing (NGS) technologies already enable comprehensive genome-wide detection of germline and somatic variants in thousands of individuals, and the pace of data production keeps accelerating. However, with increased sequencing techniques and variant calling algorithms, proper validation and determination of ground truth is essential for benchmarking, comparisons, and confirmation of novel discoveries. Datasets generated on different sequencing platforms, derived from distinct biological samples and/or using different library construction protocols can provide at least partially independent sources for crosschecking variants. While methods for validation exist to date, they fail to account for the inherent variability in the datasets, and thereby propose biased estimates for the validation rates.
Results:
Here we present a method for variant cross-validation and present software implementation (written in Matlab) that generates validation metrics and diagnostics to characterize a mutation as true somatic, germline, or artifactual. Our method adjusts for the power to observe variant-supporting evidence in the validation dataset, which allows for accurate assessment of validation rates across wide ranges of sequencing coverages and variant allelic fractions. When this method is applied to a test cohort of 172 patients from The Cancer Genome Atlas Lung Adenocarcenoma dataset, we observe a SNP and Indel validation rate of 95% and 96% respectively with a 99% power for detection. A previously implemented naive method of >8% supporting alleles in the tumor and <3% alternate allele in the normal for a validated event would have yielded rates of 72% and 95% for SNPs and Indels, values we claim to be underestimations of the true rates as they misclassify underpowered sites as a false positives.
Conclusions:
Our proposed method, MutationValidator, enables confident classification of mutations using orthogonal next-generation sequencing technologies for validation as it takes into consideration the variability in and across the datasets.
Citation Format: Mara Rosenberg, Gad Getz, Adam Kiezun, Andrey Sivachenko. MutationValidator: A computational method for variant cross-validation in next-generation sequencing data. [abstract]. In: Proceedings of the 105th Annual Meeting of the American Association for Cancer Research; 2014 Apr 5-9; San Diego, CA. Philadelphia (PA): AACR; Cancer Res 2014;74(19 Suppl):Abstract nr 2376. doi:10.1158/1538-7445.AM2014-2376
更多查看译文
AI 理解论文
溯源树
样例
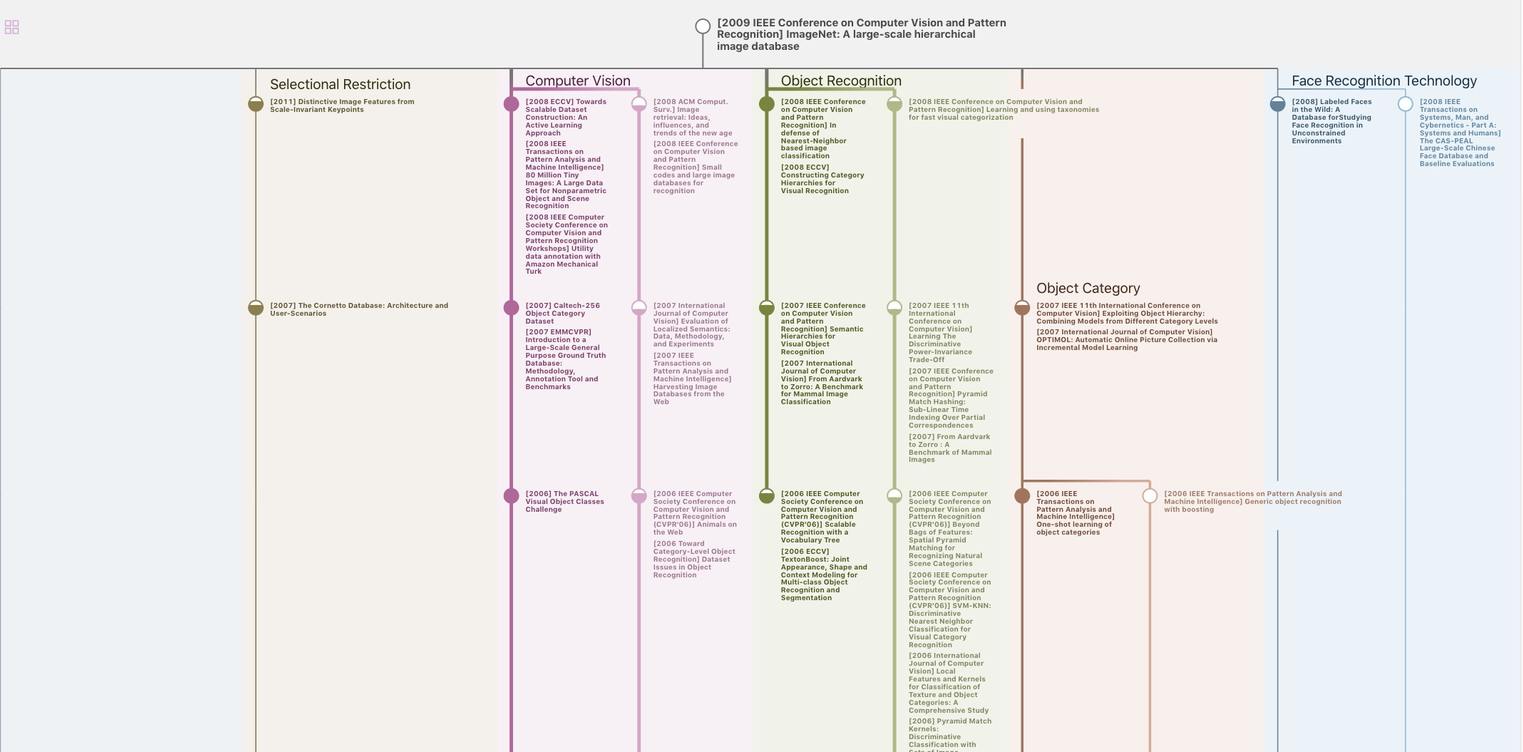
生成溯源树,研究论文发展脉络
Chat Paper
正在生成论文摘要