Learning class-specific descriptors for deformable shapes using localized spectral convolutional networks
Computer Graphics Forum(2015)
摘要
In this paper, we propose a generalization of convolutional neural networks CNN to non-Euclidean domains for the analysis of deformable shapes. Our construction is based on localized frequency analysis a generalization of the windowed Fourier transform to manifolds that is used to extract the local behavior of some dense intrinsic descriptor, roughly acting as an analogy to patches in images. The resulting local frequency representations are then passed through a bank of filters whose coefficient are determined by a learning procedure minimizing a task-specific cost. Our approach generalizes several previous methods such as HKS, WKS, spectral CNN, and GPS embeddings. Experimental results show that the proposed approach allows learning class-specific shape descriptors significantly outperforming recent state-of-the-art methods on standard benchmarks.
更多查看译文
AI 理解论文
溯源树
样例
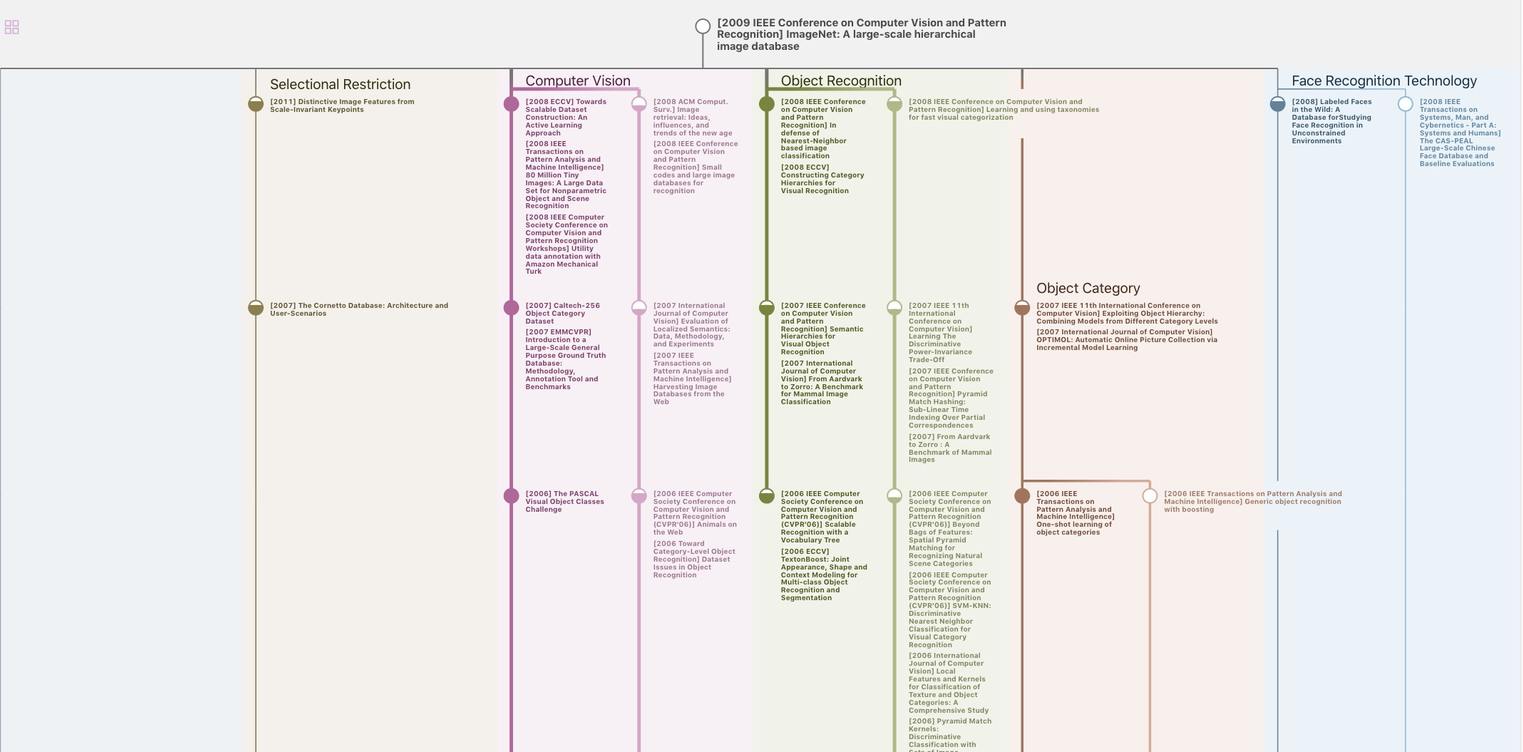
生成溯源树,研究论文发展脉络
Chat Paper
正在生成论文摘要