An improved upper bound on the expected regret of UCB-type policies for a matching-selection bandit problem
Operations Research Letters(2015)
摘要
We improved an upper bound on the expected regret of a UCB-type policy LLR for a bandit problem that repeats the following rounds: a player selects a maximal matching on a complete bipartite graph K M , N and receives a reward for each component edge of the selected matching. Rewards are assumed to be generated independently of its previous rewards according to an unknown fixed distribution. Our upper bound is smaller than the best known result (Chen et¿al., 2013) by a factor of ¿ ( M 2 / 3 ) .
更多查看译文
关键词
Multi-armed bandit problem,Matching,Regret analysis,Combinatorial bandit,Online learning
AI 理解论文
溯源树
样例
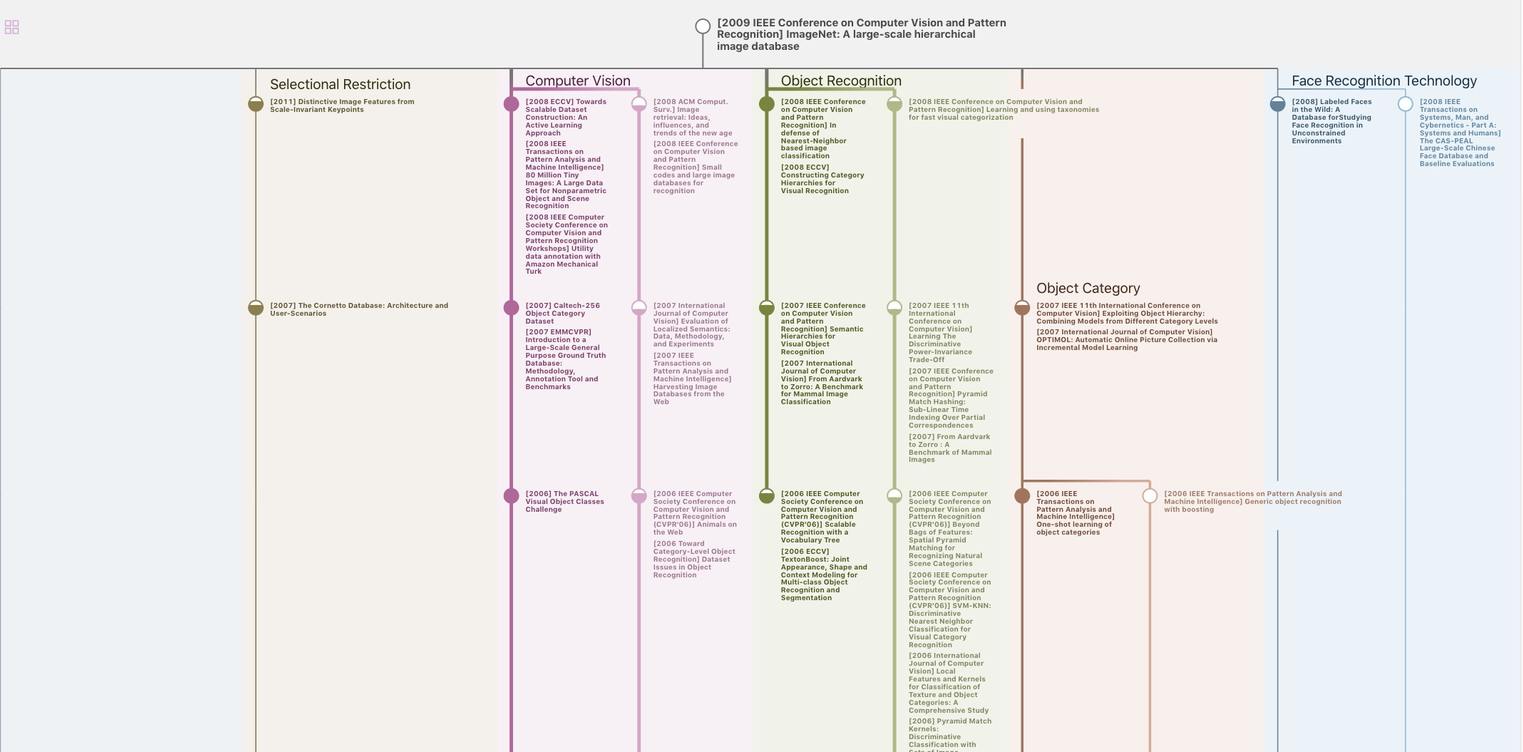
生成溯源树,研究论文发展脉络
Chat Paper
正在生成论文摘要