Dihedral angles principal geodesic analysis using nonlinear statistics
JOURNAL OF APPLIED STATISTICS(2015)
摘要
Statistics, as one of the applied sciences, has great impacts in vast area of other sciences. Prediction of protein structures with great emphasize on their geometrical features using dihedral angles has invoked the new branch of statistics, known as directional statistics. One of the available biological techniques to predict is molecular dynamics simulations producing high-dimensional molecular structure data. Hence, it is expected that the principal component analysis (PCA) can response some related statistical problems particulary to reduce dimensions of the involved variables. Since the dihedral angles are variables on non-Euclidean space (their locus is the torus), it is expected that direct implementation of PCA does not provide great information in this case. The principal geodesic analysis is one of the recent methods to reduce the dimensions in the non-Euclidean case. A procedure to utilize this technique for reducing the dimension of a set of dihedral angles is highlighted in this paper. We further propose an extension of this tool, implemented in such way the torus is approximated by the product of two unit circle and evaluate its application in studying a real data set. A comparison of this technique with some previous methods is also undertaken.
更多查看译文
关键词
principal component analysis,non-Euclidean data,principal geodesic analysis,protein structure,dihedral angles
AI 理解论文
溯源树
样例
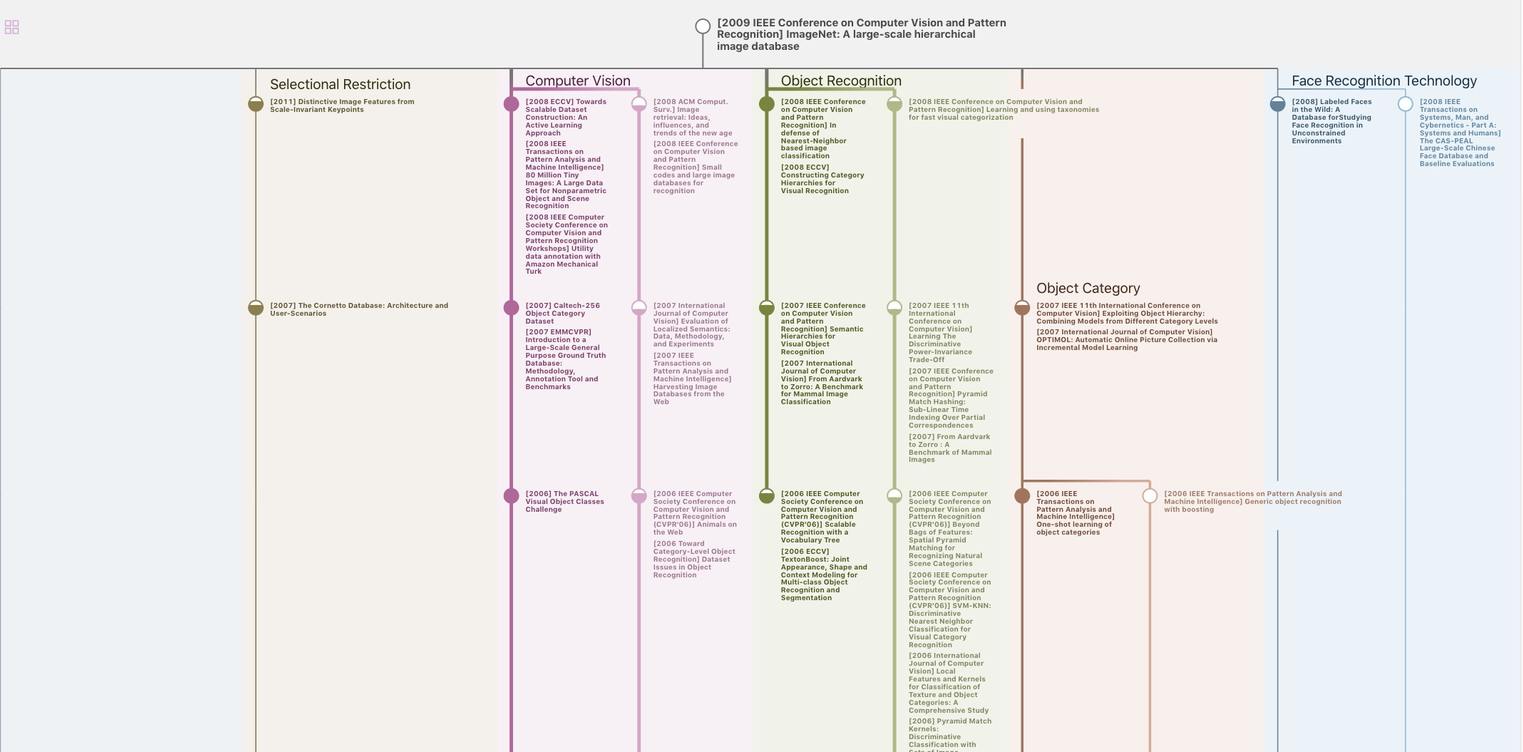
生成溯源树,研究论文发展脉络
Chat Paper
正在生成论文摘要