Auditing Black-box Models by Obscuring Features.
arXiv: Machine Learning(2016)
摘要
Data-trained predictive models are widely used to assist in decision making. But they are used as black boxes that output a prediction or score. It is therefore hard to acquire a deeper understanding of model behavior: and in particular how different attributes influence the model prediction. This is very important when trying to interpret the behavior of complex models, or ensure that certain problematic attributes (like race or gender) are not unduly influencing decisions. In this paper, we present a technique for auditing black-box models: we can study the extent to which existing models take advantage of particular features in the dataset without knowing how the models work. We show how a class of techniques originally developed for the detection and repair of disparate impact in classification models can be used to study the sensitivity of any model with respect to any feature subsets. Our approach does not require the black-box model to be retrained. This is important if (for example) the model is only accessible via an API, and contrasts our work with other methods that investigate feature influence like feature selection. We present experimental evidence for the effectiveness of our procedure using a variety of publicly available datasets and models. We also validate our procedure using techniques from interpretable learning and feature selection.
更多查看译文
AI 理解论文
溯源树
样例
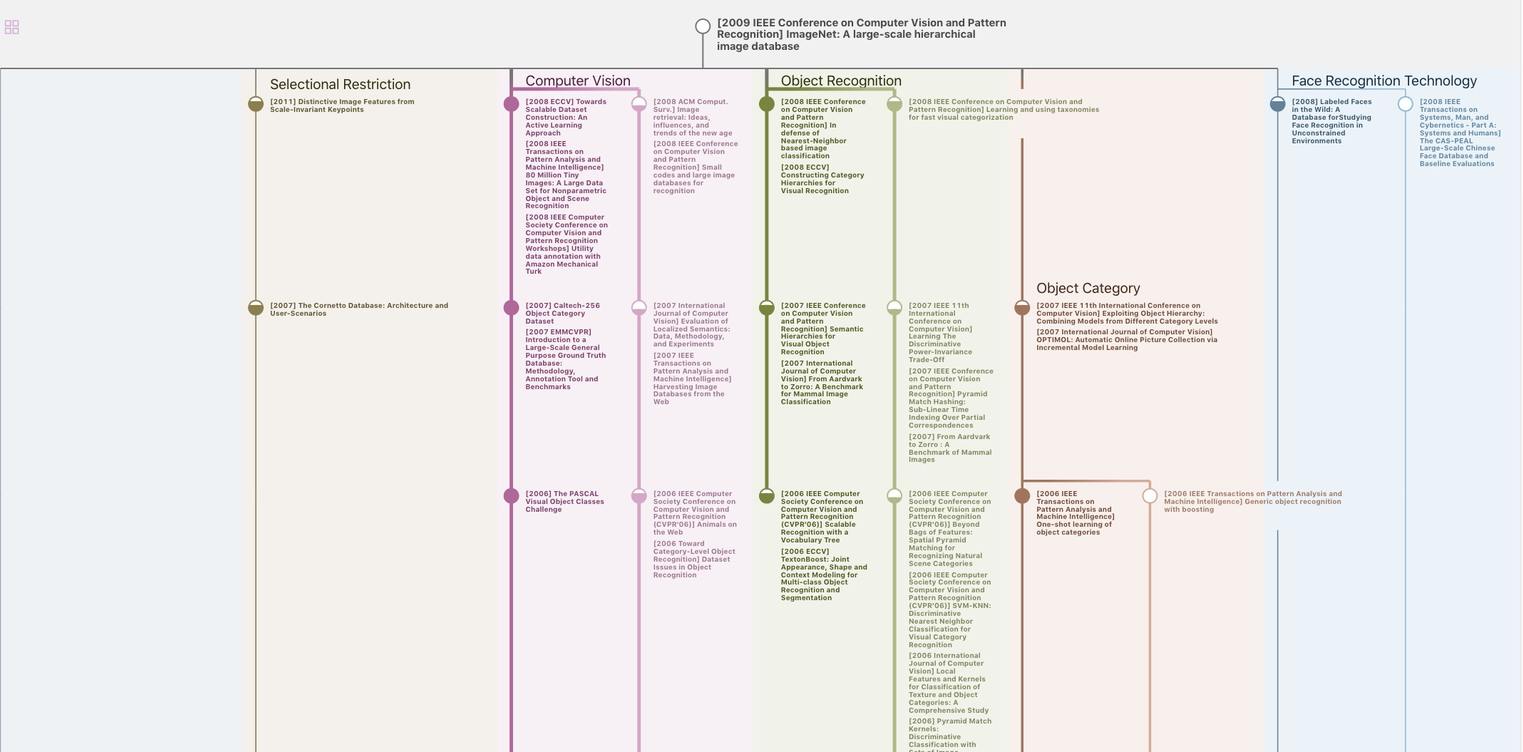
生成溯源树,研究论文发展脉络
Chat Paper
正在生成论文摘要