Exploiting similarity in system identification tasks with recurrent neural networks.
Neurocomputing(2015)
摘要
A novel dual-task learning approach based on recurrent neural networks with factored tensor components for system identification tasks is presented. The goal is to identify the dynamics of a system given few observations which are augmented by auxiliary data from a similar system. The problem is motivated by real-world use cases and a mathematical problem description is given. Further, our proposed model—the factored tensor recurrent neural network (FTRNN)—and two alternative models are introduced which are benchmarked on the cart-pole and mountain car simulations. We show that the FTRNN consistently and significantly outperformed the competing models in accuracy and data-efficiency.
更多查看译文
关键词
Multi-task learning,Recurrent neural network,Factored tensor recurrent neural network,System identification,Dynamical system
AI 理解论文
溯源树
样例
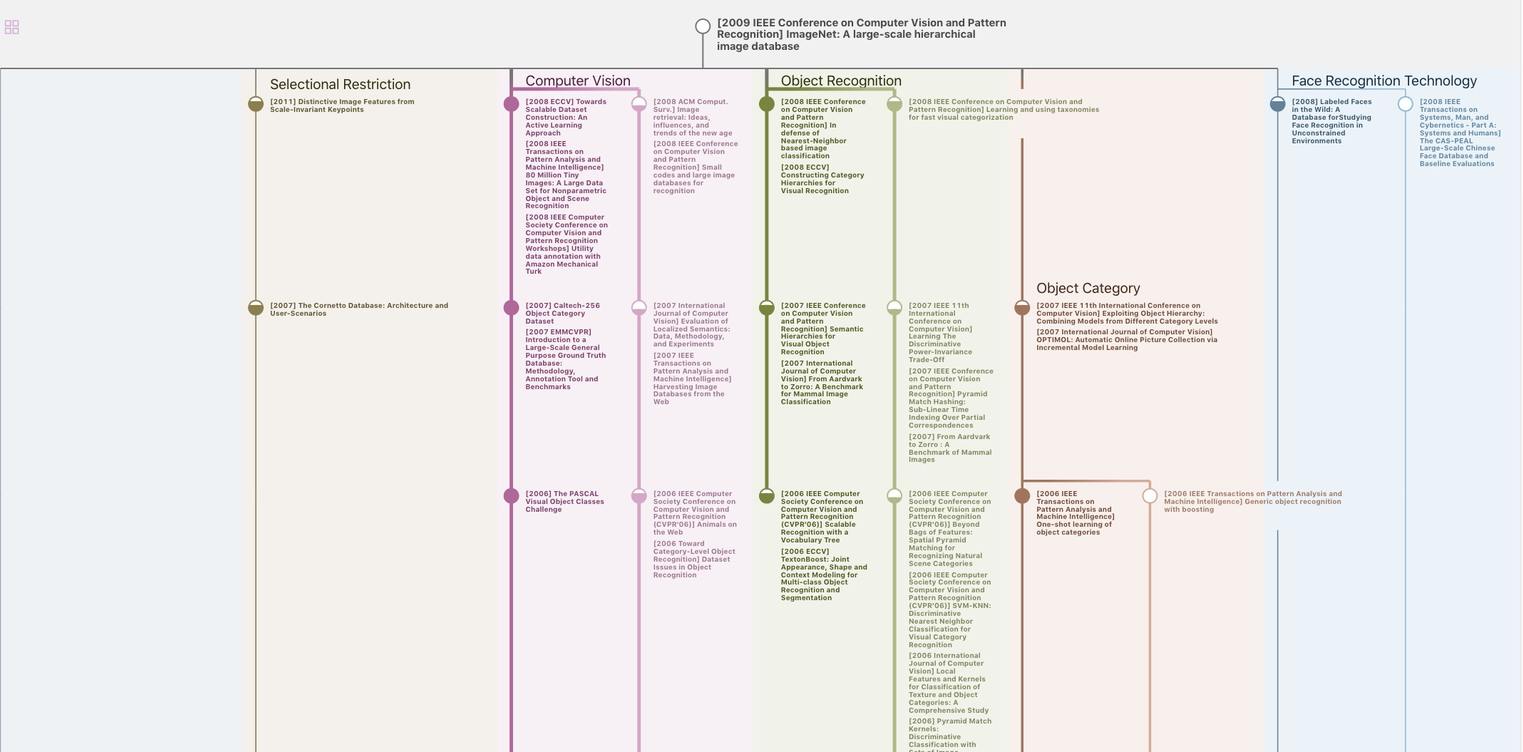
生成溯源树,研究论文发展脉络
Chat Paper
正在生成论文摘要