Improved Semi-Supervised Nmf Based Real-Time Capable Speech Enhancement
IEICE TRANSACTIONS ON FUNDAMENTALS OF ELECTRONICS COMMUNICATIONS AND COMPUTER SCIENCES(2016)
摘要
Nonnegative matrix factorization (NMF) is one of the most popular tools for speech enhancement. In this letter, we present an improved semi-supervised NMF (ISNMF)-based speech enhancement algorithm combining techniques of noise estimation and Incremental NMF (INMF). In this approach, fixed speech bases are obtained from training samples offline in advance while noise bases are trained on-the-fly whenever new noisy frame arrives. The INMF algorithm is adopted for noise bases learning because it can overcome the difficulties that conventional NMF confronts in online processing. The proposed algorithm is real-time capable in the sense that it processes the time frames of the noisy speech one by one and the computational complexity is feasible. Four different objective evaluation measures at various signal-to-noise ratio (SNR) levels demonstrate the superiority of the proposed method over traditional semi-supervised NMF (SNMF) and well-known robust principal component analysis (RPCA) algorithm.
更多查看译文
关键词
non-negative matrix factorization, incremental NMF, real-time capable, on-line training
AI 理解论文
溯源树
样例
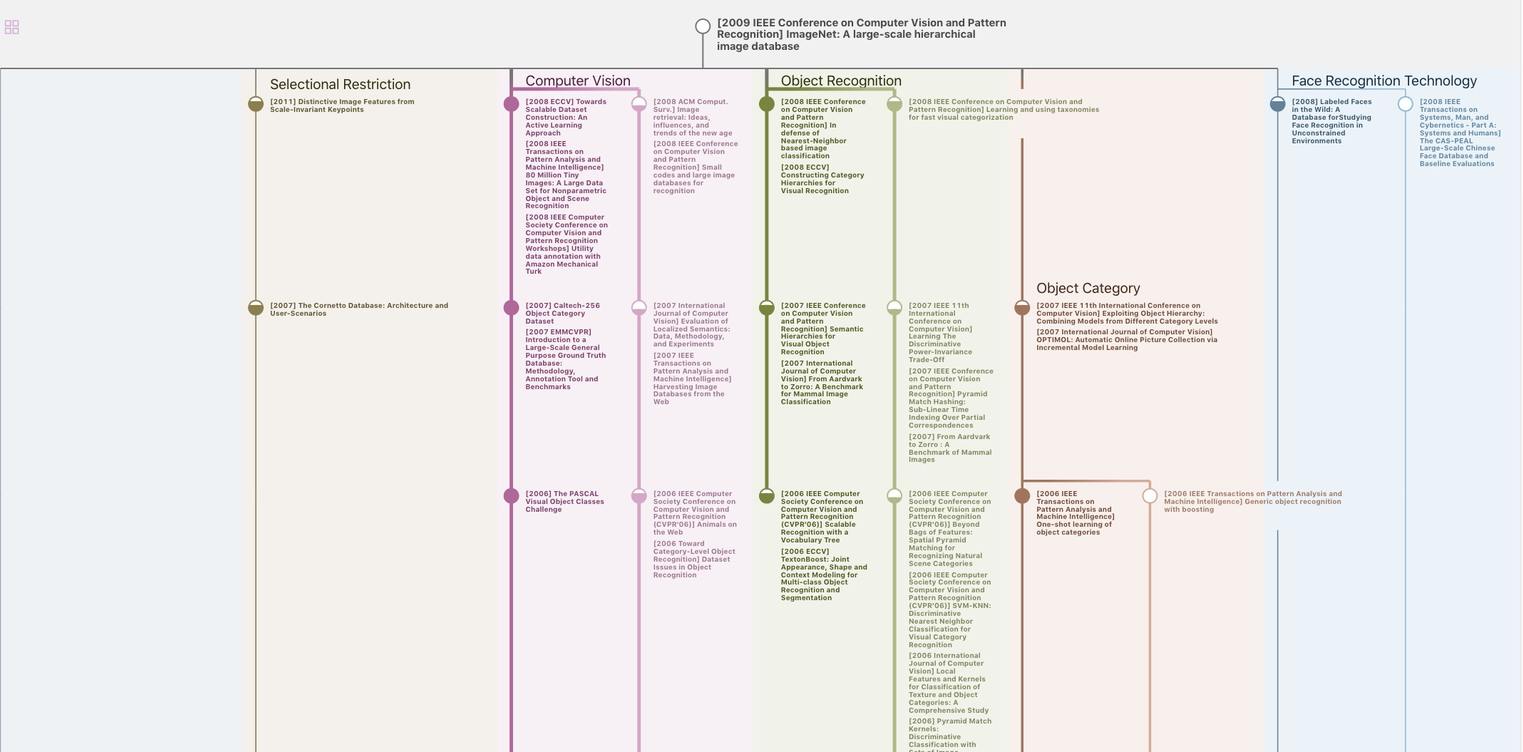
生成溯源树,研究论文发展脉络
Chat Paper
正在生成论文摘要