Factors important for good visualisation of time series
Periodicals(2016)
摘要
AbstractWe create an artificial neural network which is a version of echo state networks, ESNs. ESNs are recurrent neural networks but unlike most recurrent networks, they come with an efficient training method. We have previously Wang et al., 2011 adapted this method using ideas from neuroscale Tipping, 1996 so that the network can be used for projecting multivariate time series data onto a low dimensional manifold so that the structure in the time series can be identified by eye. In this paper, we review work on a minimal architecture echo state machine Wang et al., 2011 in the context of visualisation and show that it does not perform as well as the original. We then discuss three factors which may affect the capability of the network - its structure, size and sparsity - and show that, of these three, by far the most important is the size of the reservoir of neurons.
更多查看译文
关键词
echo state machines, visualisation, multidimensional scaling
AI 理解论文
溯源树
样例
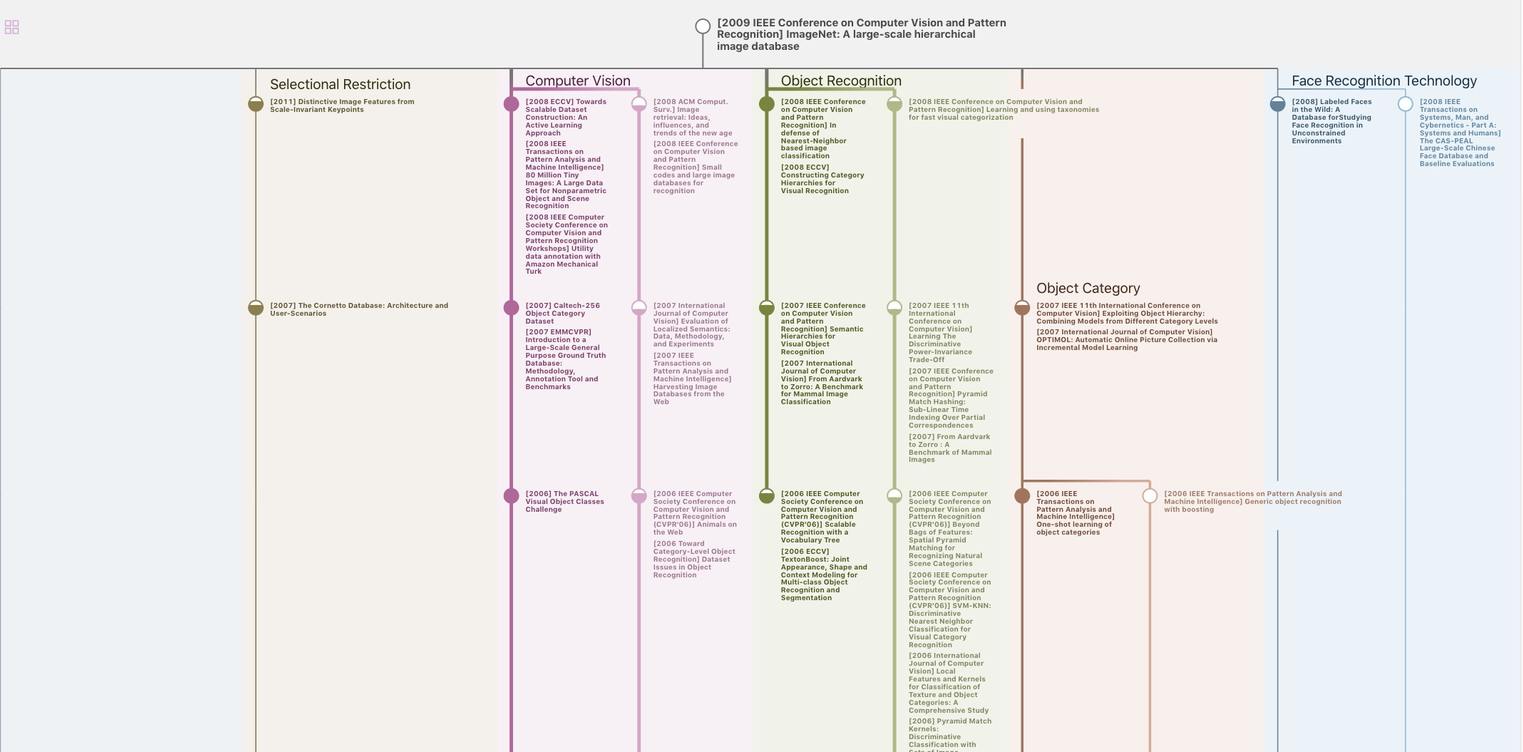
生成溯源树,研究论文发展脉络
Chat Paper
正在生成论文摘要