Estimating A Graphical Intra-Class Correlation Coefficient (Gicc) Using Multivariate Probit-Linear Mixed Models
COMPUTATIONAL STATISTICS & DATA ANALYSIS(2015)
摘要
Data reproducibility is a critical issue in all scientific experiments. In this manuscript, the problem of quantifying the reproducibility of graphical measurements is considered. The image intra-class correlation coefficient (I2C2) is generalized and the graphical intra-class correlation coefficient (GICC) is proposed for such purpose. The concept for GICC is based on multivariate probit-linear mixed effect models. A Markov Chain Monte Carlo EM (mcmcEM) algorithm is used for estimating the GICC. Simulation results with varied settings are demonstrated and our method is applied to the KIRBY21 test-retest dataset. (C) 2015 Elsevier B.V. All rights reserved.
更多查看译文
关键词
Graphical intra class correlation coefficient,Multivariate probit-linear mixed model,MCMCEM
AI 理解论文
溯源树
样例
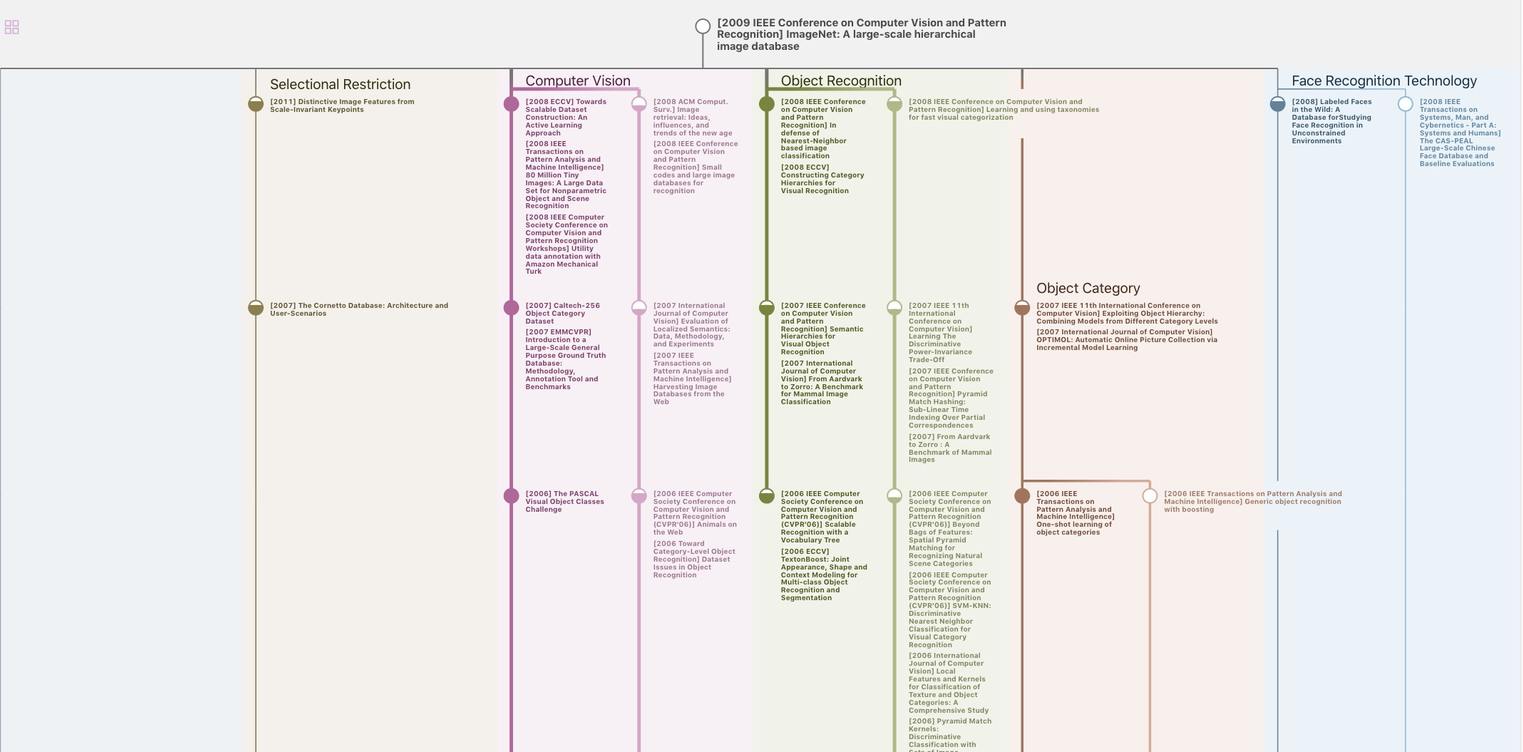
生成溯源树,研究论文发展脉络
Chat Paper
正在生成论文摘要