Motion-compensated frame interpolation with weighted motion estimation and hierarchical vector refinement.
Neurocomputing(2016)
摘要
Large amounts of data have been gathered by techniques such as mobile devices, remote sensing and cameras. With the rapid development of high-definition digital TV and multimedia information systems, motion-compensated frame interpolation (MCFI) has become a widely used tactic for frame rate up-conversion (FRUC) to improve the visual property of video. In this paper, we propose a novel MCFI algorithm based on weighted motion estimation (Weighted ME), motion vector (MV) segmentation, and hierarchical vector refinement. We first present an MV clustering based on the Weighted ME to obtain more accurate MVs and partition a frame into moving areas and background. To capture scene changes, we then apply a hierarchical vector refinement scheme to the moving areas that consists of three steps: pre-screening, reclassification, and smoothing. In this scheme, we use an overlapped block ME (OBME) method that uses multi-candidate pre-screening to discover unreliable MVs and protect the moving area edge structures from being damaged. We then employ the bidirectional prediction difference (BPD) to identify outliers using strong correlation with adjacent blocks. Meanwhile, an adaptive vector median filter (AVMF) is adopted to refine the block size, which can effectively smooth blocking artifacts and ghost effects. Experiments show the algorithm achieves a high image quality both subjectively and objectively. In particular, it has a good adaptability in video sequences with fast motion and complex backgrounds.
更多查看译文
关键词
Weighted motion estimation,Motion vector clustering,Hierarchical motion vector refinement,Motion-compensated frame interpolation
AI 理解论文
溯源树
样例
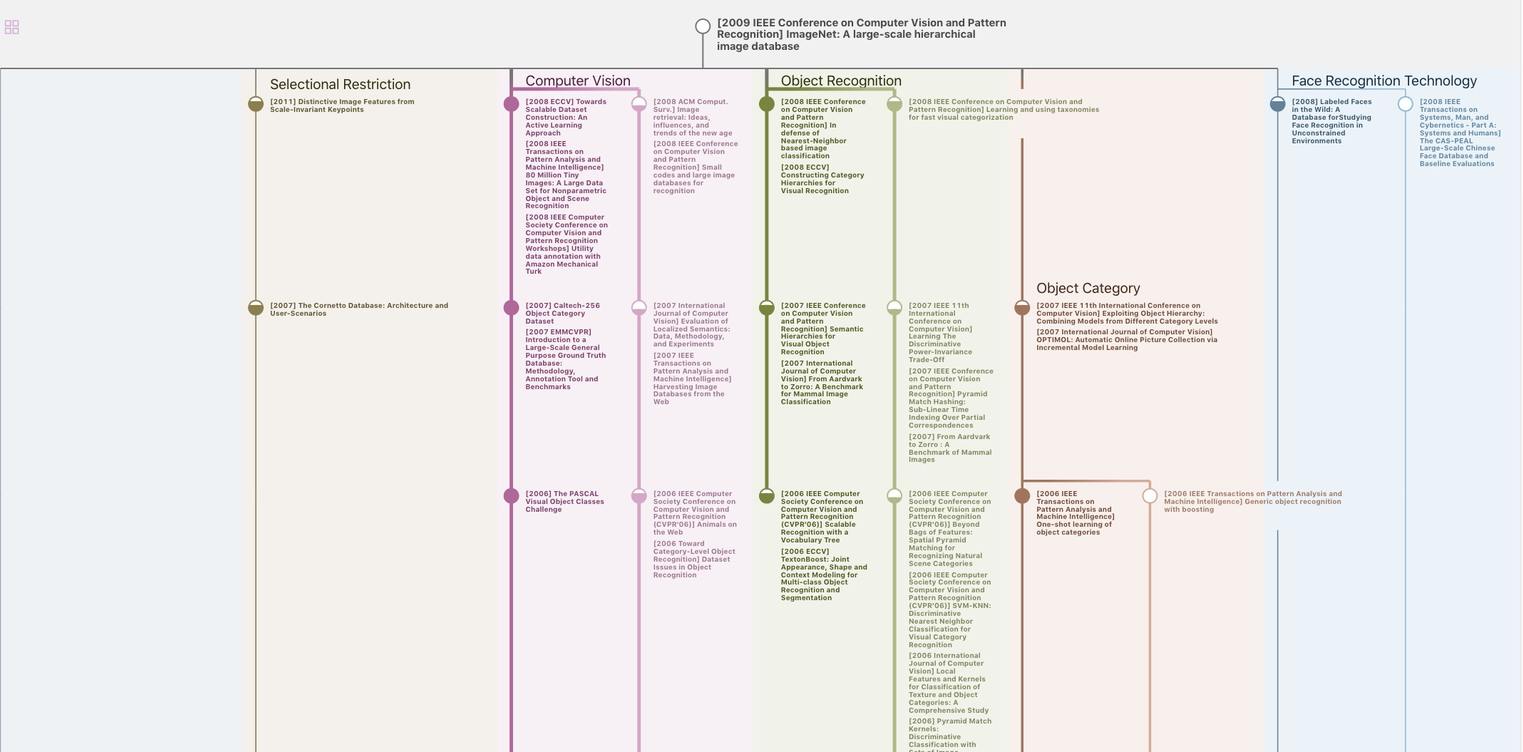
生成溯源树,研究论文发展脉络
Chat Paper
正在生成论文摘要