An Empirical Study of Skew-Insensitive Splitting Criteria and Its Application in Traditional Chinese Medicine
Intelligent Automation & Soft Computing(2014)
关键词
Imbalanced learning,Skew-insensitive splitting criteria,Decision trees,Chronic obstructive pulmonary disease
AI 理解论文
溯源树
样例
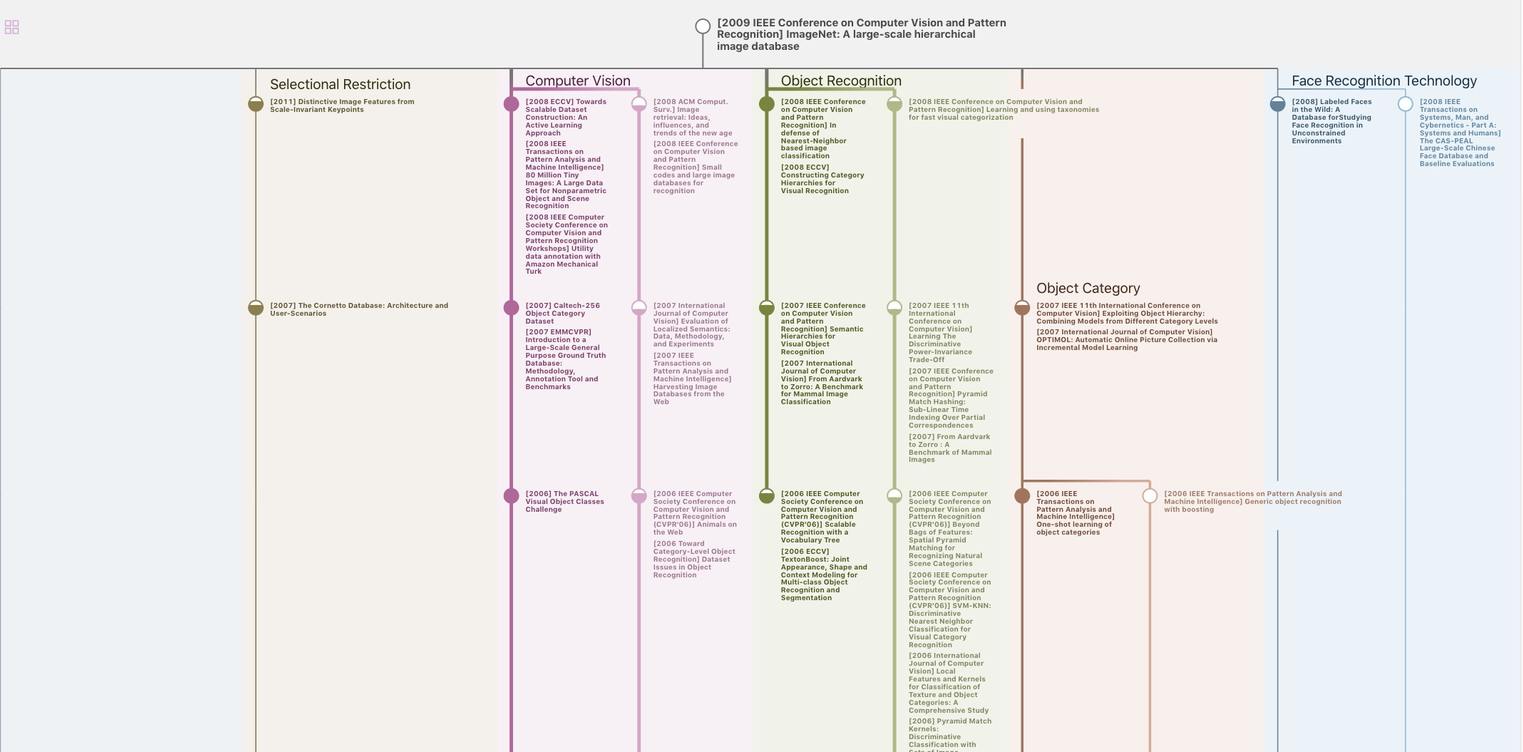
生成溯源树,研究论文发展脉络
Chat Paper
正在生成论文摘要