Newsvendor Problems With Demand Shocks And Unknown Demand Distributions
DECISION SCIENCES(2016)
摘要
In today's competitive market, demand volume and even the underlying demand distribution can change quickly for a newsvendor seller. We refer to sudden changes in demand distribution as demand shocks. When a newsvendor seller has limited demand distribution information and also experiences underlying demand shocks, the majority of existing methods for newsvendor problems may not work well since they either require demand distribution information or assume stationary demand distribution. We present a new, robust, and effective machine learning algorithm for newsvendor problems with demand shocks but without any demand distribution information. The algorithm needs only an approximate estimate of the lower and upper bounds of demand range; no other knowledge such as demand mean, variance, or distribution type is necessary. We establish the theoretical bounds that determine this machine learning algorithm's performance in handling demand shocks. Computational experiments show that this algorithm outperforms the traditional approaches in a variety of situations including large and frequent shocks of the demand mean. The method can also be used as a meta-algorithm by incorporating other traditional approaches as experts. Working together, the original algorithm and the extended meta-algorithm can help manufacturers and retailers better adapt their production and inventory control decisions in dynamic environments where demand information is limited and demand shocks are frequent
更多查看译文
关键词
Demand Forecasting, Demand Shocks, Inventory Management, Machine Learning Algorithm, Newsvendor Problem and Stochastic Demands
AI 理解论文
溯源树
样例
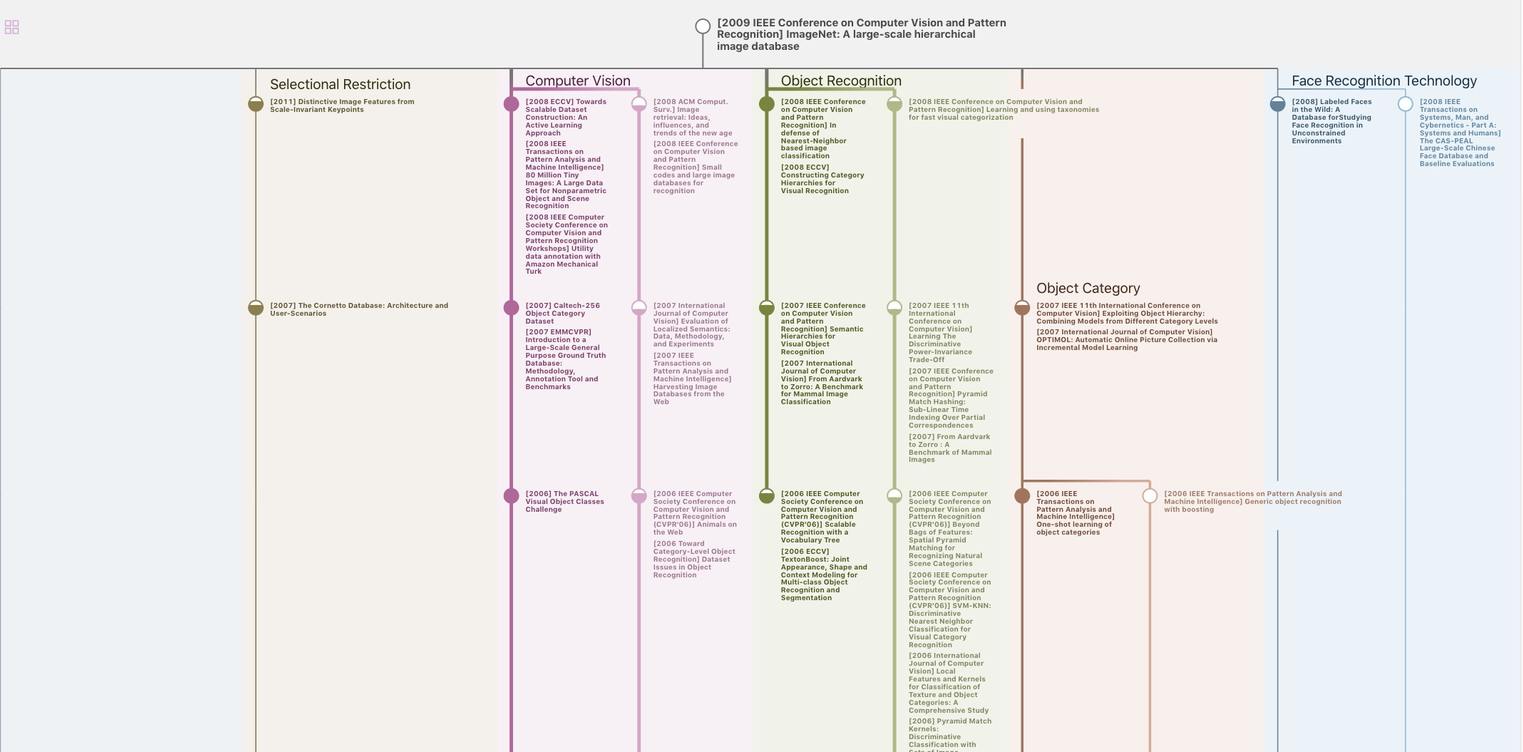
生成溯源树,研究论文发展脉络
Chat Paper
正在生成论文摘要