Multiscale Spatial Decomposition for Skew-Distributed Data with Parallel Spatial Kernel Smoothing
Environment & planning B, Planning & design/Environment and planning B, Planning and design(2014)
摘要
A data-adaptive multiscale spatial decomposition model is proposed to deal with skew-distributed data (eg, population or GDP). Relying on the filtering characteristics according to the bandwidth change of kernel smoothing, the parallel spatial kernel smoothing with different bandwidths is constructed as spatial filter banks for filtering spatial variations at different spatial scales. The filtering residual, a function changing with the spatial scale, is then extracted by parallel spatial kernel smoothing. With a change point detection model based on the second deviation, standard deviation of the residential data is selected for identifying robust significant scales. Then we present the iterative algorithm to extract and remove significant spatial variations at different scales. With well-designed stop criteria, the full hierarchical spatial scale structure in the original spatial process can be adaptively established without assigning the decomposition levels artificially. The computation processes and the statistical and spatial distribution characteristics are demonstrated with case studies of 2003 Chinese population data and GDP data, and the results show that the proposed model is suitable for decomposing the spatial data with spatial heterogeneity. Comparison with the 2D wavelet decomposition suggests that our model has better data-adaptive and shape-preserving ability.
更多查看译文
关键词
multiscale spatial decomposition,spatial kernel smoothing,spatial scale
AI 理解论文
溯源树
样例
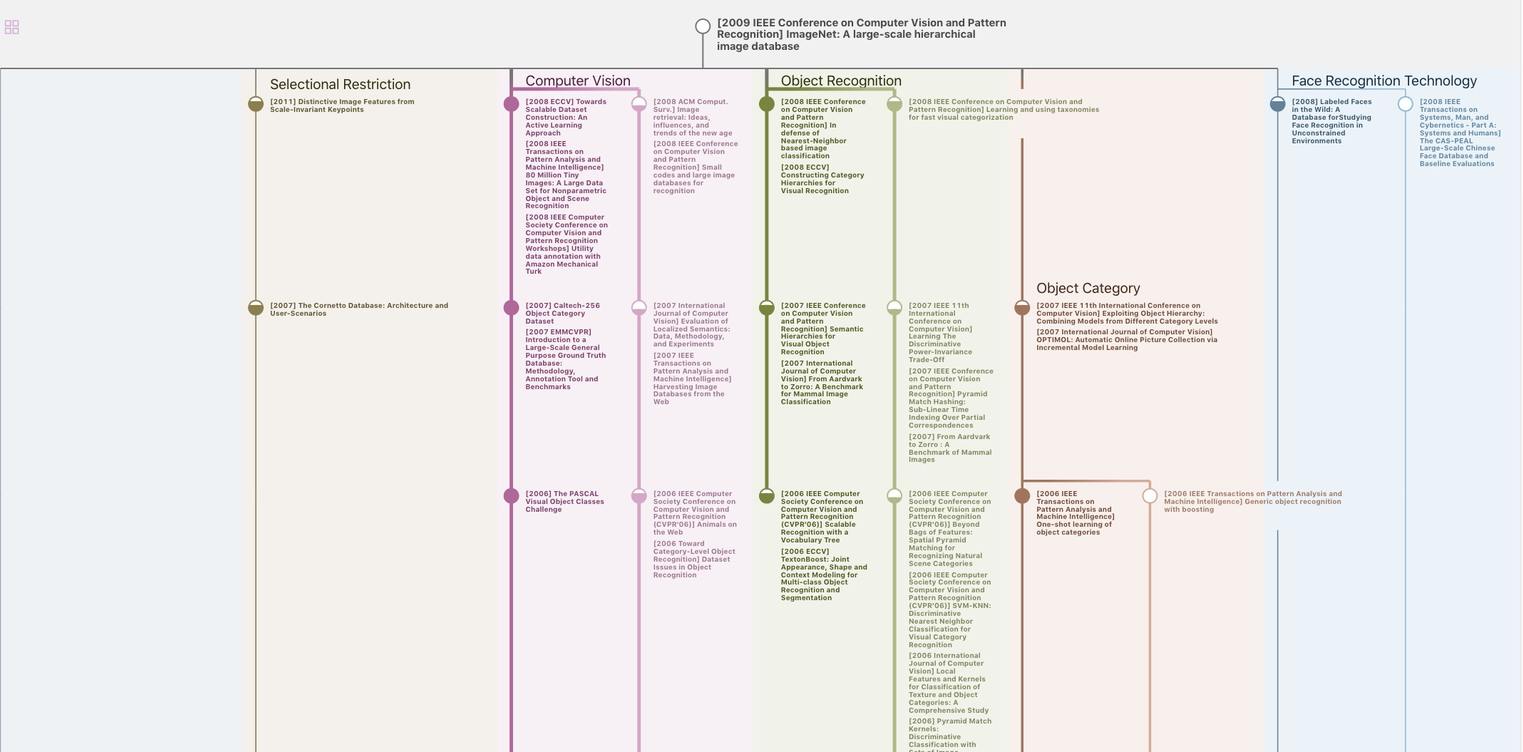
生成溯源树,研究论文发展脉络
Chat Paper
正在生成论文摘要