Get More With Less: Near Real-Time Image Clustering on Mobile Phones
CoRR(2015)
摘要
Machine learning algorithms, in conjunction with user data, hold the promise of revolutionizing the way we interact with our phones, and indeed their widespread adoption in the design of apps bear testimony to this promise. However, currently, the computationally expensive segments of the learning pipeline, such as feature extraction and model training, are offloaded to the cloud, resulting in an over-reliance on the network and under-utilization of computing resources available on mobile platforms. In this paper, we show that by combining the computing power distributed over a number of phones, judicious optimization choices, and contextual information it is possible to execute the end-to-end pipeline entirely on the phones at the edge of the network, efficiently. We also show that by harnessing the power of this combination, it is possible to execute a computationally expensive pipeline at near real-time. To demonstrate our approach, we implement an end-to-end image-processing pipeline -- that includes feature extraction, vocabulary learning, vectorization, and image clustering -- on a set of mobile phones. Our results show a 75% improvement over the standard, full pipeline implementation running on the phones without modification -- reducing the time to one minute under certain conditions. We believe that this result is a promising indication that fully distributed, infrastructure-less computing is possible on networks of mobile phones; enabling a new class of mobile applications that are less reliant on the cloud.
更多查看译文
AI 理解论文
溯源树
样例
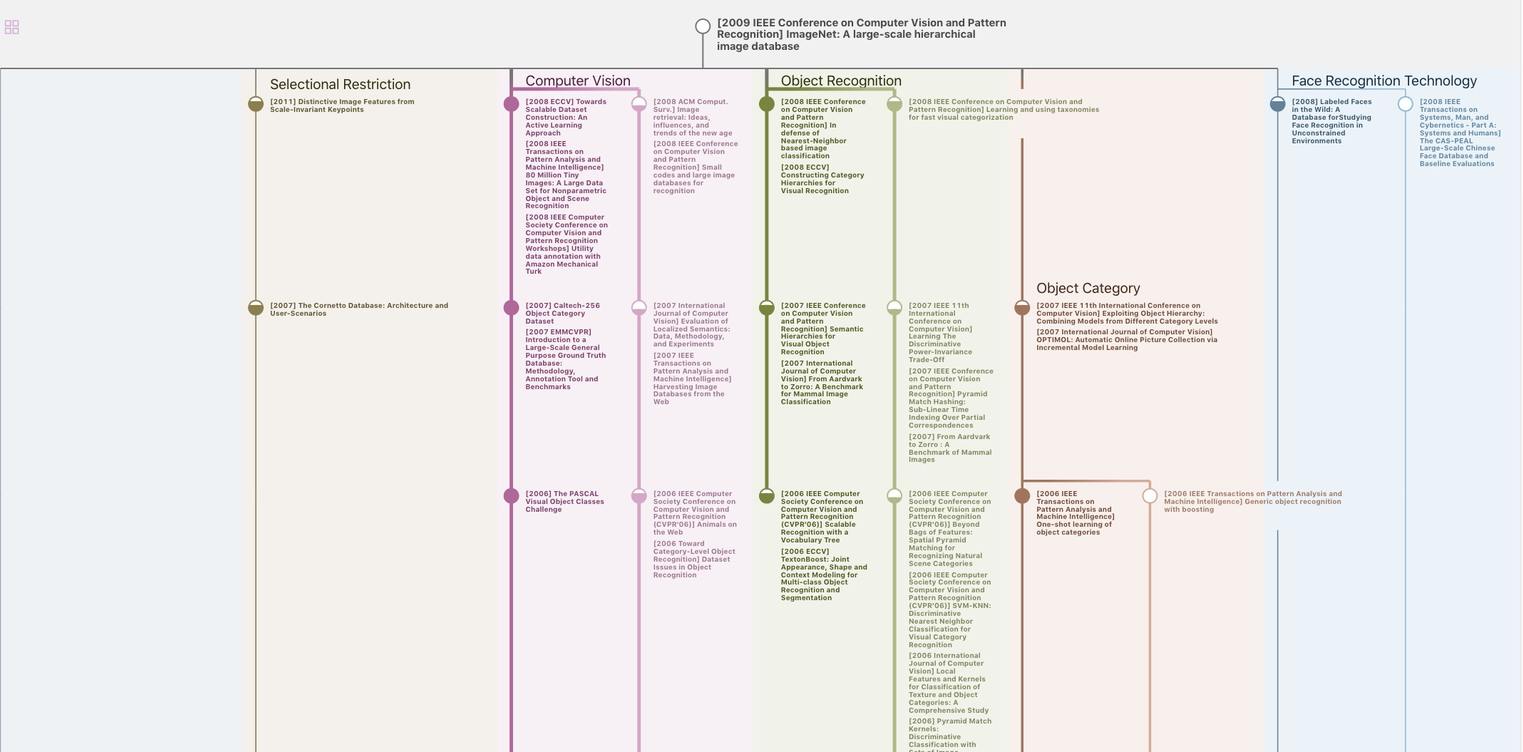
生成溯源树,研究论文发展脉络
Chat Paper
正在生成论文摘要