On training bi-directional neural network language model with noise contrastive estimation
2016 10th International Symposium on Chinese Spoken Language Processing (ISCSLP)(2016)
摘要
Although uni-directional recurrent neural network language model(RNNLM) has been very successful, it's hard to train a bi-directional RNNLM properly due to the generative nature of language model. In this work, we propose to train bi-directional RNNLM with noise contrastive estimation(NCE), since the properities of NCE training will help the model to acheieve sentence-level normalization. Experiments are conducted on two hand-crafted tasks on the PTB data set: a rescore task and a sanity test. Although(regretfully), the model trained by NCE did not out-perform the baseline uni-directional NNLM, it is shown that NCE-trained bi-directional NNLM behaves well in the sanity test and outperformed the one trained by conventional maximum likelihood training on the rescore task.
更多查看译文
关键词
Language model,recurrent neural network,noise contrastive estimation
AI 理解论文
溯源树
样例
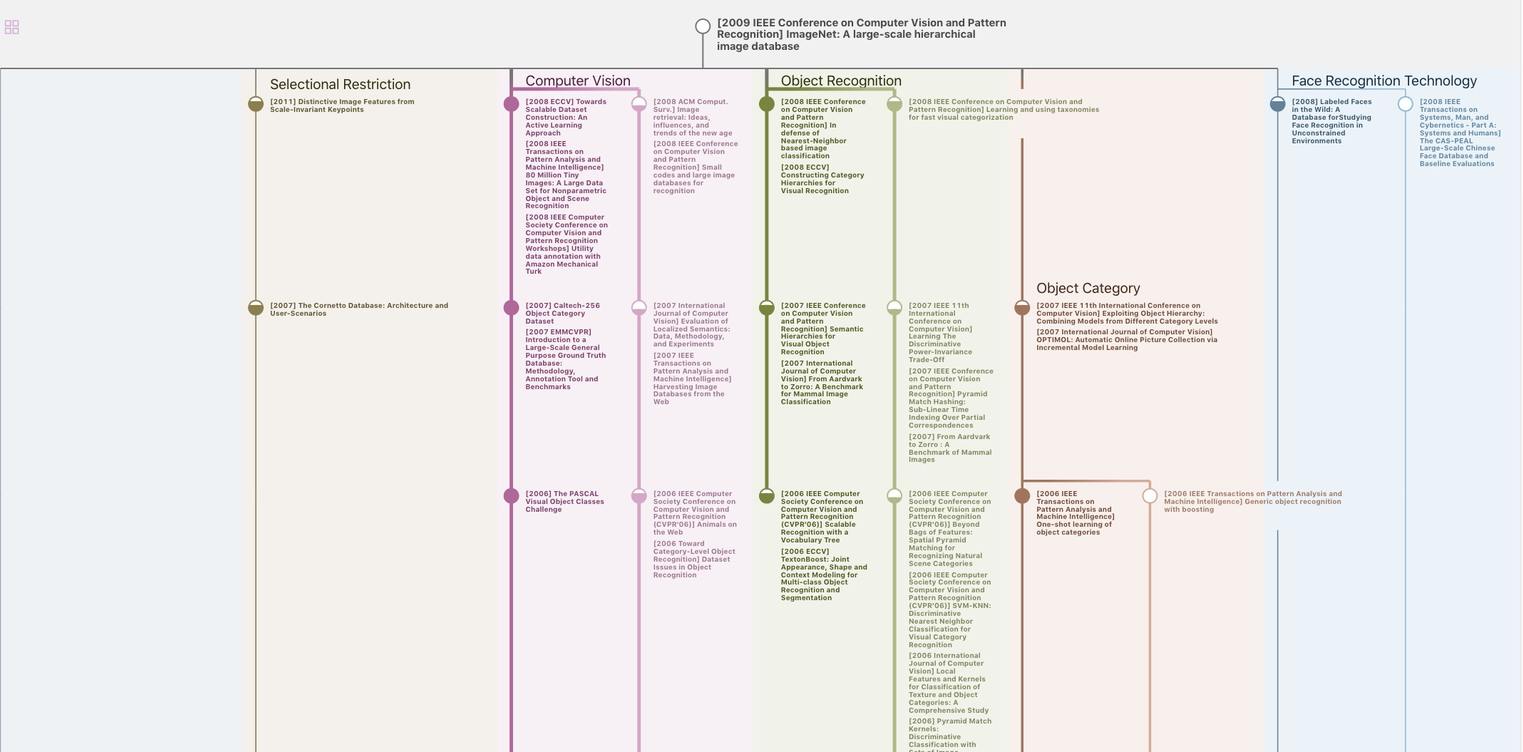
生成溯源树,研究论文发展脉络
Chat Paper
正在生成论文摘要