Dealing with Nuisance Parameters using Machine Learning in High Energy Physics: a Review
arxiv(2020)
摘要
In this work we discuss the impact of nuisance parameters on the effectiveness of machine learning in high-energy physics problems, and provide a review of techniques that may reduce or remove their effect in the search for optimal selection criteria and variable transformations. Nuisance parameters often limit the usefulness of supervised learning in physical analyses due to the degradation of model performances in real data and/or the reduction of their statistical reach. The approaches discussed include nuisance-parametrized models, modified or adversary losses, semi-supervised learning approaches and inference-aware techniques.
更多查看译文
关键词
high energy physics,machine learning,nuisance parameters
AI 理解论文
溯源树
样例
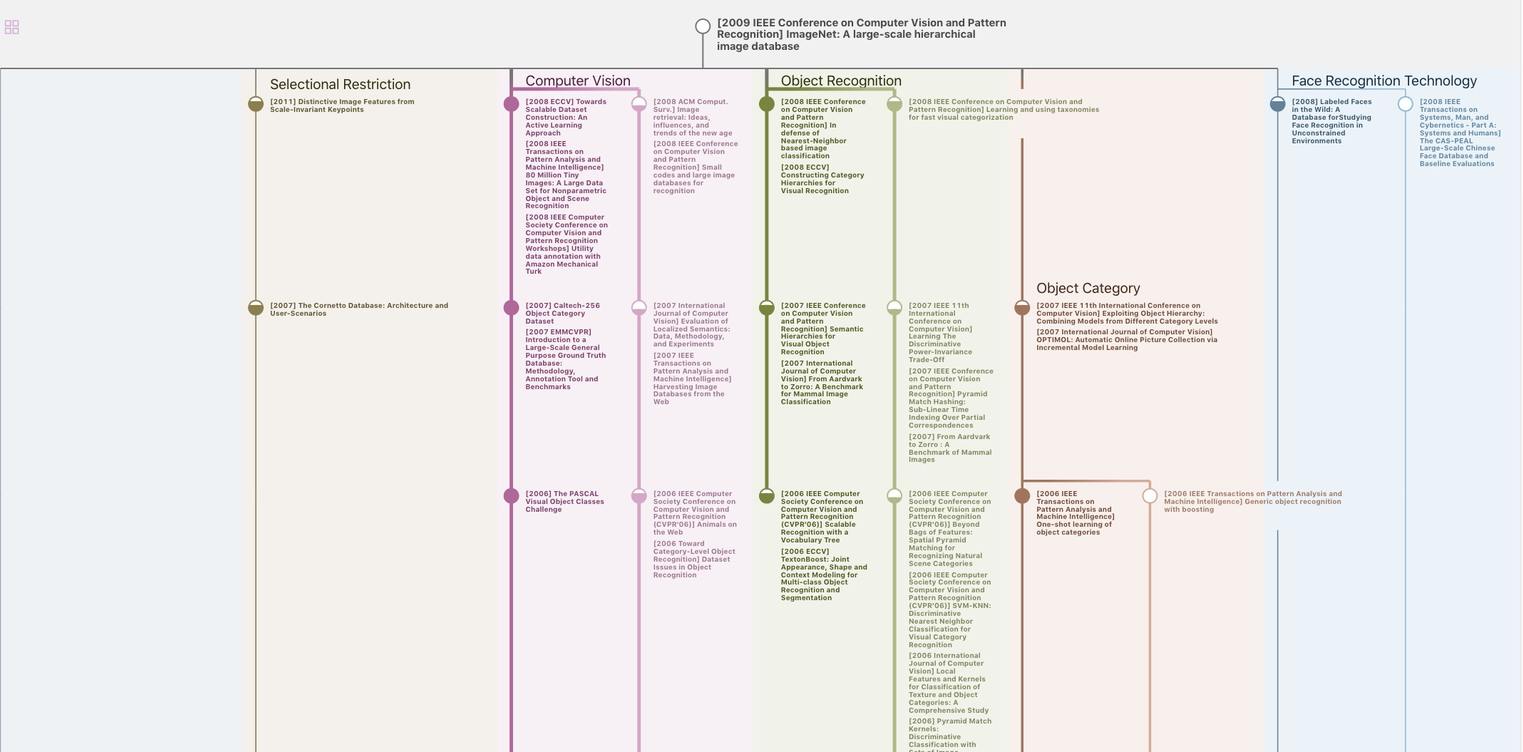
生成溯源树,研究论文发展脉络
Chat Paper
正在生成论文摘要