Approximation with random bases: Pro et Contra.
Information Sciences(2016)
摘要
In this work we discuss the problem of selecting suitable approximators from families of parameterized elementary functions that are known to be dense in a Hilbert space of functions. We consider and analyze published procedures, both randomized and deterministic, for selecting elements from these families that have been shown to ensure the rate of convergence in L2 norm of order O(1/N), where N is the number of elements. We show that both randomized and deterministic procedures are successful if additional information about the families of functions to be approximated is provided. In the absence of such additional information one may observe exponential growth of the number of terms needed to approximate the function and/or extreme sensitivity of the outcome of the approximation to parameters. Implications of our analysis for applications of neural networks in modeling and control are illustrated with examples.
更多查看译文
关键词
Random bases,Measure concentration,Neural networks,Approximation
AI 理解论文
溯源树
样例
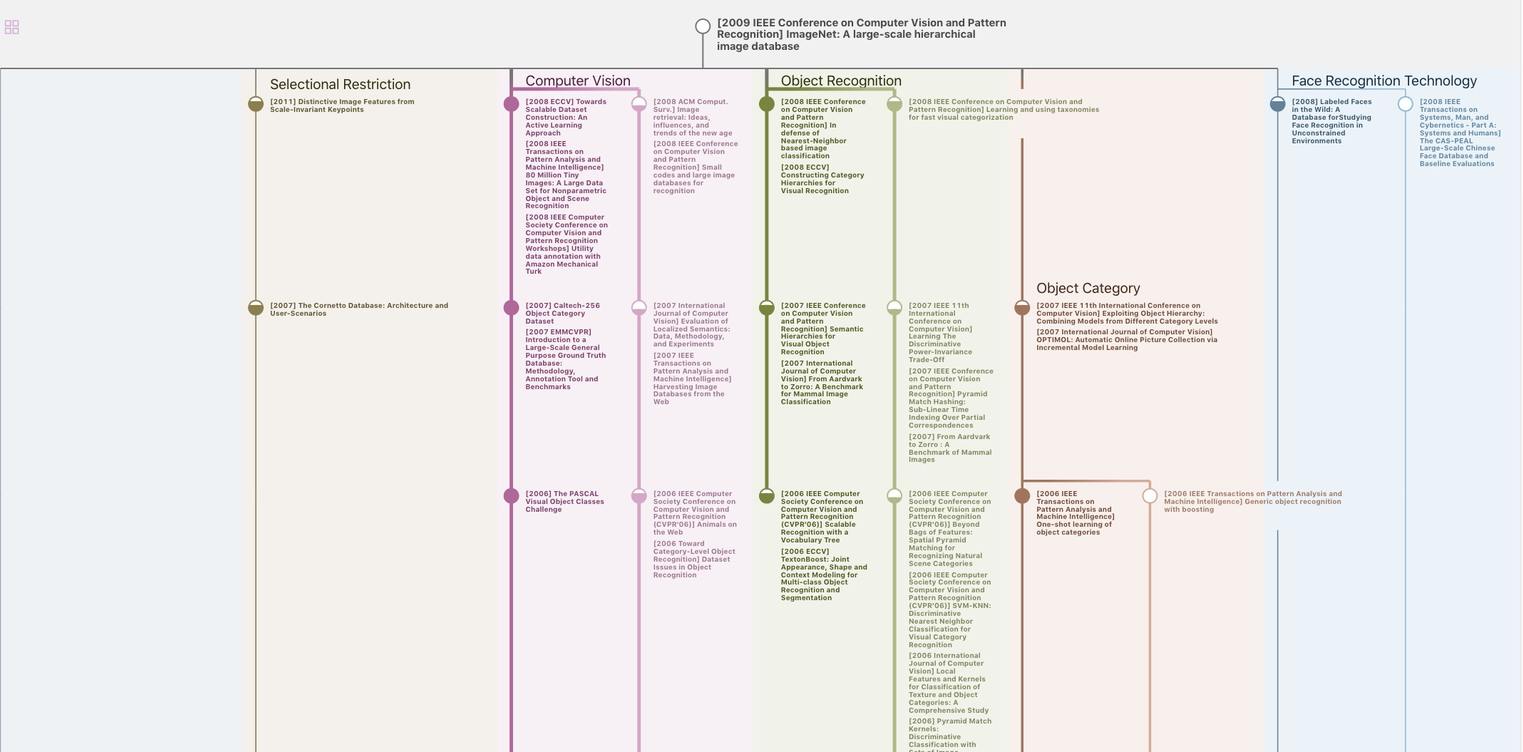
生成溯源树,研究论文发展脉络
Chat Paper
正在生成论文摘要