Multi-source adaptation learning with global and local regularization by exploiting joint kernel sparse representation.
Knowledge-Based Systems(2016)
摘要
While the advantages of using information from multi-source domains for establishing an adaptation model have been widely recognized, how to boosting the robustness of the learning model has only recently received attention. For achieving a robust adaptation learning model for visual classification tasks, by exploiting multi-source adaptation regularization joint kernel sparse representation (ARJKSR), we propose a novel Multi-source Adaptation learning method with Global and Local Regularization (MAGLR), which aims to minimize a cost function that properly trades off the global and local learning costs so as to utilize both the local and global discriminative information of target domain. Specifically, we first learn the optimal ARJKSR of domain datasets, which jointly represents the datasets by a sparse linear combination of a self-expressive dictionary in some optimal reproduced kernel Hilbert space (RKHS) recovered by minimizing the inter-domain distribution discrepancy, whilst constraining the observations from domains to share their sparse representations. Thus, we simultaneously take into account correlations as well as coupling information among multiple source domains. Based on the ARJKSR, we then derive a robust label prediction matrix for unlabeled instances from the target domain based on the classical graph-based semi-supervised learning diagram, into which global and local regularization are incorporated. Furthermore, a multi-kernel regression way is exploited to extend MAGLR to out-of-sample data. The validity of our method is examined by several real-world visual classification tasks. Experimental results demonstrate the superiority of our method.
更多查看译文
关键词
Multi-source adaptation learning,Joint sparse representation,Graph-based semi-supervised learning,Global and local regularization
AI 理解论文
溯源树
样例
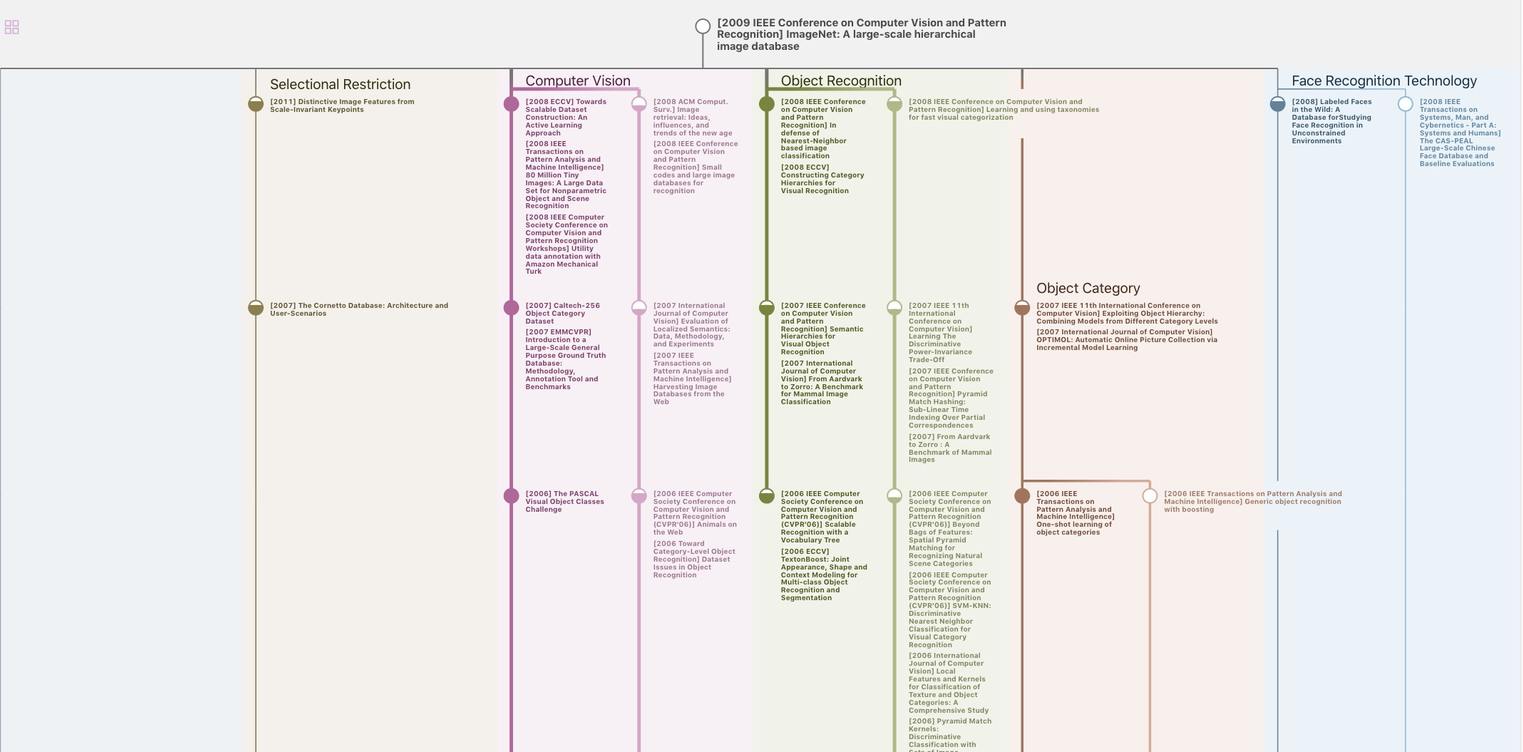
生成溯源树,研究论文发展脉络
Chat Paper
正在生成论文摘要