Hierarchical clustering based on the information bottleneck method using a control process
Pattern Analysis and Applications(2015)
摘要
Clustering techniques aim organizing data into groups whose members are similar. A key element of these techniques is the definition of a similarity measure. The information bottleneck method provides us a full solution of the clustering problem with no need to define a similarity measure, since a variable X is clustered depending on a control variable Y by maximizing the mutual information between them. In this paper, we propose a hierarchical clustering algorithm based on the information bottleneck method such that, instead of using a control variable, the different possible values of a Markov process are clustered by maximally preserving the mutual information between two consecutive states of the Markov process. These two states can be seen as the input and the output of an information channel that is used as a control process, similarly to how the variable Y is used as a control variable in the original information bottleneck algorithm. We present both agglomerative and divisive versions of our hierarchical clustering approach and two different applications. The first one, to quantize an image by grouping intensity bins of the image histograms, is tested on synthetic, photographic and medical images and compared with hand-labelled images, hierarchical clustering using Euclidean distance and non-negative matrix factorization methods. The second one, to cluster brain regions by grouping them depending on their connectivity, is tested on medical data. In all the applications, the obtained results demonstrate the efficacy of the method in getting clusters with high mutual information.
更多查看译文
关键词
Information bottleneck,Mutual information,Clustering,Image segmentation
AI 理解论文
溯源树
样例
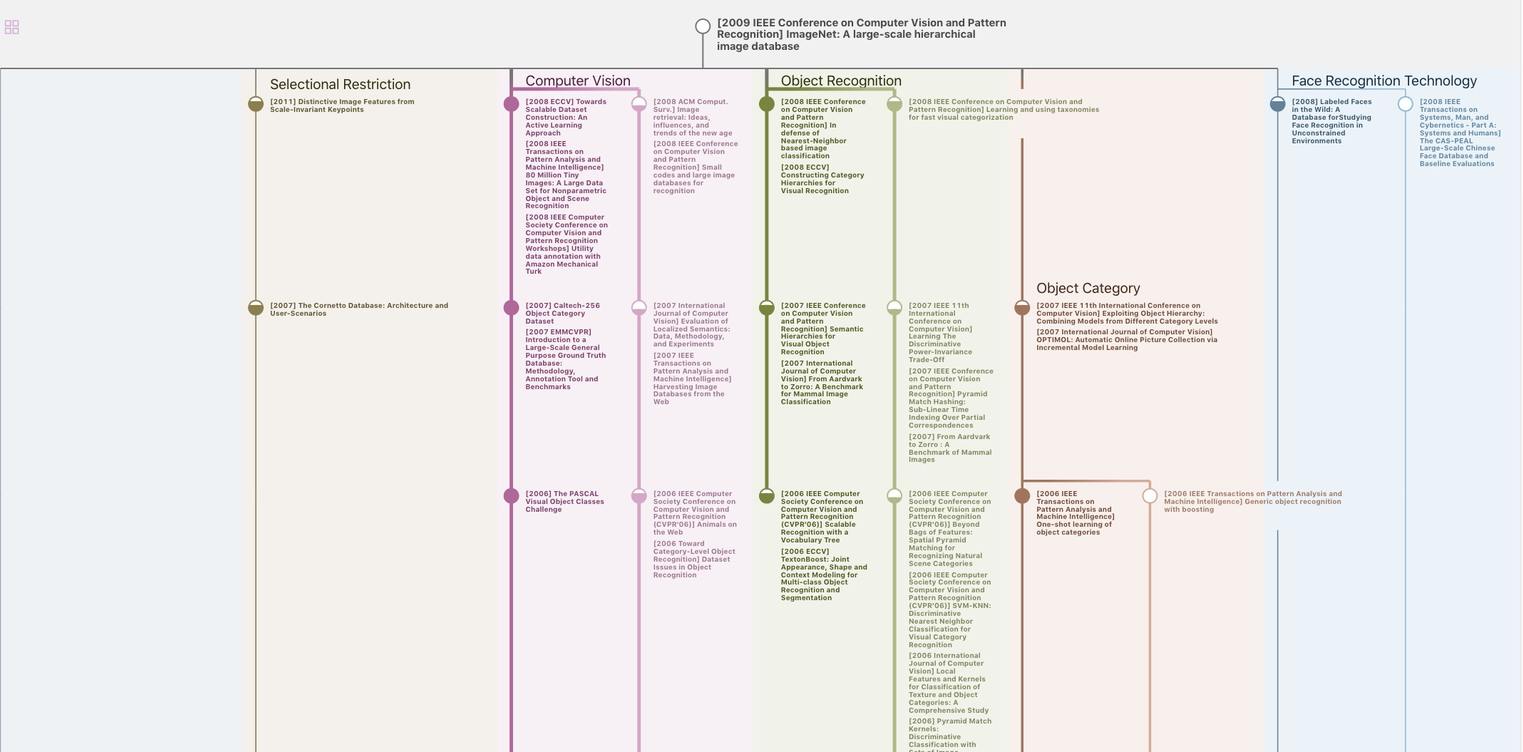
生成溯源树,研究论文发展脉络
Chat Paper
正在生成论文摘要