Dropout Versus Weight Decay for Deep Networks.
arXiv: Learning(2016)
摘要
study dropout and weight decay applied to deep networks with rectified linear units and the quadratic loss. We show how using dropout in this context can be viewed as adding a regularization penalty term that grows exponentially with the depth of the network when the more traditional weight decay penalty grows polynomially. then show how this difference affects the inductive bias of algorithms using one regularizer or the other: we describe a random source of data that dropout is unwilling to fit, but that is compatible with the inductive bias of weight decay. also describe a source that is compatible with the inductive bias of dropout, but not weight decay. We also show that, in contrast with the case of generalized linear models, when used with deep networks with rectified linear units and the quadratic loss, the regularization penalty of dropout (a) is not only a function of the marginals on the independent variables, but also depends on the response variables, and (b) can be negative. Finally, the dropout penalty can drive a learning algorithm to use negative weights even when trained with monotone training data.
更多查看译文
AI 理解论文
溯源树
样例
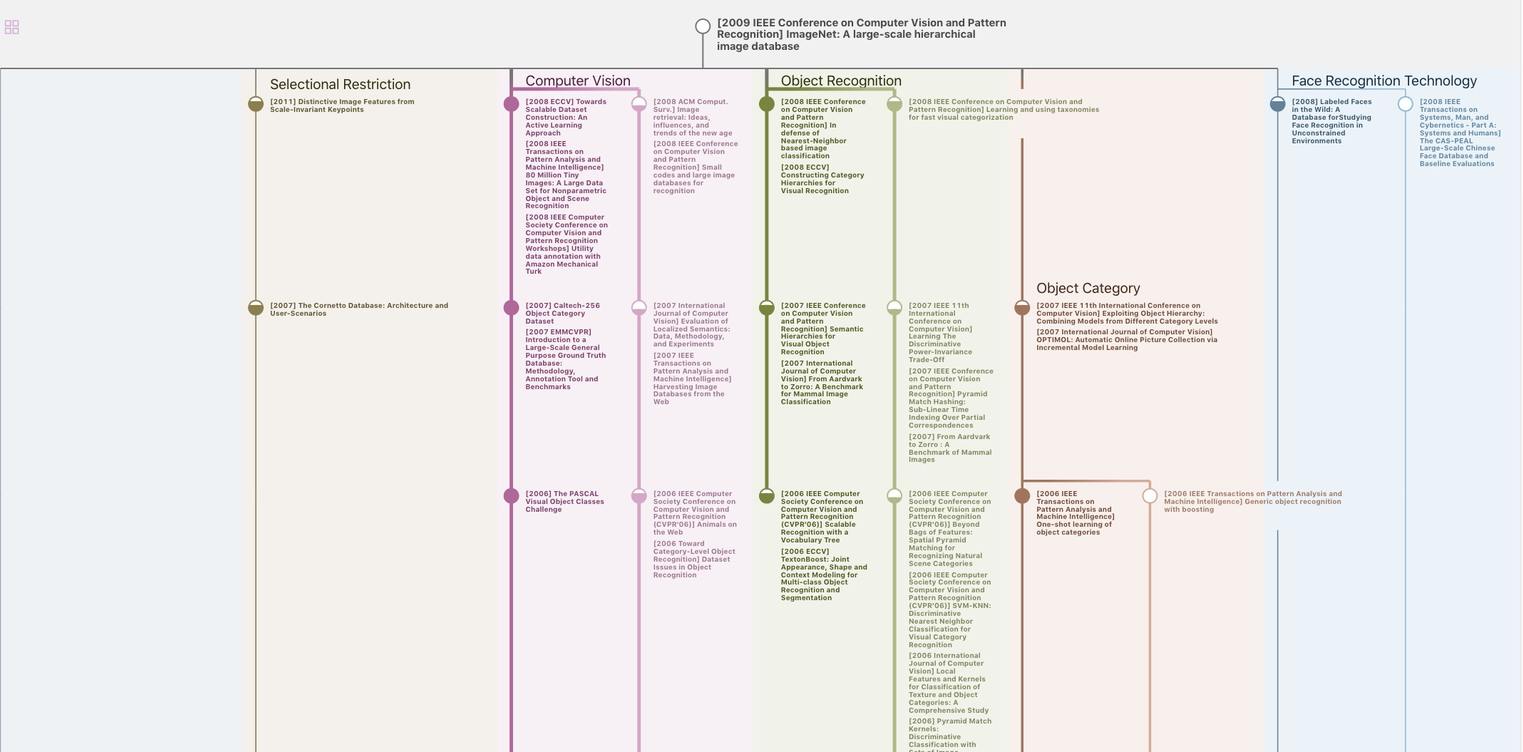
生成溯源树,研究论文发展脉络
Chat Paper
正在生成论文摘要