Efficient Convolutional Neural Networks For Pixelwise Classification On Heterogeneous Hardware Systems
2016 IEEE 13th International Symposium on Biomedical Imaging (ISBI)(2016)
摘要
With recent advances in high-throughput Electron Microscopy (EM) imaging it is now possible to image an entire nervous system of organisms like Drosophila melanogaster. One of the bottlenecks to reconstruct a connectome from these large volumes (approximate to 100 TiB) is the pixel-wise prediction of membranes. The time it would typically take to process such a volume using a convolutional neural network (CNN) with a sliding window approach is in the order of years on a current GPU. With sliding windows, however, a lot of redundant computations are carried out. In this paper, we present an extension to the Caffe library to increase throughput by predicting many pixels at once. On a sliding window network successfully used for membrane classification, we show that our method achieves a speedup of up to 57x, maintaining identical prediction results.
更多查看译文
关键词
convolutional neural networks,pixel wise classification,electron microscopy,loss functions
AI 理解论文
溯源树
样例
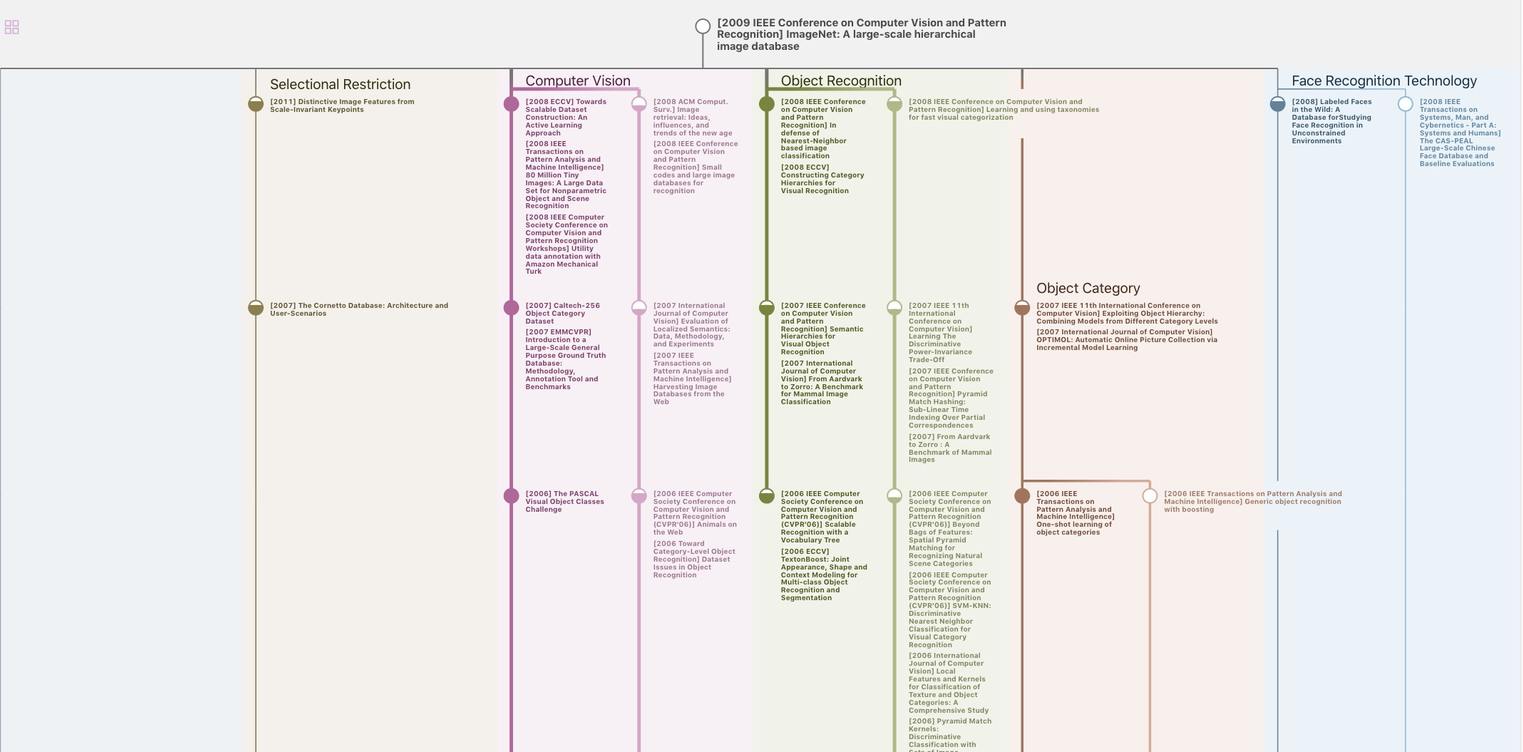
生成溯源树,研究论文发展脉络
Chat Paper
正在生成论文摘要