Fully Convolutional Attention Localization Networks: Efficient Attention Localization for Fine-Grained Recognition.
arXiv: Computer Vision and Pattern Recognition(2016)
摘要
Fine-grained recognition is challenging mainly because the inter-class differences between fine-grained classes are usually local and subtle while intra-class differences could be large due to pose variations. In order to distinguish them from intra-class variations, it is essential to zoom in on highly discriminative local regions. In this work, we introduce a reinforcement learning-based fully convolutional attention localization network to adaptively select multiple task-driven visual attention regions. We show that zooming in on the selected attention regions significantly improves the performance of fine-grained recognition. Compared to previous reinforcement learning-based models, the proposed approach is noticeably more computationally efficient during both training and testing because of its fully-convolutional architecture, and it is capable of simultaneous focusing its glimpse on multiple visual attention regions. The experiments demonstrate that the proposed method achieves notably higher classification accuracy on three benchmark fine-grained recognition datasets: Stanford Dogs, Stanford Cars, and CUB-200-2011.
更多查看译文
AI 理解论文
溯源树
样例
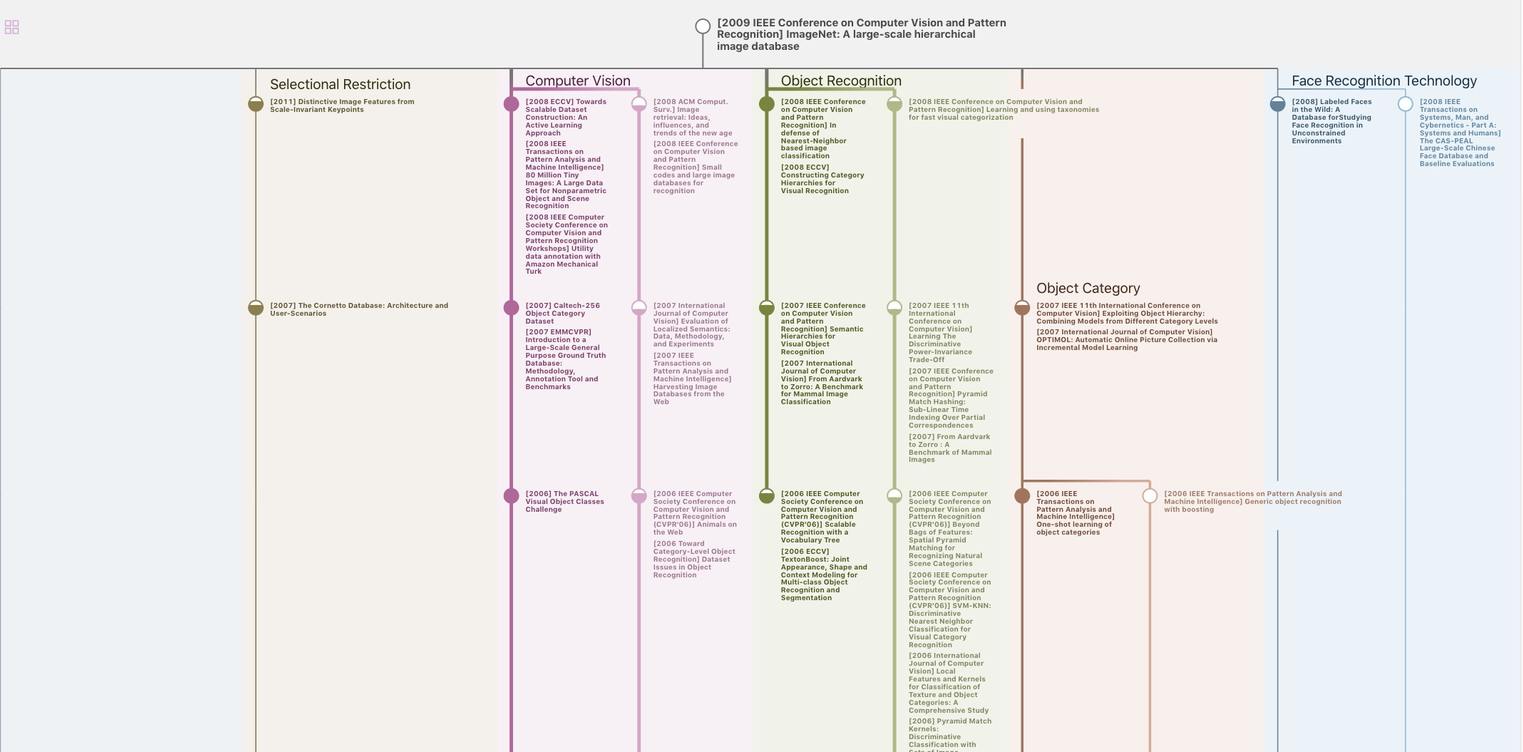
生成溯源树,研究论文发展脉络
Chat Paper
正在生成论文摘要