A Novel Fast Training Method for SVM and Its Application in Fault Diagnosis of Service Robot.
International journal of online and biomedical engineering(2015)
摘要
Support Vector Machines (SVM) is a set of popular machine learning algorithms which have been successfully applied in diverse aspects, but for large training data sets the processing time and computational costs are prohibitive. This paper presents a novel fast training method for SVM, which is applied in the fault diagnosis of service robot. Firstly, sensor data are sampled under different running conditions of the robot and those samples are divided as training sets and testing sets. Secondly, the sampled data are preprocessed and the principal component analysis (PCA) model is established for fault feature extraction. Thirdly, the feature vectors are used to train the SVM classifier, which achieves the fault diagnosis of the robot. To speed up the training process of SVM, on the one hand, sample reduction is done using the proposed support vectors selection (SVS) algorithm, which can ensure good classification accuracy and generalization capability. On the other hand, we take advantage of the excellent parallel computing abilities of Graphics Processing Unit (GPU) to pre-calculate the kernel matrix, which avoids the recalculation during the cross validation process. Experimental results illustrate that the proposed method can significantly reduce the training time without decreasing the classification accuracy.
更多查看译文
关键词
SVM,fast training method,support vectors selection,GPU,robot fault diagnosis
AI 理解论文
溯源树
样例
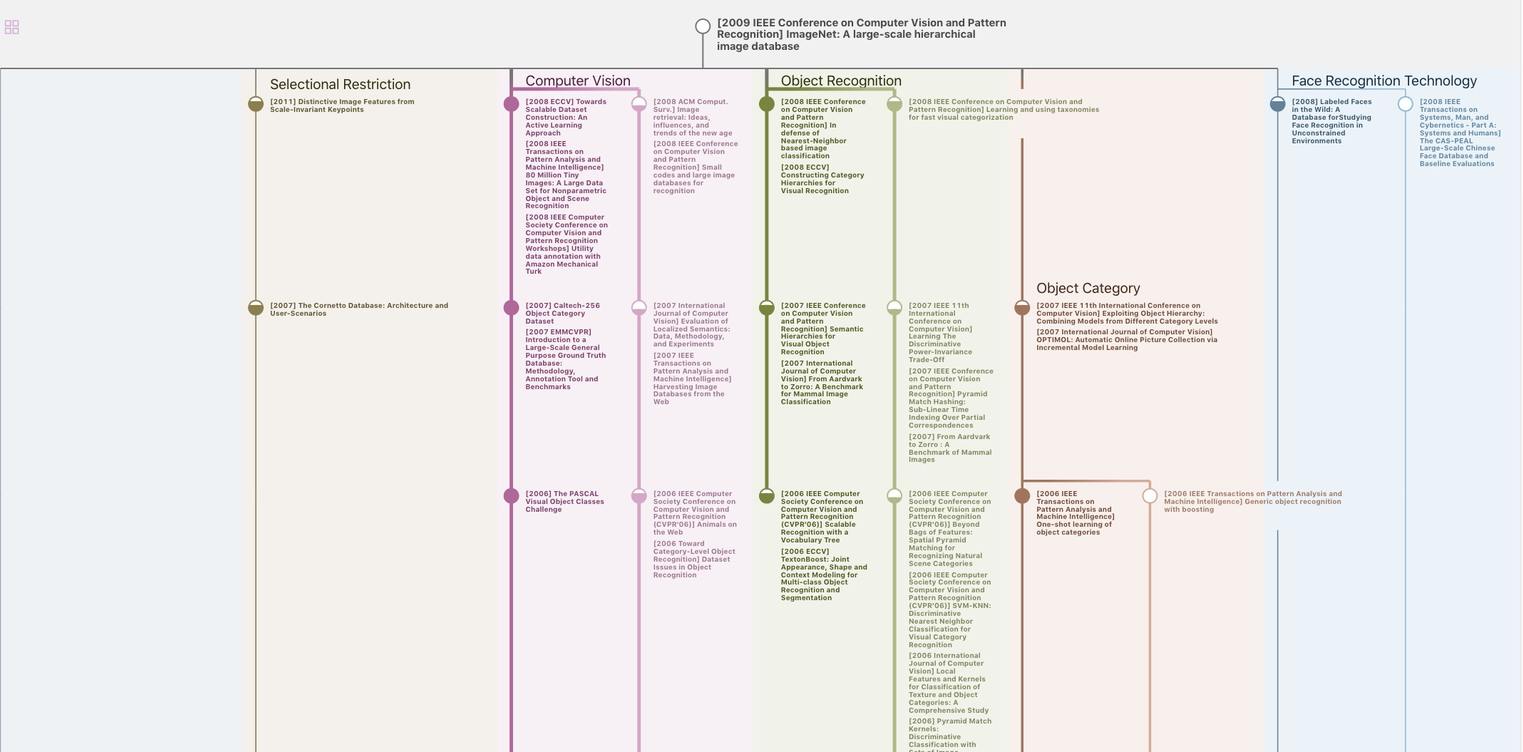
生成溯源树,研究论文发展脉络
Chat Paper
正在生成论文摘要