Bayesian method for simultaneous gross error detection and data reconciliation
AICHE JOURNAL(2015)
摘要
Process measurements collected from daily industrial plant operations are essential for process monitoring, control, and optimization. However, those measurements are generally corrupted by errors, which include gross errors and random errors. Conventionally, those two types of errors were addressed separately by gross error detection and data reconciliation. Solving the simultaneous gross error detection and data reconciliation problem using the hierarchical Bayesian inference technique is focused. The proposed approach solves the following problems in a unified framework. First, it detects which measurements contain gross errors. Second, the magnitudes of the gross errors are estimated. Third, the covariance matrix of the random errors is estimated. Finally, data reconciliation is performed using the maximum a posteriori estimation. The proposed algorithm is applicable to both linear and nonlinear systems. For nonlinear case, the algorithm does not involve any linearization or approximation steps. Numerical case studies are provided to demonstrate the effectiveness of the proposed method. (c) 2015 American Institute of Chemical Engineers AIChE J, 61: 3232-3248, 2015
更多查看译文
关键词
gross error detection,data reconciliation,Bayesian inference,serial strategy
AI 理解论文
溯源树
样例
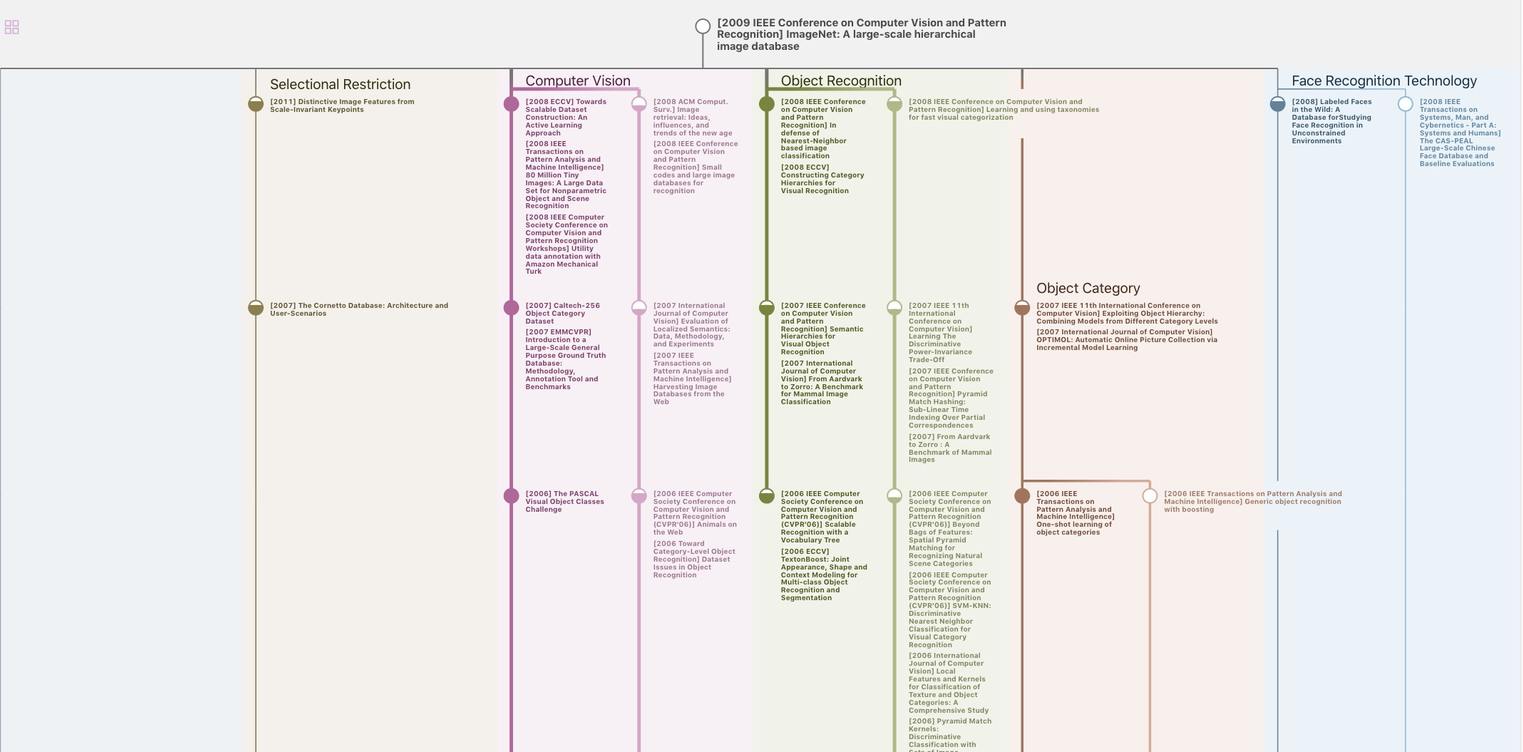
生成溯源树,研究论文发展脉络
Chat Paper
正在生成论文摘要