Robust mixture model cluster analysis using adaptive kernels
JOURNAL OF APPLIED STATISTICS(2013)
摘要
The traditional mixture model assumes that a dataset is composed of several populations of Gaussian distributions. In real life, however, data often do not fit the restrictions of normality very well. It is likely that data from a single population exhibiting either asymmetrical or heavy-tail behavior could be erroneously modeled as two populations, resulting in suboptimal decisions. To avoid these pitfalls, we generalize the mixture model using adaptive kernel density estimators. Because kernel density estimators enforce no functional form, we can adapt to non-normal asymmetric, kurtotic, and tail characteristics in each population independently. This, in effect, robustifies mixture modeling. We adapt two computational algorithms, genetic algorithm with regularized Mahalanobis distance and genetic expectation maximization algorithm, to optimize the kernel mixture model (KMM) and use results from robust estimation theory in order to data-adaptively regularize both. Finally, we likewise extend the information criterion ICOMP to score the KMM. We use these tools to simultaneously select the best mixture model and classify all observations without making any subjective decisions. The performance of the KMM is demonstrated on two medical datasets; in both cases, we recover the clinically determined group structure and substantially improve patient classification rates over the Gaussian mixture model.
更多查看译文
关键词
mixture modeling,non-parametric estimation,unsupervised classification,robust estimation,kernel density estimation
AI 理解论文
溯源树
样例
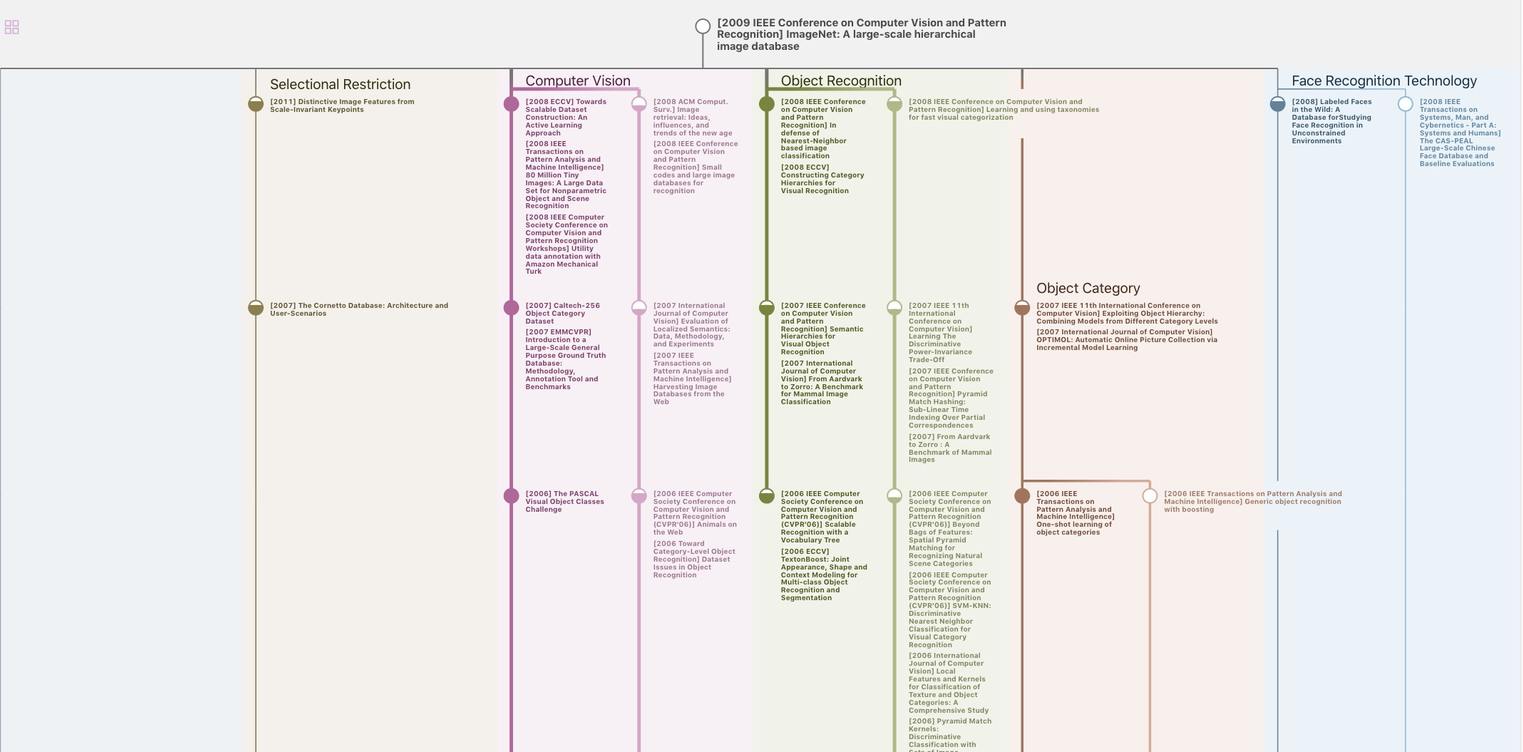
生成溯源树,研究论文发展脉络
Chat Paper
正在生成论文摘要