Nonnegative Component Representation With Hierarchical Dictionary Learning Strategy For Action Recognition
IEICE TRANSACTIONS ON INFORMATION AND SYSTEMS(2016)
摘要
Nonnegative component representation (NCR) is a midlevel representation based on nonnegative matrix factorization (NMF). Recently, it has attached much attention and achieved encouraging result for action recognition. In this paper, we propose a novel hierarchical dictionary learning strategy (HDLS) for NMF to improve the performance of NCR. Considering the variability of action classes, HDLS clusters the similar classes into groups and forms a two-layer hierarchical class model. The groups in the first layer are disjoint, while in the second layer, the classes in each group are correlated. HDLS takes account of the differences between two layers and proposes to use different dictionary learning methods for this two layers, including the discriminant class-specific NMF for the first layer and the discriminant joint dictionary NMF for the second layer. The proposed approach is extensively tested on three public datasets and the experimental results demonstrate the effectiveness and superiority of NCR with HDLS for large-scale action recognition.
更多查看译文
关键词
hierarchical dictionary learning strategy, nonnegative component representation, nonnegative matrix factorization, action recognition
AI 理解论文
溯源树
样例
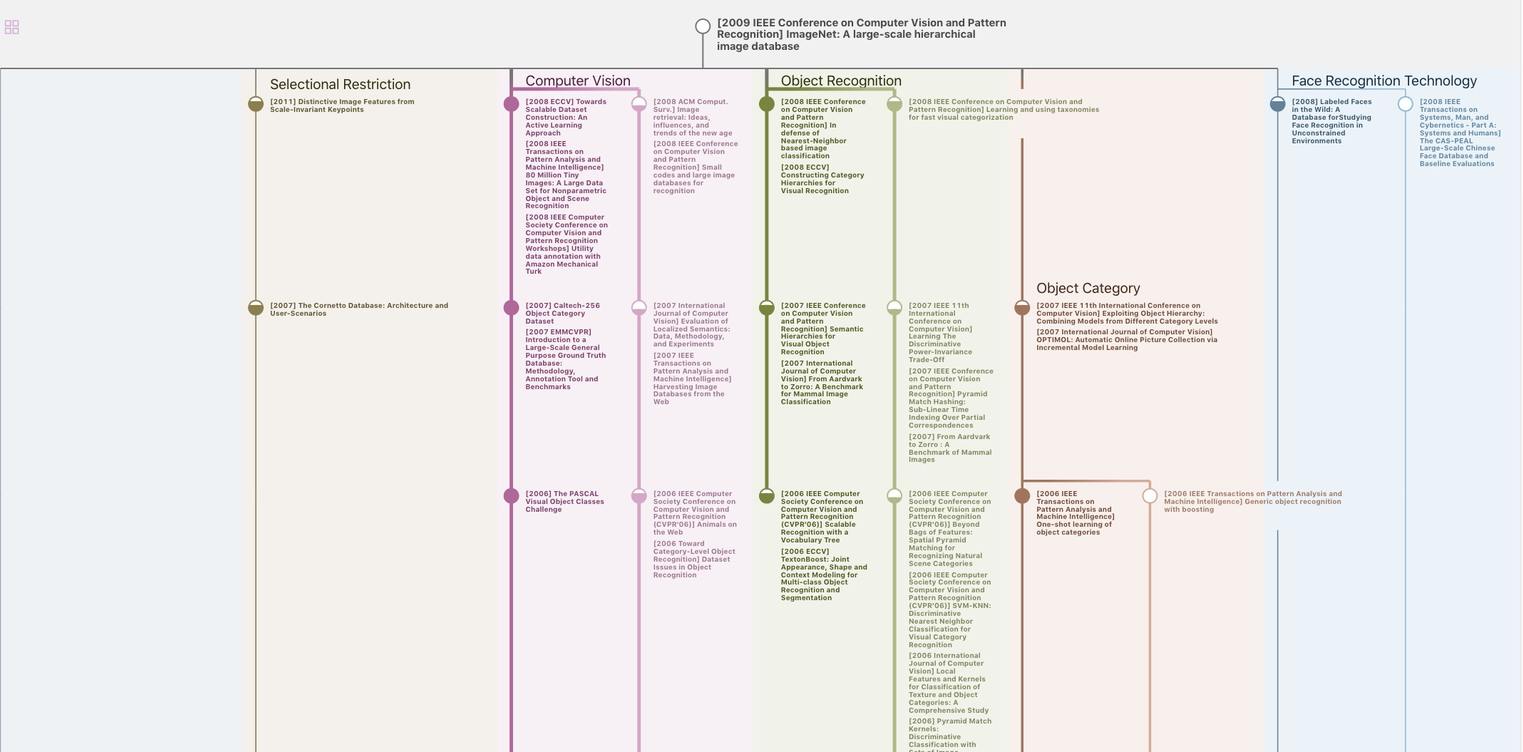
生成溯源树,研究论文发展脉络
Chat Paper
正在生成论文摘要