Towards the design of an end-to-end automated system for image and video-based recognition
2016 Information Theory and Applications Workshop (ITA)(2016)
摘要
Over many decades, researchers working in object recognition have longed for an end-to-end automated system that will simply accept 2D or 3D image or videos as inputs and output the labels of objects in the input data. Computer vision methods that use representations derived based on geometric, radiometric and neural considerations and statistical and structural matchers and artificial neural network-based methods where a multi-layer network learns the mapping from inputs to class labels have provided competing approaches for image recognition problems. Over the last four years, methods based on Deep Convolutional Neural Networks (DCNNs) have shown impressive performance improvements on object detection/recognition challenge problems. This has been made possible due to the availability of large annotated data, a better understanding of the non-linear mapping between image and class labels as well as the affordability of GPUs. In this paper, we present a brief history of developments in computer vision and artificial neural networks over the last forty years for the problem of image-based recognition. We then present the design details of a deep learning system for end-to-end unconstrained face verification/recognition. Some open issues regarding DCNNs for object recognition problems are then discussed. We caution the readers that the views expressed in this paper are from the authors and authors only!
更多查看译文
关键词
end-to-end automated system,image-based recognition,video-based recognition,object recognition,computer vision,image representations,geometric considerations,radiometric considerations,neural considerations,statistical matchers,structural matchers,artificial neural network,multilayer network,deep convolutional neural networks,DCNNs,object detection,deep learning system,end-to-end unconstrained face recognition
AI 理解论文
溯源树
样例
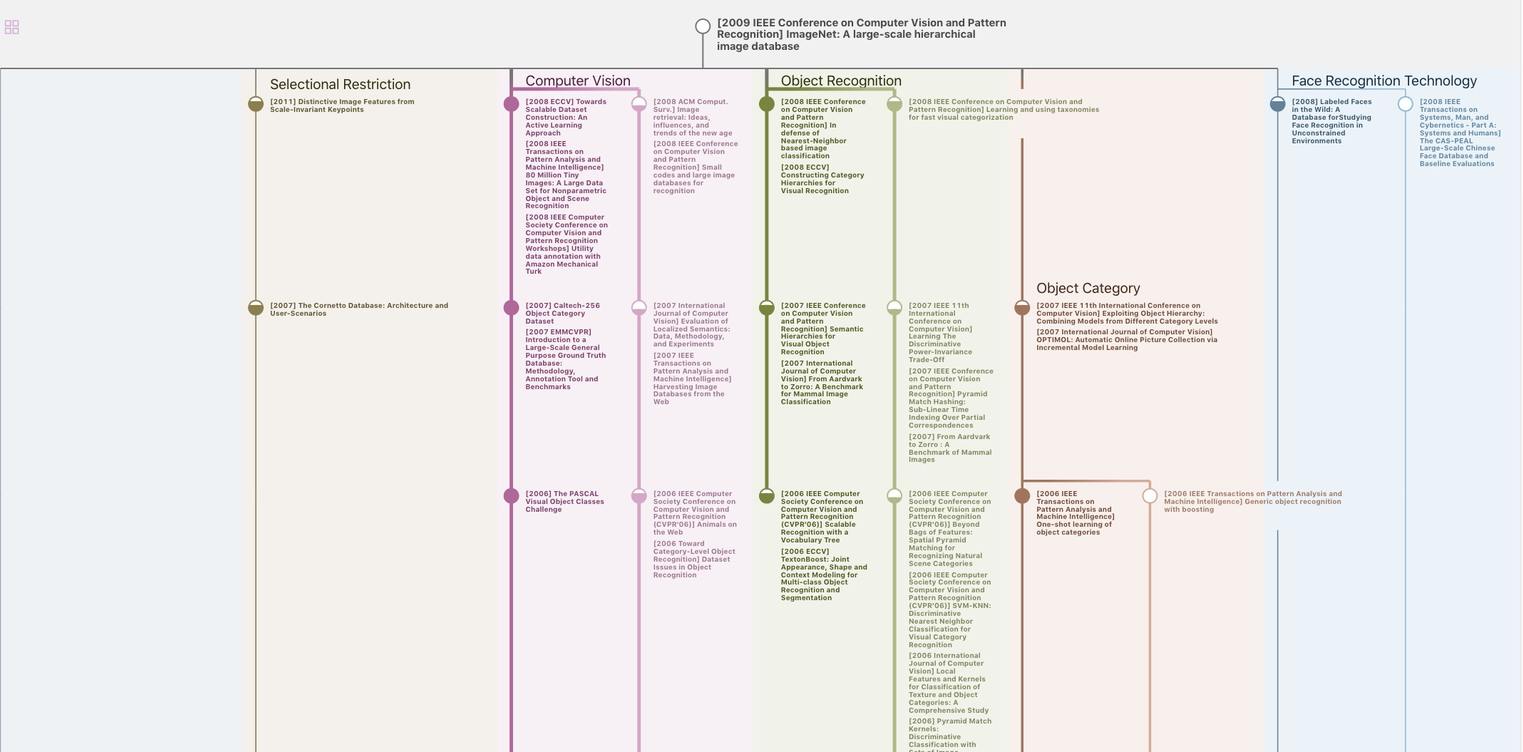
生成溯源树,研究论文发展脉络
Chat Paper
正在生成论文摘要