Sequential Information Guided Sensing
CoRR(2015)
摘要
We study the value of information in sequential compressed sensing by characterizing the performance of sequential information guided sensing in practical scenarios when information is inaccurate. In particular, we assume the signal distribution is parameterized through Gaussian or Gaussian mixtures with estimated mean and covariance matrices, and we can measure compressively through a noisy linear projection or using one-sparse vectors, i.e., observing one entry of the signal each time. We establish a set of performance bounds for the bias and variance of the signal estimator via posterior mean, by capturing the conditional entropy (which is also related to the size of the uncertainty), and the additional power required due to inaccurate information to reach a desired precision. Based on this, we further study how to estimate covariance based on direct samples or covariance sketching. Numerical examples also demonstrate the superior performance of Info-Greedy Sensing algorithms compared with their random and non-adaptive counterparts.
更多查看译文
关键词
sequential information guided
AI 理解论文
溯源树
样例
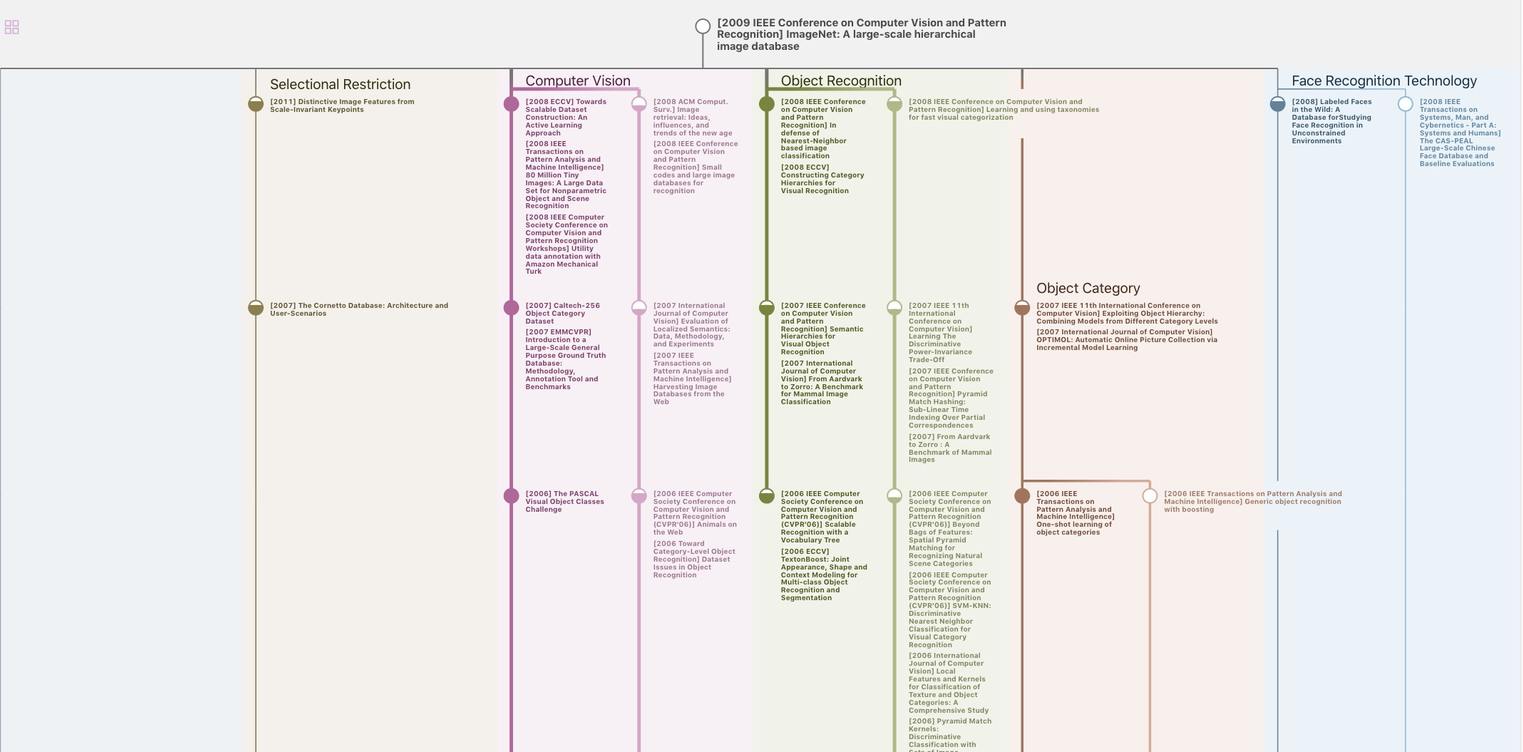
生成溯源树,研究论文发展脉络
Chat Paper
正在生成论文摘要