Linear Shape Deformation Models with Local Support Using Graph-Based Structured Matrix Factorisation
2016 IEEE CONFERENCE ON COMPUTER VISION AND PATTERN RECOGNITION (CVPR)(2016)
摘要
Representing 3D shape deformations by high-dimensional linear models has many applications in computer vision and medical imaging. Commonly, using Principal Components Analysis a low-dimensional subspace of the high-dimensional shape space is determined. However, the resulting factors (the most dominant eigenvectors of the covariance matrix) have global support, i.e. changing the coefficient of a single factor deforms the entire shape. Based on matrix factorisation with sparsity and graph-based regularisation terms, we present a method to obtain deformation factors with local support. The benefits include better flexibility and interpretability as well as the possibility of interactively deforming shapes locally. We demonstrate that for brain shapes our method outperforms the state of the art in local support models with respect to generalisation and sparse reconstruction, whereas for body shapes our method gives more realistic deformations.
更多查看译文
关键词
linear shape deformation model,graph-based regularization,matrix factorization,3D shape deformation representation,computer vision,medical imaging,principal component analysis,PCA,eigenvector,covariance matrix,sparse reconstruction
AI 理解论文
溯源树
样例
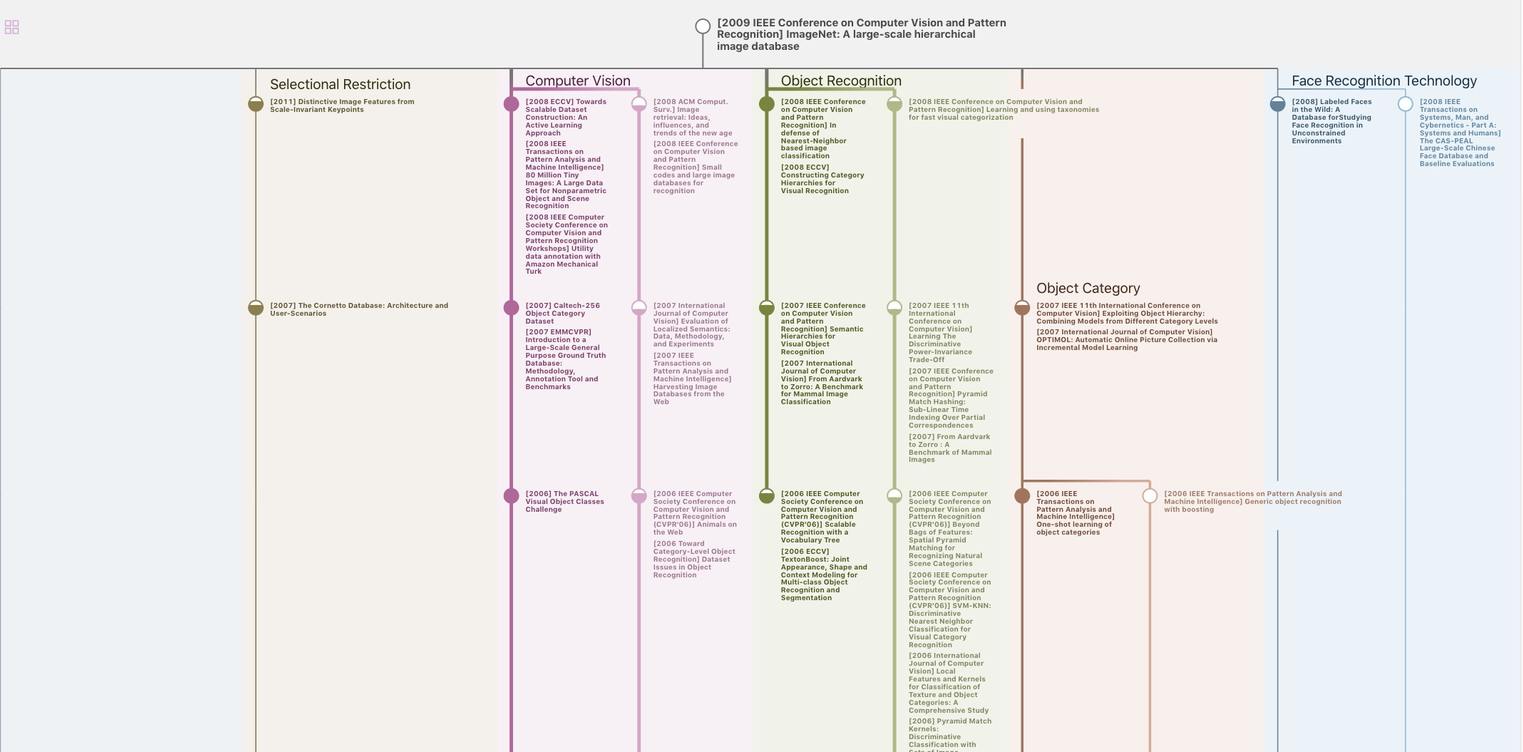
生成溯源树,研究论文发展脉络
Chat Paper
正在生成论文摘要