Statistical Evidence Measured on a Properly Calibrated Scale for Multinomial Hypothesis Comparisons.
ENTROPY(2016)
摘要
Measurement of the strength of statistical evidence is a primary objective of statistical analysis throughout the biological and social sciences. Various quantities have been proposed as definitions of statistical evidence, notably the likelihood ratio, the Bayes factor and the relative belief ratio. Each of these can be motivated by direct appeal to intuition. However, for an evidence measure to be reliably used for scientific purposes, it must be properly calibrated, so that one "degree" on the measurement scale always refers to the same amount of underlying evidence, and the calibration problem has not been resolved for these familiar evidential statistics. We have developed a methodology for addressing the calibration issue itself, and previously applied this methodology to derive a calibrated evidence measure E in application to a broad class of hypothesis contrasts in the setting of binomial (single-parameter) likelihoods. Here we substantially generalize previous results to include the m-dimensional multinomial (multiple-parameter) likelihood. In the process we further articulate our methodology for addressing the measurement calibration issue, and we show explicitly how the more familiar definitions of statistical evidence are patently not well behaved with respect to the underlying evidence. We also continue to see striking connections between the calculating equations for E and equations from thermodynamics as we move to more complicated forms of the likelihood.
更多查看译文
关键词
statistical evidence,information dynamics,entropy,thermodynamics,multinomial distribution
AI 理解论文
溯源树
样例
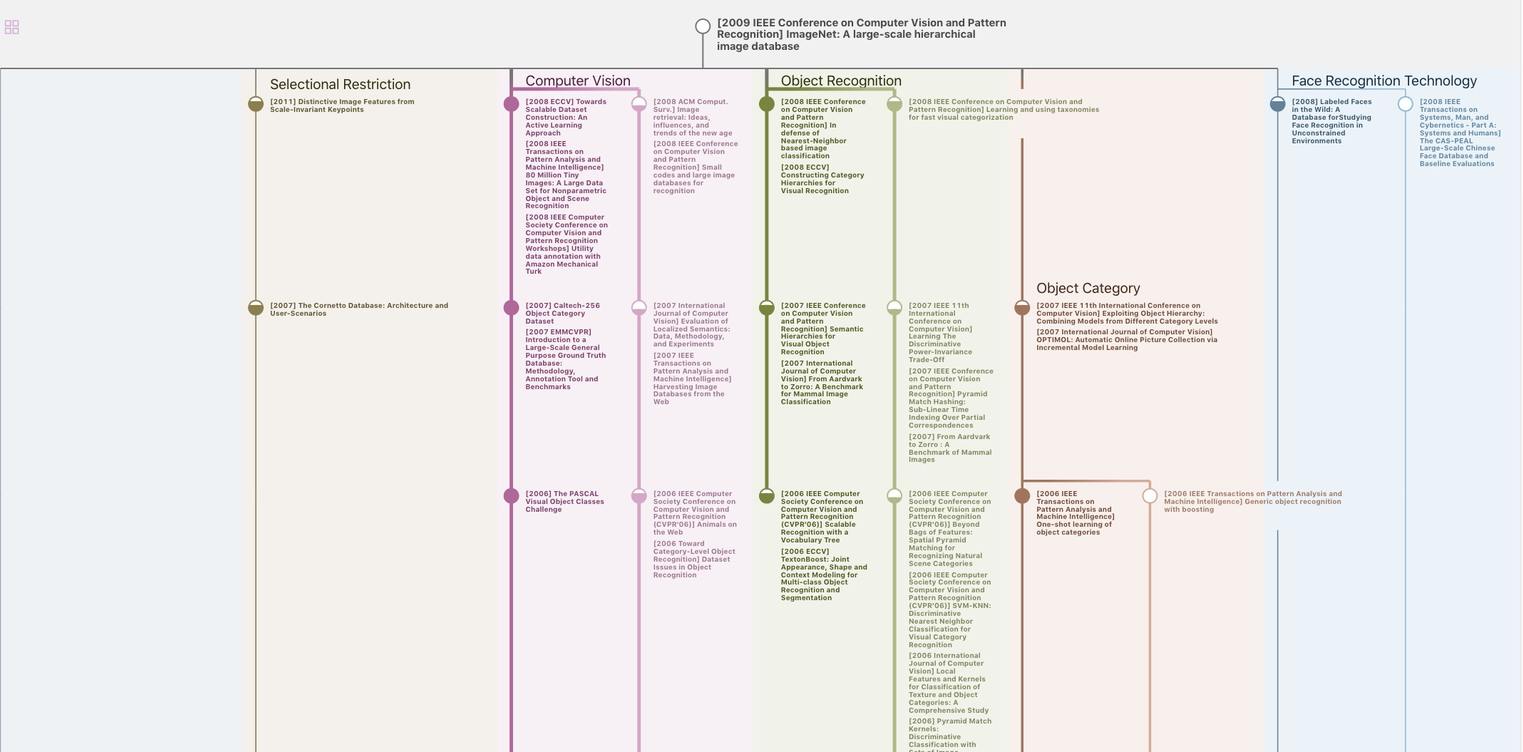
生成溯源树,研究论文发展脉络
Chat Paper
正在生成论文摘要