Automatic Erroneous Data Detection Over Type-Annotated Linked Data
IEICE TRANSACTIONS ON INFORMATION AND SYSTEMS(2016)
摘要
These days, the Web contains a huge volume of (semi-) structured data, called Linked Data (LD). However, LD suffer in data quality, and this poor data quality brings the need to identify erroneous data. Because manual erroneous data checking is impractical, automatic erroneous data detection is necessary. According to the data publishing guidelines of LD, data should use (already defined) ontology which populates type-annotated LD. Usually, the data type annotation helps in understanding the data. However, in our observation, the data type annotation could be used to identify erroneous data. Therefore, to automatically identify possible erroneous data over the type-annotated LD, we propose a framework that uses a novel nearest-neighbor based error detection technique. We conduct experiments of our framework on DBpedia, a type-annotated LD dataset, and found that our framework shows better performance of error detection in comparison with state-of-the-art framework.
更多查看译文
关键词
type-annotated LD, data quality, erroneous data detection
AI 理解论文
溯源树
样例
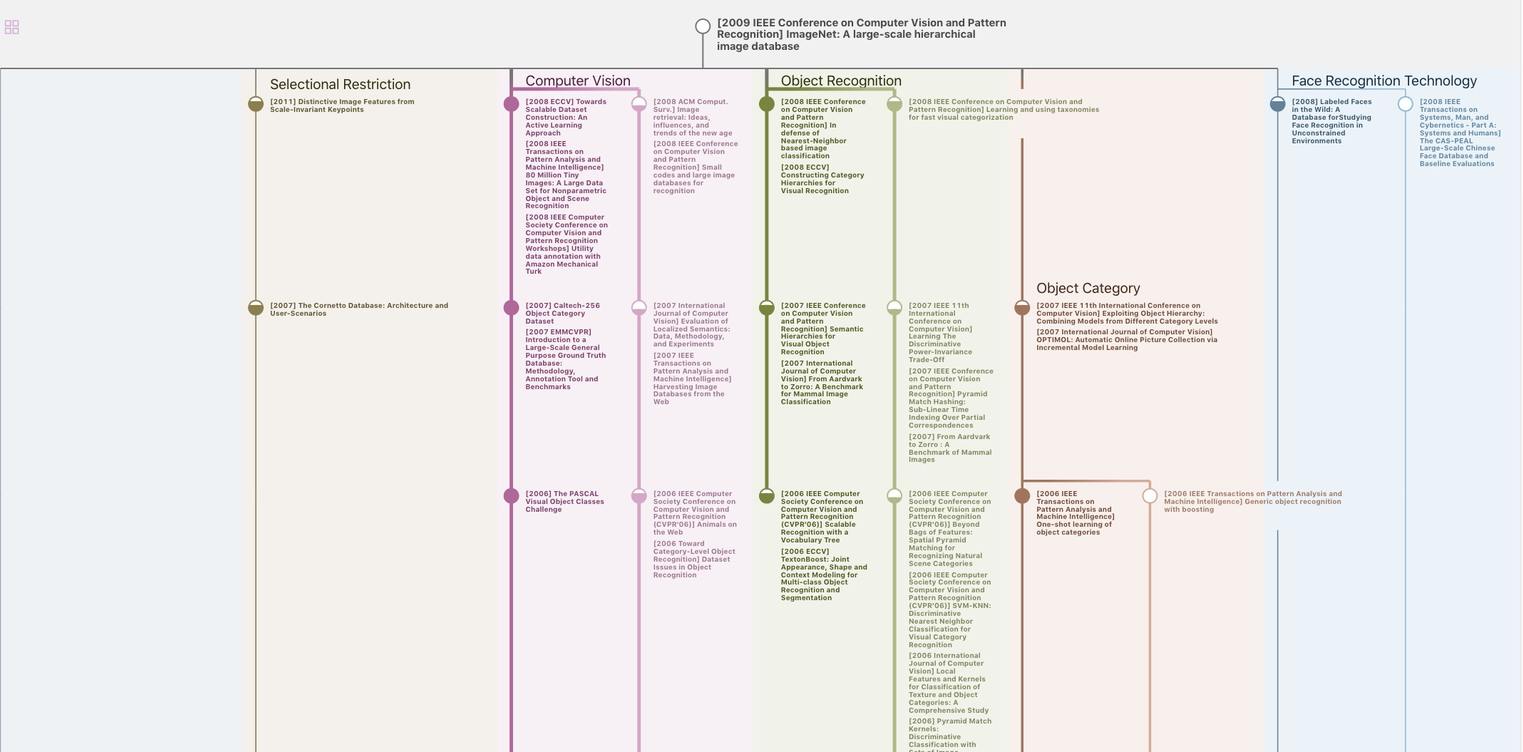
生成溯源树,研究论文发展脉络
Chat Paper
正在生成论文摘要