Opposition chaotic fitness mutation based adaptive inertia weight BPSO for feature selection in text clustering
Applied Soft Computing(2016)
摘要
Graphical abstractDisplay Omitted HighlightsA feature selection method based on binary particle swarm optimization is presented.Fitness based adaptive inertia weight is integrated with the binary particle swarm optimization to dynamically control the exploration and exploitation of the particle in the search space.Opposition and mutation are integrated with the binary particle swarm optimization improve it's search capability.The performance of the clustering algorithm improves with the features selected by proposed method. Due to the ever increasing number of documents in the digital form, automated text clustering has become a promising method for the text analysis in last few decades. A major issue in the text clustering is high dimensionality of the feature space. Most of these features are irrelevant, redundant, and noisy that mislead the underlying algorithm. Therefore, feature selection is an essential step in the text clustering to reduce dimensionality of the feature space and to improve accuracy of the underlying clustering algorithm. In this paper, a hybrid intelligent algorithm, which combines the binary particle swarm optimization (BPSO) with opposition-based learning, chaotic map, fitness based dynamic inertia weight, and mutation, is proposed to solve feature selection problem in the text clustering. Here, fitness based dynamic inertia weight is integrated with the BPSO to control movement of the particles based on their current status, and the mutation and the chaotic strategy are applied to enhance the global search capability of the algorithm. Moreover, an opposition-based initialization is used to start with a set of promising and well-diversified solutions to achieve a better final solution. In addition, the opposition-based learning method is also used to generate opposite position of the gbest particle to get rid of the stagnation in the swarm. To prove effectiveness of the proposed method, experimental analysis is conducted on three different benchmark text datasets Reuters-21578, Classic4, and WebKB. The experimental results demonstrate that the proposed method selects more informative features set compared to the competitive methods as it attains higher clustering accuracy. Moreover, it also improves convergence speed of the BPSO.
更多查看译文
关键词
Text clustering,Binary particle swarm optimization,Mutation,Chaotic map,Opposition-based learning
AI 理解论文
溯源树
样例
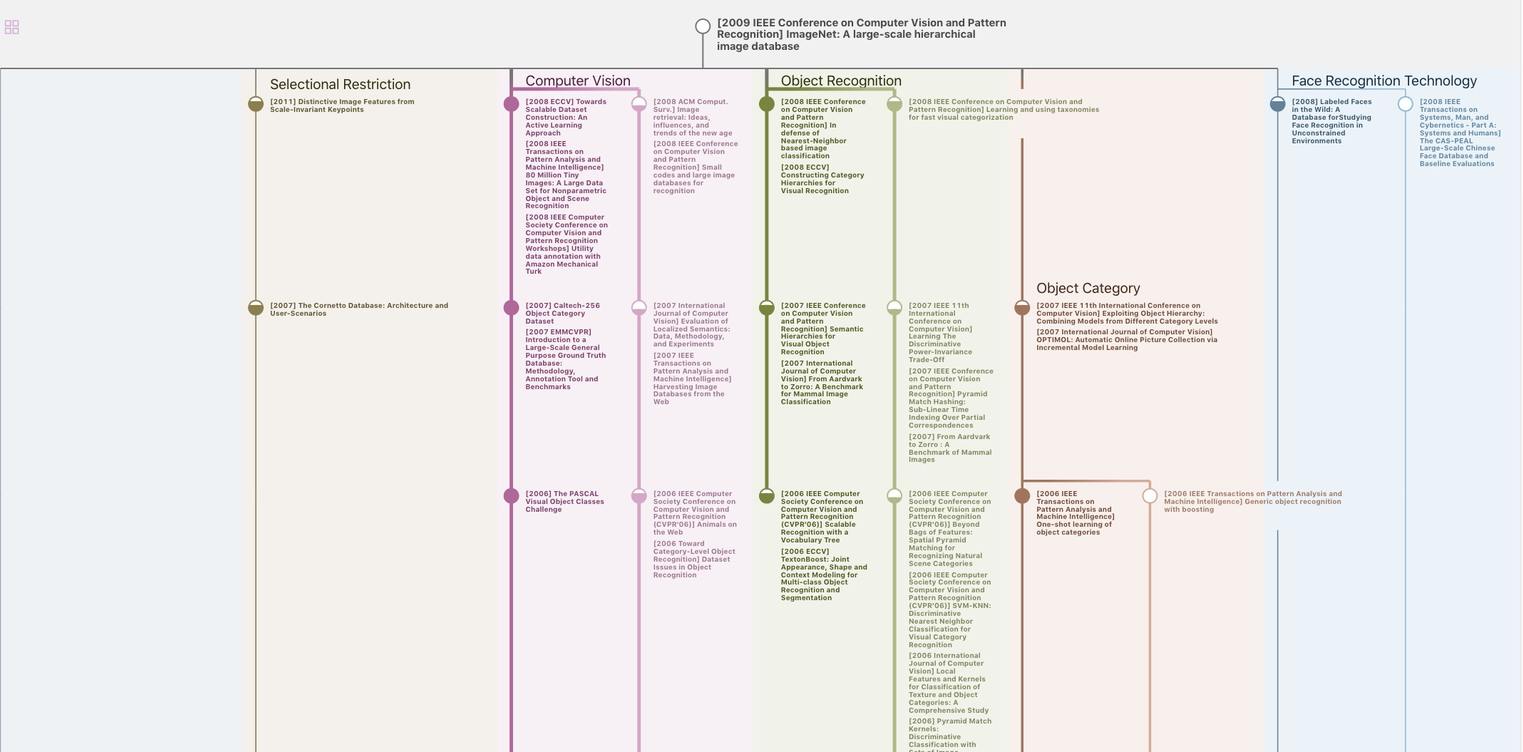
生成溯源树,研究论文发展脉络
Chat Paper
正在生成论文摘要