Near-Bayesian Support Vector Machines for imbalanced data classification with equal or unequal misclassification costs
Neural Networks(2015)
摘要
Support Vector Machines (SVMs) form a family of popular classifier algorithms originally developed to solve two-class classification problems. However, SVMs are likely to perform poorly in situations with data imbalance between the classes, particularly when the target class is under-represented. This paper proposes a Near-Bayesian Support Vector Machine (NBSVM) for such imbalanced classification problems, by combining the philosophies of decision boundary shift and unequal regularization costs. Based on certain assumptions which hold true for most real-world datasets, we use the fractions of representation from each of the classes, to achieve the boundary shift as well as the asymmetric regularization costs. The proposed approach is extended to the multi-class scenario and also adapted for cases with unequal misclassification costs for the different classes. Extensive comparison with standard SVM and some state-of-the-art methods is furnished as a proof of the ability of the proposed approach to perform competitively on imbalanced datasets. A modified Sequential Minimal Optimization (SMO) algorithm is also presented to solve the NBSVM optimization problem in a computationally efficient manner.
更多查看译文
关键词
Bayes error,Decision boundary shift,Imbalanced data,Multi-class classification,Support Vector Machines,Unequal costs
AI 理解论文
溯源树
样例
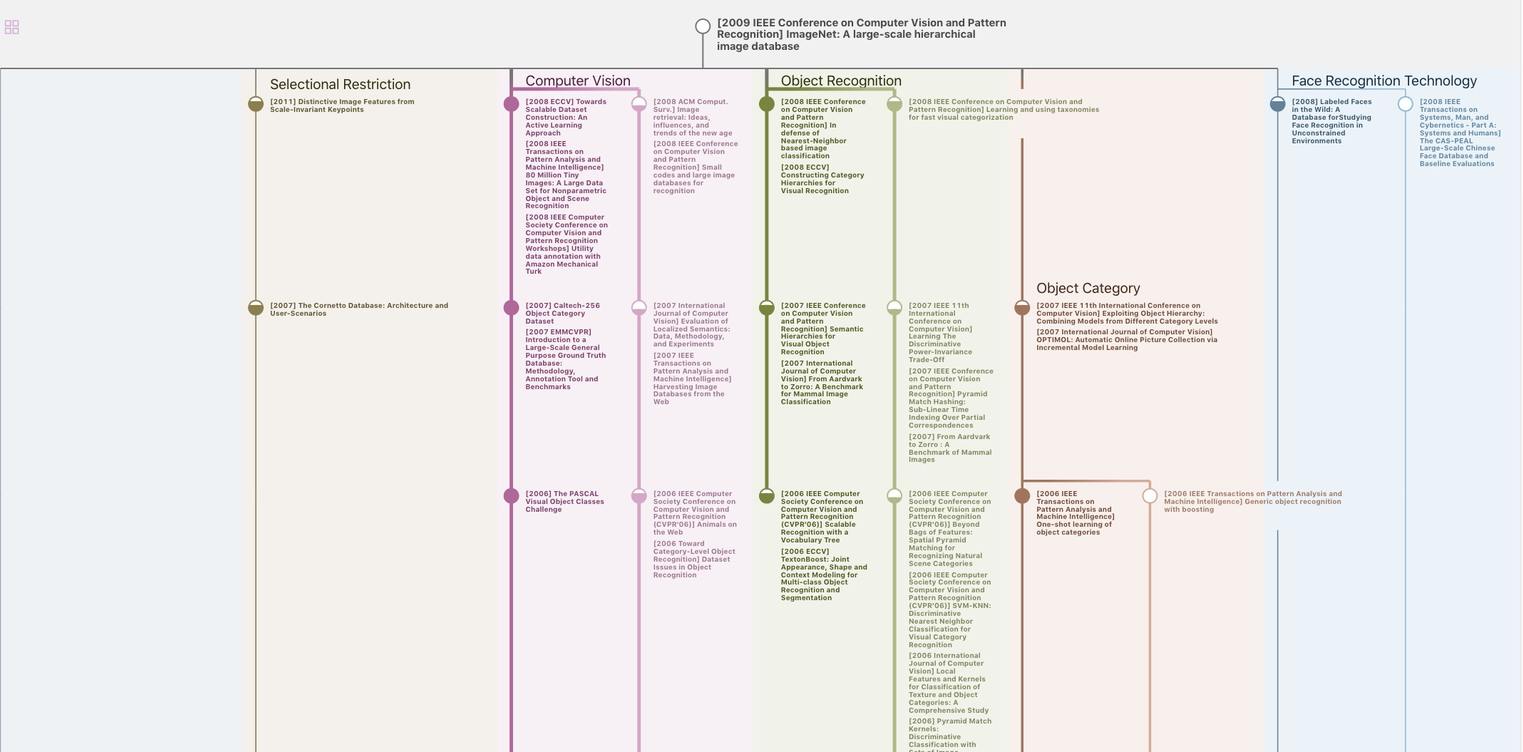
生成溯源树,研究论文发展脉络
Chat Paper
正在生成论文摘要