Lanczos and the Riemannian SVD in information retrieval applications
NUMERICAL LINEAR ALGEBRA WITH APPLICATIONS(2005)
摘要
Variations of the latent semantic indexing (LSI) method in information retrieval (IR) require the computation of singular subspaces associated with the k dominant singular values of a large m x 11 sparse matrix A, where k << min(m,n). The Riemannian SVD was recently generalized to low-rank matrices arising in IR and shown to be an effective approach for formulating an enhanced semantic model that captures the latent term-document structure of the data. However, in terms of storage and computation requirements, its implementation can be much improved for large-scale applications. We discuss an efficient and reliable algorithm, called SPK-RSVD-LS], as an alternative approach for deriving the enhanced semantic model. The algorithm combines the generalized Riemannian SVD and the Lanczos method with full reorthogonalization and explicit restart strategies. We demonstrate that our approach performs as well as the original low-rank Riemannian SVD method by comparing their retrieval performance on a well-known benchmark document collection. Copyright (c) 2004 John Wiley & Sons, Ltd.
更多查看译文
关键词
information retrieval,latent semantic indexing,Lanezos method,singular value decomposition,sparse
AI 理解论文
溯源树
样例
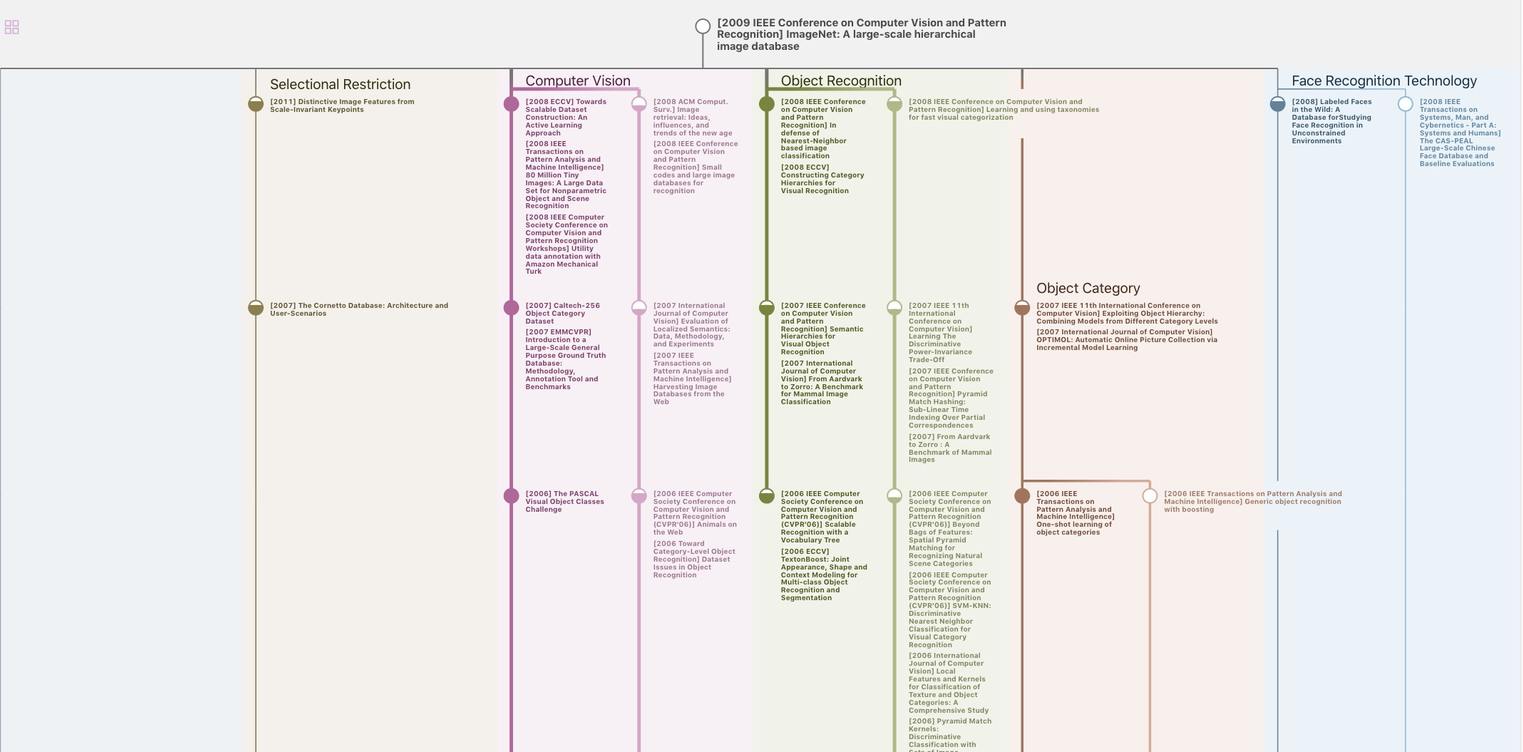
生成溯源树,研究论文发展脉络
Chat Paper
正在生成论文摘要