GSETSK: a generic self-evolving TSK fuzzy neural network with a novel Hebbian-based rule reduction approach.
Applied Soft Computing(2015)
摘要
Takagi-Sugeno-Kang (TSK) fuzzy systems have been widely applied for solving function approximation and regression-centric problems. Existing dynamic TSK models proposed in the literature can be broadly classified into two classes. Class I TSK models are essentially fuzzy systems that are limited to time-invariant environments. Class II TSK models are generally evolving systems that can learn in time-variant environments. This paper attempts to address the issues of achieving compact, up-to-date fuzzy rule bases and interpretable knowledge bases in TSK models. It proposes a novel rule pruning method which is simple, computationally efficient and biologically plausible. This rule pruning algorithm applies a gradual forgetting approach and adopts the Hebbian learning mechanism behind the long-term potentiation phenomenon in the brain. It also proposes a merging approach which is used to improve the interpretability of the knowledge bases. This approach can prevent derived fuzzy sets from expanding too many times to protect their semantic meanings. These two approaches are incorporated into a generic self-evolving Takagi-Sugeno-Kang fuzzy framework (GSETSK) which adopts an online data-driven incremental-learning-based approach. Extensive experiments were conducted to evaluate the performance of the proposed GSETSK against other established evolving TSK systems. GSETSK has also been tested on real world dataset using the high-way traffic flow density and Dow Jones index time series. The results are encouraging. GSETSK demonstrates its fast learning ability in time-variant environments. In addition, GSETSK derives an up-to-date and better interpretable fuzzy rule base while maintaining a high level of modeling accuracy at the same time. (C) 2015 Elsevier B.V. All rights reserved.
更多查看译文
关键词
Self-evolving,Fuzzy neural network,Neuro-fuzzy systems
AI 理解论文
溯源树
样例
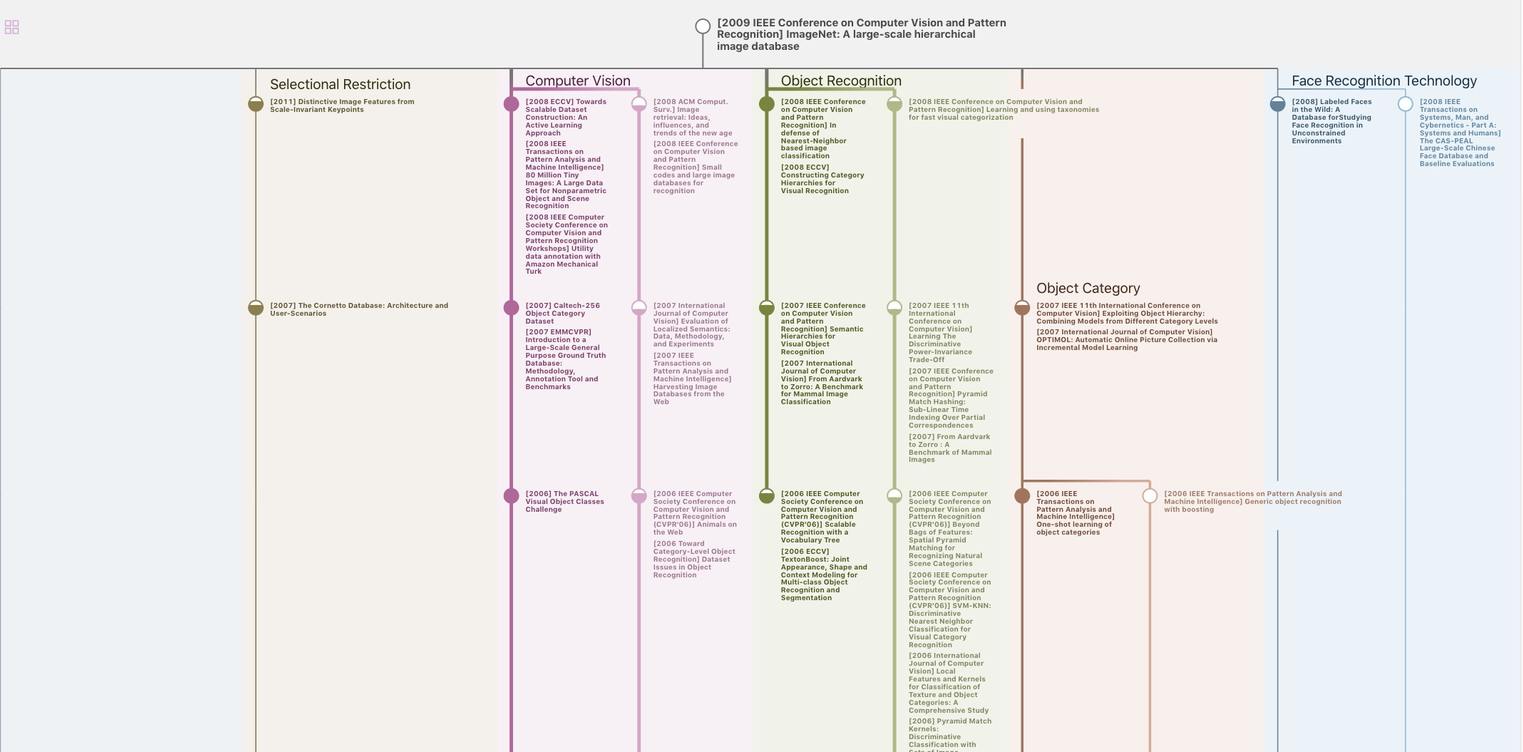
生成溯源树,研究论文发展脉络
Chat Paper
正在生成论文摘要