Estimating Clustering Coefficients and Size of Social Networks via Random Walk
TWEB(2015)
摘要
This work addresses the problem of estimating social network measures. Specifically, the measures at hand are the network average and global clustering coefficients and the number of registered users. The algorithms at hand (1) assume no prior knowledge about the network and (2) access the network using only the publicly available interface. More precisely, this work provides (a) a unified approach for clustering coefficients estimation and (b) a new network size estimator. The unified approach for the clustering coefficients yields the first external access algorithm for estimating the global clustering coefficient. The new network size estimator offers improved accuracy compared to prior art estimators. Our approach is to view a social network as an undirected graph and use the public interface to retrieve a random walk. To estimate the clustering coefficient, the connectivity of each node in the random walk sequence is tested in turn. We show that the error drops exponentially in the number of random walk steps. For the network size estimation we offer a generalized view of prior art estimators that in turn yields an improved estimator. All algorithms are validated on several publicly available social network datasets.
更多查看译文
关键词
Algorithms,Estimation,sampling,clustering coefficient,social network
AI 理解论文
溯源树
样例
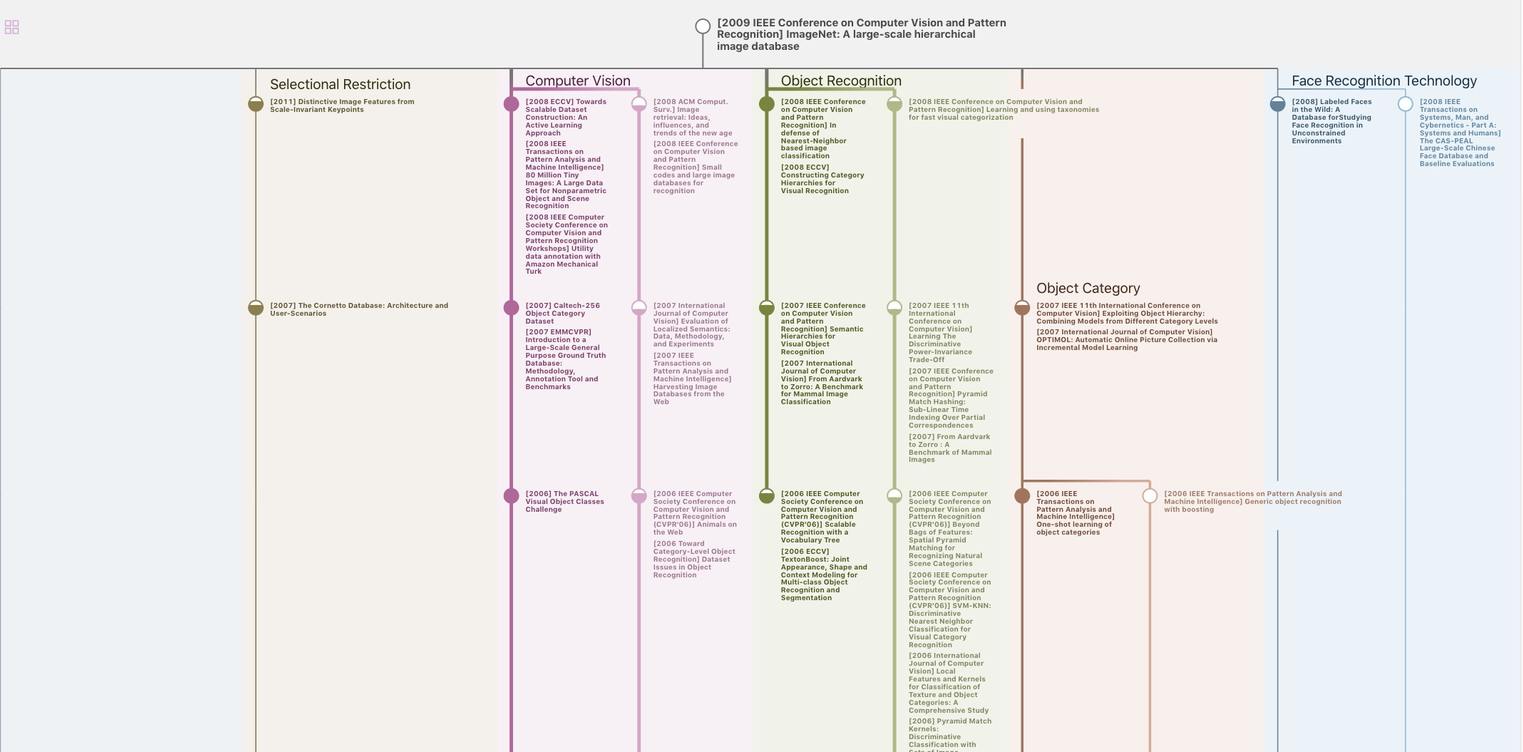
生成溯源树,研究论文发展脉络
Chat Paper
正在生成论文摘要