Parameterization of the InVEST Crop Pollination Model to spatially predict abundance of wild blueberry (Vaccinium angustifolium Aiton) native bee pollinators in Maine, USA.
Environmental Modelling and Software(2016)
摘要
Non-native honeybees historically have been managed for crop pollination, however, recent population declines draw attention to pollination services provided by native bees. We applied the InVEST Crop Pollination model, developed to predict native bee abundance from habitat resources, in Maine's wild blueberry crop landscape. We evaluated model performance with parameters informed by four approaches: 1) expert opinion; 2) sensitivity analysis; 3) sensitivity analysis informed model optimization; and, 4) simulated annealing (uninformed) model optimization. Uninformed optimization improved model performance by 29% compared to expert opinion-informed model, while sensitivity-analysis informed optimization improved model performance by 54%. This suggests that expert opinion may not result in the best parameter values for the InVEST model. The proportion of deciduous/mixed forest within 2000¿m of a blueberry field also reliably predicted native bee abundance in blueberry fields, however, the InVEST model provides an efficient tool to estimate bee abundance beyond the field perimeter. The expert opinion-informed InVEST model was less reliable than the sensitivity analyses-informed model.Proportion of deciduous/mixed forest within 2000¿m also predicted within-field bee abundance.InVEST provides reliable bee abundance predictions at the landscape extent in Maine.
更多查看译文
关键词
Bee community,Prediction,Model,Maine,Blueberry,Landscape,Expert
AI 理解论文
溯源树
样例
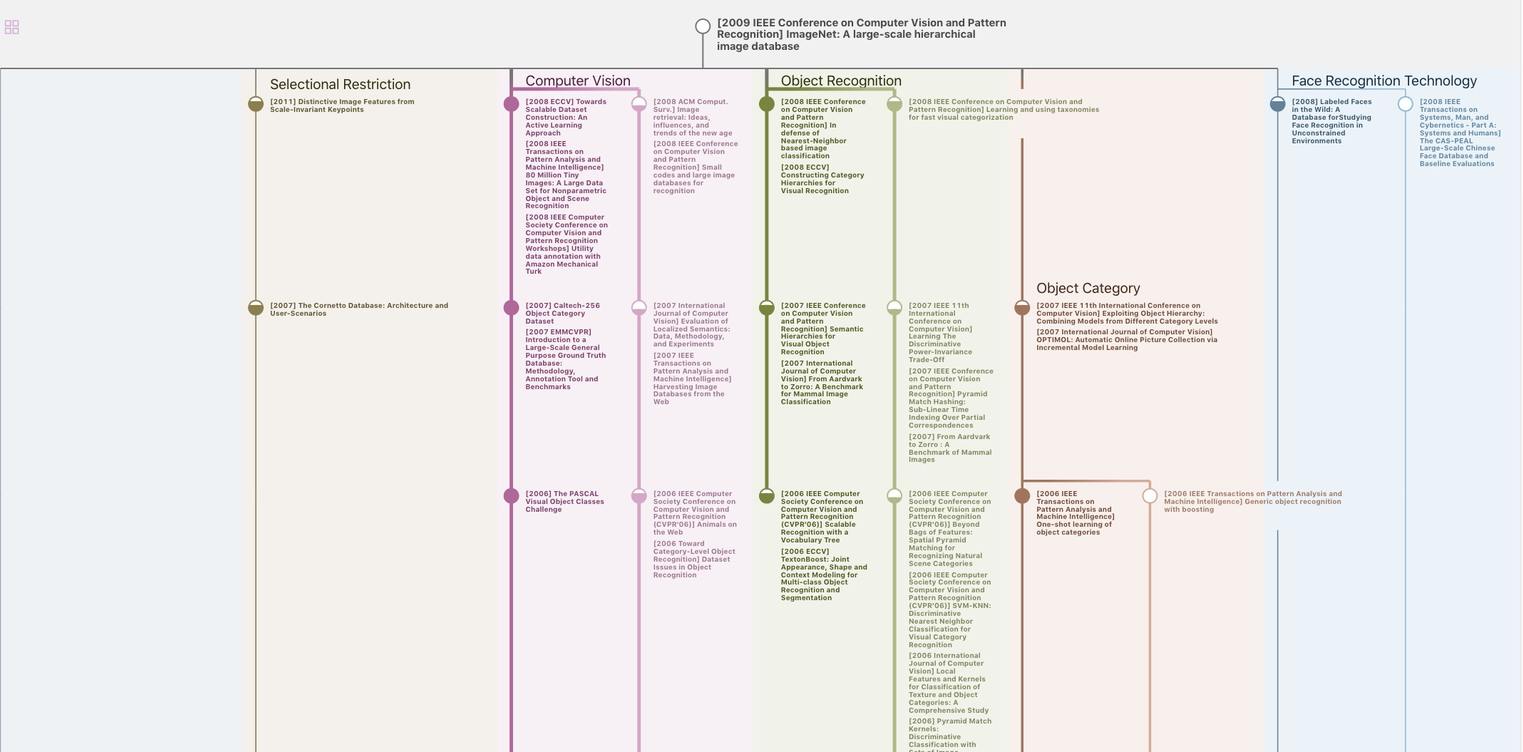
生成溯源树,研究论文发展脉络
Chat Paper
正在生成论文摘要