Multisection in the Stochastic Block Model using Semidefinite Programming
COMPRESSED SENSING AND ITS APPLICATIONS(2015)
摘要
We consider the problem of identifying underlying community-like structures in graphs. Towards this end we study the Stochastic Block Model (SBM) on $k$-clusters: a random model on $n=km$ vertices, partitioned in $k$ equal sized clusters, with edges sampled independently across clusters with probability $q$ and within clusters with probability $p$, $p>q$. The goal is to recover the initial "hidden" partition of $[n]$. We study semidefinite programming (SDP) based algorithms in this context. In the regime $p = \frac{\alpha \log(m)}{m}$ and $q = \frac{\beta \log(m)}{m}$ we show that a certain natural SDP based algorithm solves the problem of {\em exact recovery} in the $k$-community SBM, with high probability, whenever $\sqrt{\alpha} - \sqrt{\beta} > \sqrt{1}$, as long as $k=o(\log n)$. This threshold is known to be the information theoretically optimal. We also study the case when $k=\theta(\log(n))$. In this case however we achieve recovery guarantees that no longer match the optimal condition $\sqrt{\alpha} - \sqrt{\beta} > \sqrt{1}$, thus leaving achieving optimality for this range an open question.
更多查看译文
关键词
Graph partitioning,Random models,Stochastic block model,Semidefinite programming,Dual certificate
AI 理解论文
溯源树
样例
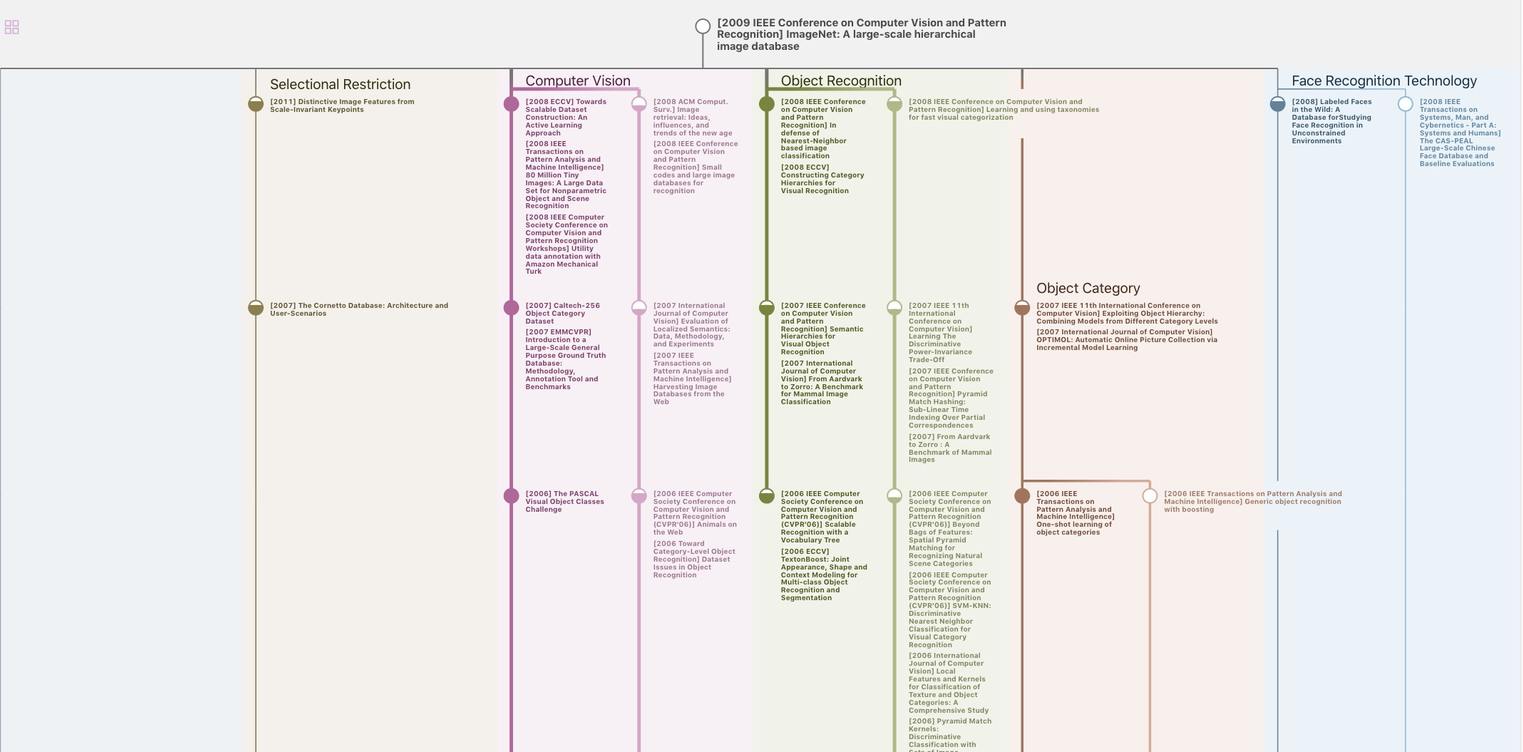
生成溯源树,研究论文发展脉络
Chat Paper
正在生成论文摘要