Scale-Free Online Learning
Theoretical Computer Science(2016)
摘要
We design algorithms for online linear optimization that have optimal regret and at the same time do not need to know any upper or lower bounds on the norm of the loss vectors. We achieve adaptiveness to the norms of the loss vectors by scale invariance, i.e., our algorithms make exactly the same decisions if the sequence of loss vectors is multiplied by any positive constant. One of our algorithms works for any decision set, bounded or unbounded. For unbounded decisions sets, this is the first adaptive algorithm for online linear optimization with a non-vacuous regret bound. We also study a popular scale-free variant of online mirror descent algorithm, and we show that in two natural settings it has linear or worse regret.
更多查看译文
关键词
Online algorithms,Optimization,Regret bounds,Online learning
AI 理解论文
溯源树
样例
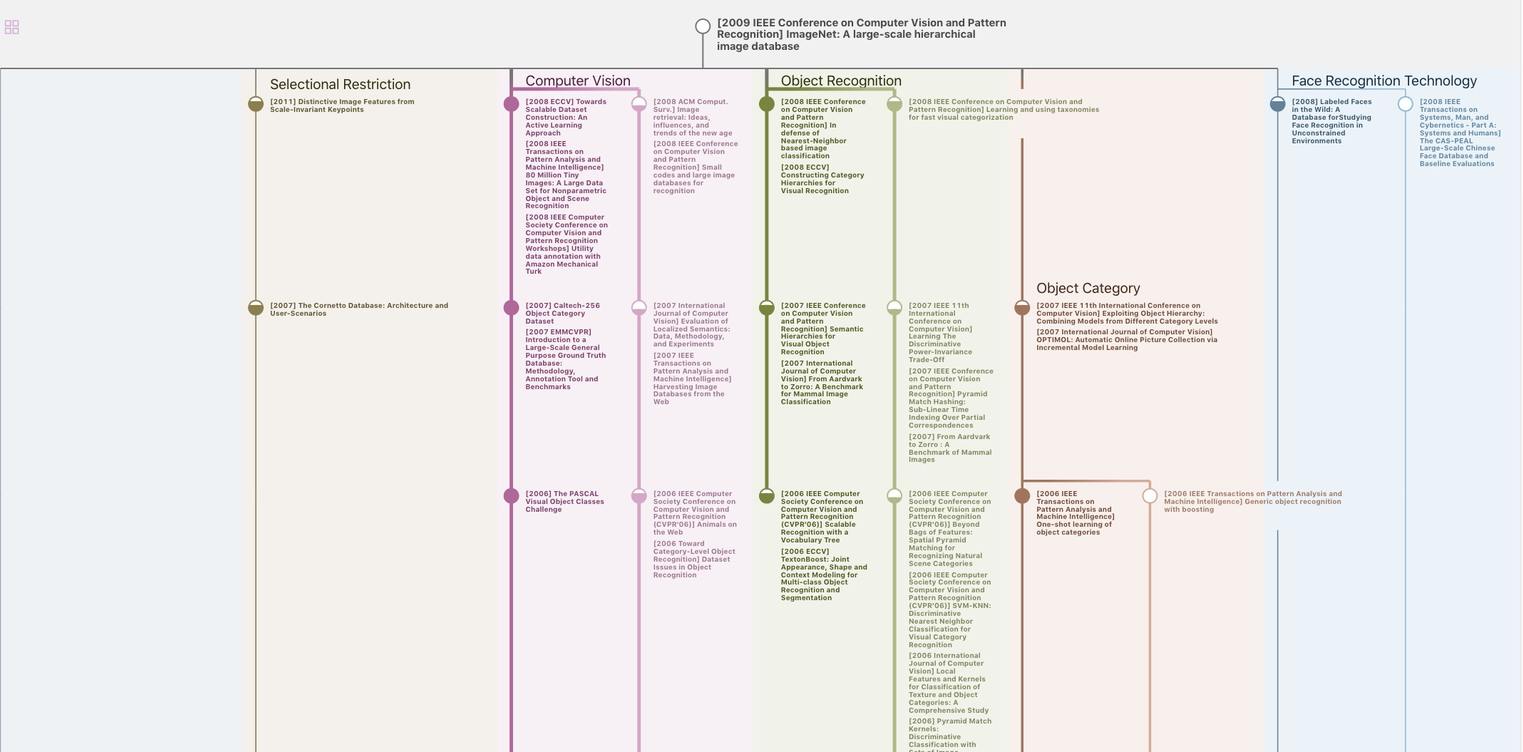
生成溯源树,研究论文发展脉络
Chat Paper
正在生成论文摘要