Modeling and evaluating Gaussian mixture model based on motion granularity
Intelligent Service Robotics(2016)
摘要
To model manipulation tasks, we propose a novel method for learning manipulation skills based on the degree of motion granularity. Even though manipulation tasks usually consist of a mixture of fine-grained and coarse-grained movements, to the best of our knowledge, manipulation skills have so far been modeled without considering their motion granularity. To model such a manipulation skill, Gaussian mixture models (GMMs) have been represented using several well-known techniques such as principal component analysis, k -means, Bayesian information criterion, and expectation-maximization (EM) algorithms. However, in this GMM, there is a problem in that when a mixture of fine-grained and coarse-grained movements is modeled as a GMM, fine-grained movements tend to be poorly represented. To resolve this issue, we measure a continuous degree of motion granularity for every time step of a manipulation task from a GMM. Then, we remodel the GMM by weighting a conventional k -means algorithm with motion granularity. Finally, we also estimate the parameters of the GMM by weighting the conventional EM with motion granularity. To validate our proposed method, we evaluate the GMM estimated using our proposed method by comparing it with those estimated by different GMMs in terms of inference, regression, and generalization using a robot arm that performs two daily tasks, namely decorating a very small area and passing through a narrow tunnel.
更多查看译文
关键词
Motion granularity,Gaussian mixture model,Weighted k-means,Weighted EM
AI 理解论文
溯源树
样例
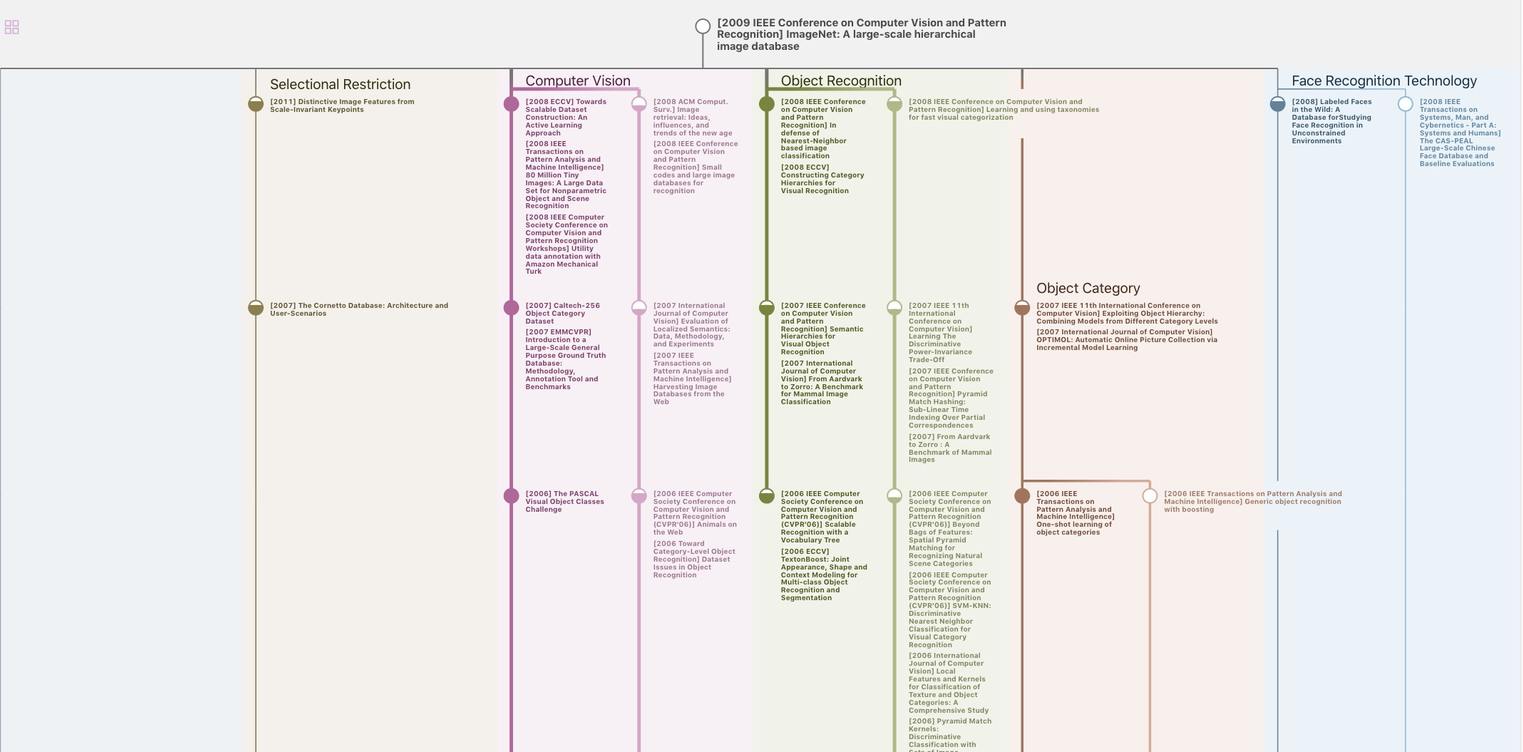
生成溯源树,研究论文发展脉络
Chat Paper
正在生成论文摘要