Deep Reflectance Maps
2016 IEEE Conference on Computer Vision and Pattern Recognition (CVPR)(2015)
摘要
Undoing the image formation process and therefore decomposing appearance into its intrinsic properties is a challenging task due to the under-constraint nature of this inverse problem. While significant progress has been made on inferring shape, materials and illumination from images only, progress in an unconstrained setting is still limited. We propose a convolutional neural architecture to estimate reflectance maps of specular materials in natural lighting conditions. We achieve this in an end-to-end learning formulation that directly predicts a reflectance map from the image itself. We show how to improve estimates by facilitating additional supervision in an indirect scheme that first predicts surface orientation and afterwards predicts the reflectance map by a learning-based sparse data interpolation. In order to analyze performance on this difficult task, we propose a new challenge of Specular MAterials on SHapes with complex IllumiNation (SMASHINg) using both synthetic and real images. Furthermore, we show the application of our method to a range of image-based editing tasks on real images.
更多查看译文
关键词
deep reflectance maps,image formation,appearance decomposition,convolutional neural architecture,reflectance map estimation,natural lighting conditions,end-to-end learning formulation,reflectance map prediction,surface orientation prediction,learning-based sparse data interpolation,specular materials on shapes with complex illumination SMASHINg,image editing
AI 理解论文
溯源树
样例
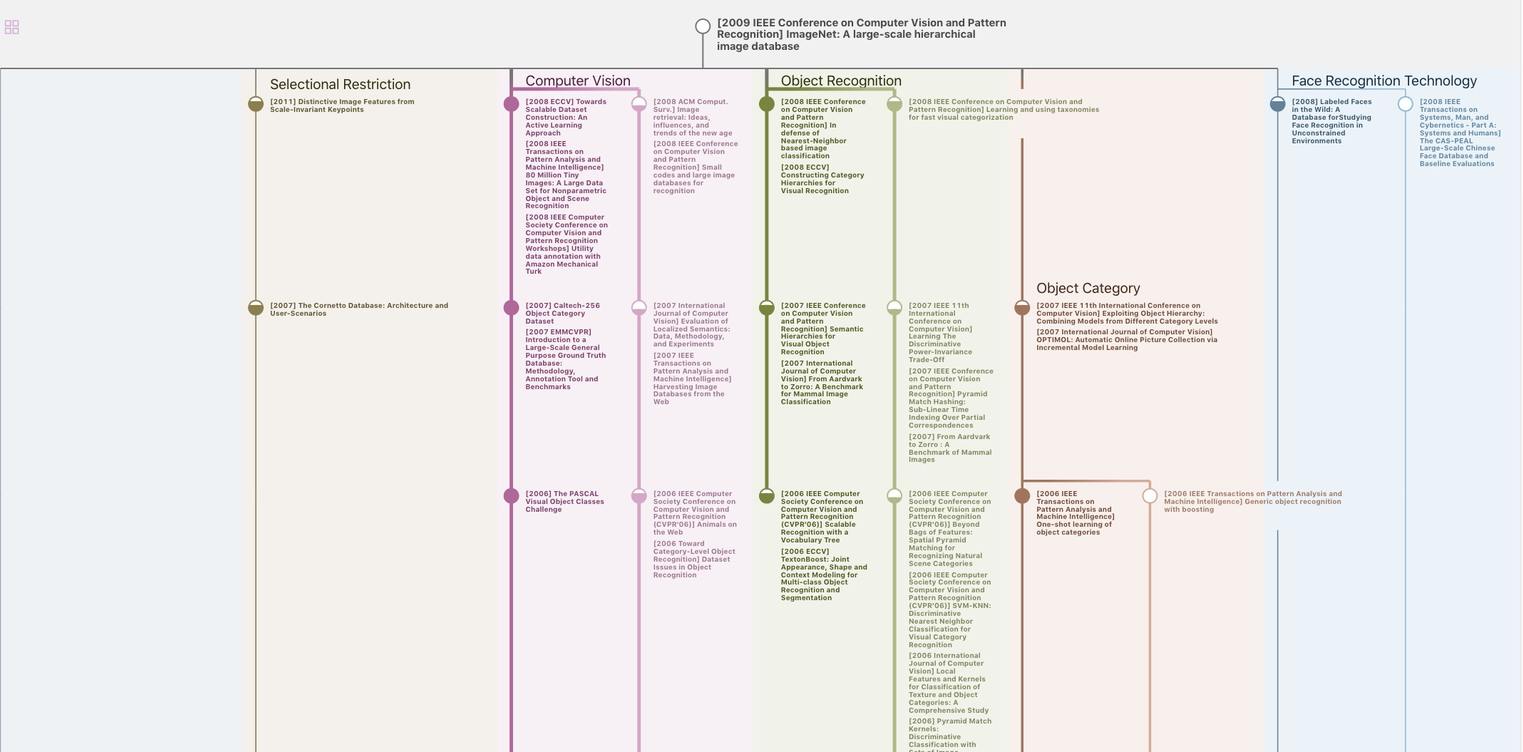
生成溯源树,研究论文发展脉络
Chat Paper
正在生成论文摘要