Class specific sparse representation for classification
Signal Processing(2015)
摘要
Motivated by the fact that the signals tend to have a representation biased towards their own classes, we propose a novel Sparse Representation-based Classifier (SRC) named Class Specific Sparse Representation-based Classifier (CSSRC), which incorporates the class information in the representation learning. Unlike the conventional SRC algorithms, CSSRC defines each class as a group and then impels these groups to compete for representing the test sample. To achieve such property, CSSRC imposes a L1-norm constraint to the classes for compulsively selecting the most relevant classes and introduces a L2-norm constraint to the samples belonging to the same class for making sure that all homogeneous samples can be sufficiently exploited for representation. Since CSSRC is a typical structure sparse representation issue, it can be efficiently solved by the convex optimization. Seven popular visual and audio signal databases are employed for evaluation. The results demonstrate its effectiveness in comparison with the state-of-the-art classifiers.
更多查看译文
关键词
Signal classification,Image classification,Sparse learning,Group sparsity,Face recognition,Collaborative representation
AI 理解论文
溯源树
样例
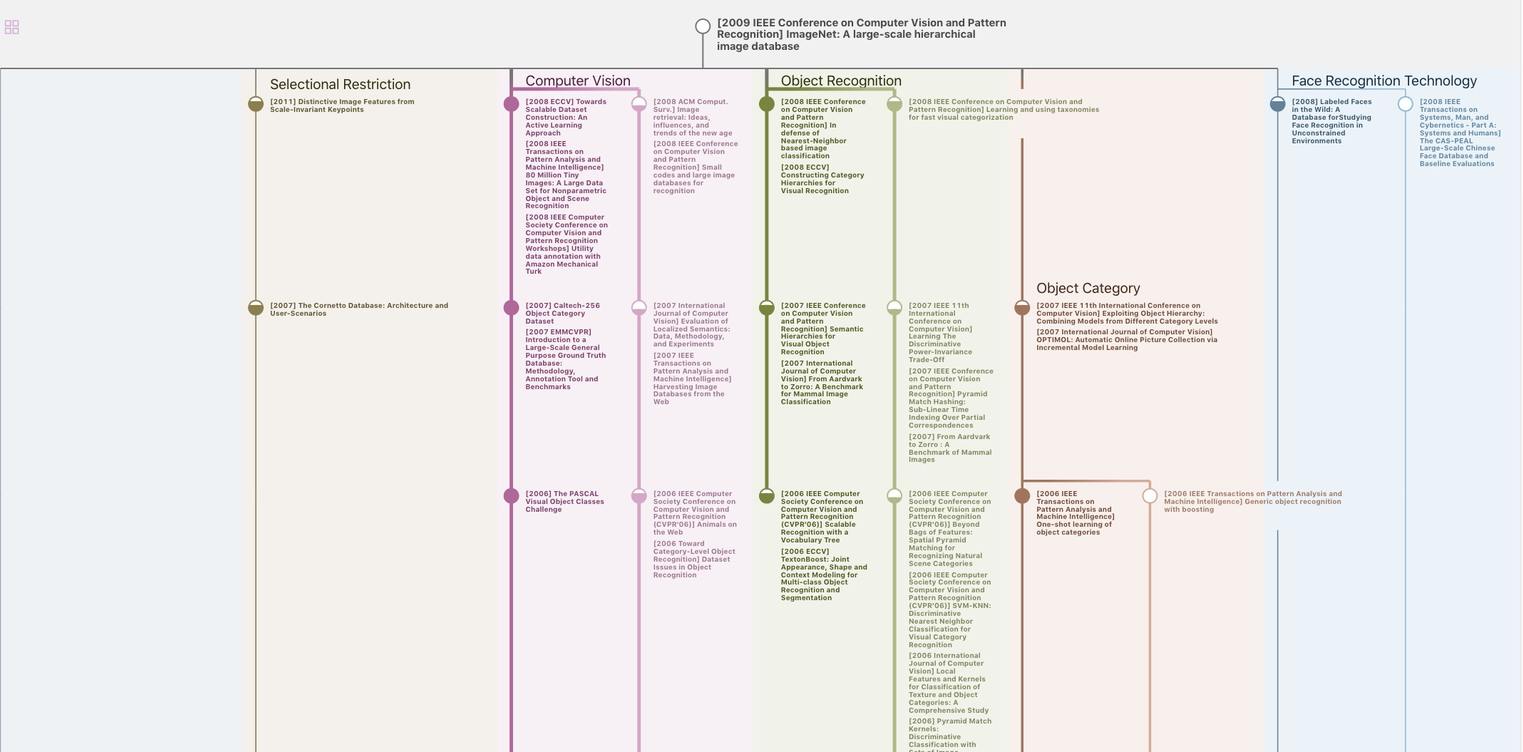
生成溯源树,研究论文发展脉络
Chat Paper
正在生成论文摘要