Efficient Frequent Directions Algorithm for Sparse Matrices
KDD '16: The 22nd ACM SIGKDD International Conference on Knowledge Discovery and Data Mining San Francisco California USA August, 2016(2016)
摘要
This paper describes Sparse Frequent Directions, a variant of Frequent Directions for sketching sparse matrices. It resembles the original algorithm in many ways: both receive the rows of an input matrix $A^{n \times d}$ one by one in the streaming setting and compute a small sketch $B \in R^{\ell \times d}$. Both share the same strong (provably optimal) asymptotic guarantees with respect to the space-accuracy tradeoff in the streaming setting. However, unlike Frequent Directions which runs in $O(nd\ell)$ time regardless of the sparsity of the input matrix $A$, Sparse Frequent Directions runs in $\tilde{O} (nnz(A)\ell + n\ell^2)$ time. Our analysis loosens the dependence on computing the Singular Value Decomposition (SVD) as a black box within the Frequent Directions algorithm. Our bounds require recent results on the properties of fast approximate SVD computations. Finally, we empirically demonstrate that these asymptotic improvements are practical and significant on real and synthetic data.
更多查看译文
关键词
Frequent Directions,Sparse Matrix,Matrix Sketching
AI 理解论文
溯源树
样例
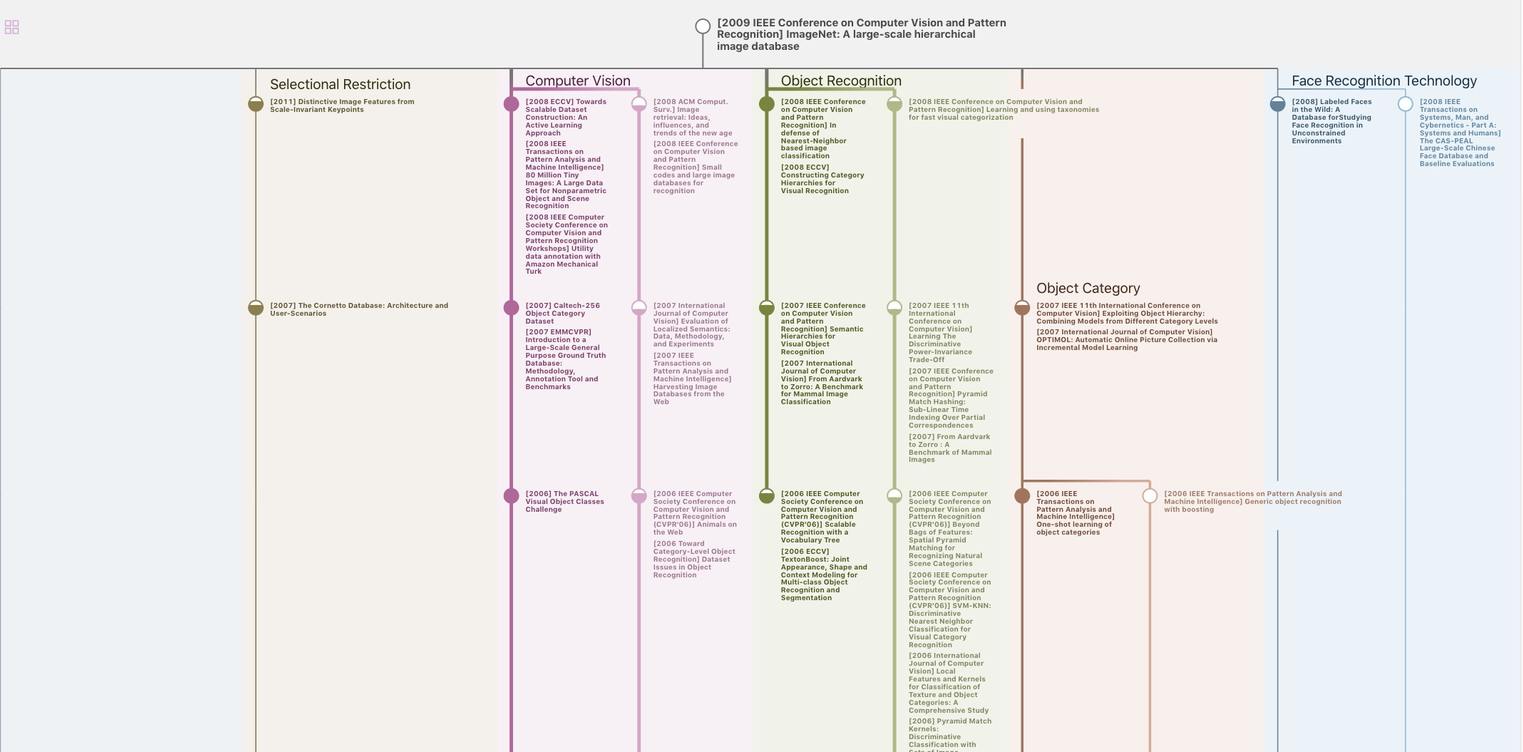
生成溯源树,研究论文发展脉络
Chat Paper
正在生成论文摘要