Optimal Solutions for Adaptive Search Problems with Entropy Objectives
CoRR(2015)
摘要
The problem of searching for an unknown object occurs in important applications ranging from security, medicine and defense. Sensors with the capability to process information rapidly require adaptive algorithms to control their search in response to noisy observations. In this paper, we discuss classes of dynamic, adaptive search problems, and formulate the resulting sensor control problems as stochastic control problems with imperfect information, based on previous work on noisy search problems. The structure of these problems, with objective functions related to information entropy, allows for a complete characterization of the optimal strategies and the optimal cost for the resulting finite-horizon stochastic control problems. We study the problem where an individual sensor is capable of searching over multiple sub-regions in a time, and provide a constructive algorithm for determining optimal policies in real time based on convex optimization. We also study the problem in which there are multiple sensors, each of which is only capable of detecting over one sub-region in a time, jointly searching for an object. Whereas this can be viewed as a special case of our multi-region results, we show that the computation of optimal policies can be decoupled into single-sensor individual scalar convex optimization problems, and provide simple symmetry conditions where the solutions can be determined analytically. We also consider the case where individual sensors can select the accuracy of their sensing modes with different costs, and derive optimal strategies for these problems in terms of the solutions of scalar convex optimization problems. We illustrate our results with experiments using multiple sensors searching for a single object.
更多查看译文
AI 理解论文
溯源树
样例
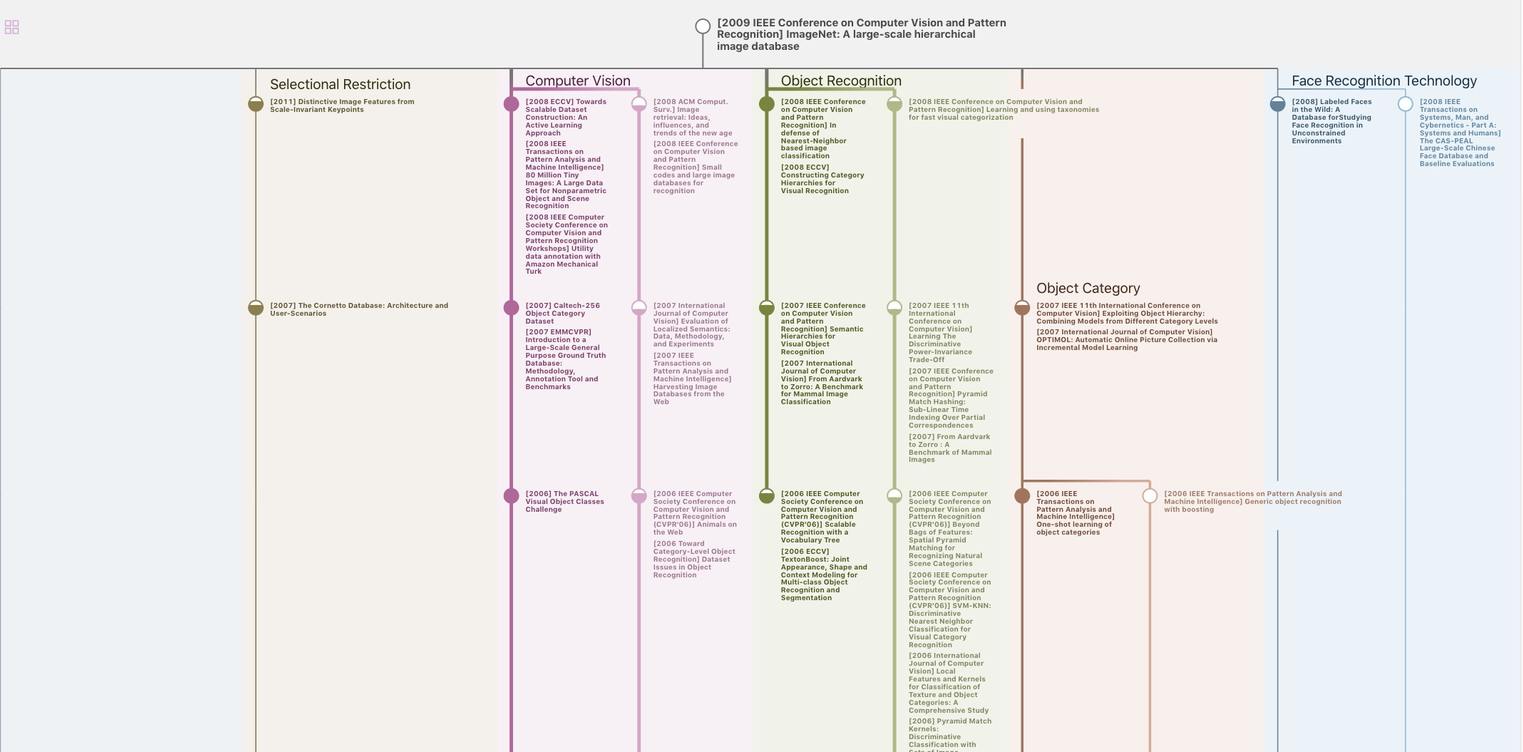
生成溯源树,研究论文发展脉络
Chat Paper
正在生成论文摘要