Deep Dependency Substructure-Based Learning for Multidocument Summarization
ACM Trans. Inf. Syst.(2015)
摘要
Most extractive style topic-focused multidocument summarization systems generate a summary by ranking textual units in multiple documents and extracting a proper subset of sentences biased to the given topic. Usually, the textual units are simply represented as sentences or n-grams, which do not carry deep syntactic and semantic information. This article presents a novel extractive topic-focused multidocument summarization framework. The framework proposes a new kind of more meaningful and informative units named frequent Deep Dependency Sub-Structure (DDSS) and a topic-sensitive Multi-Task Learning (MTL) model for frequent DDSS ranking. Given a document set, first, we parse all the sentences into deep dependency structures with a Head-driven Phrase Structure Grammar (HPSG) parser and mine the frequent DDSSs after semantic normalization. Then we employ a topic-sensitive MTL model to learn the importance of these frequent DDSSs. Finally, we exploit an Integer Linear Programming (ILP) formulation and use the frequent DDSSs as the essentials for summary extraction. Experimental results on two DUC datasets demonstrate that our proposed approach can achieve state-of-the-art performance. Both the DDSS information and the topic-sensitive MTL model are validated to be very helpful for topic-focused multidocument summarization.
更多查看译文
关键词
Algorithms,Experimentation,Document summarization,deep dependency sub-structure,multi-task learning
AI 理解论文
溯源树
样例
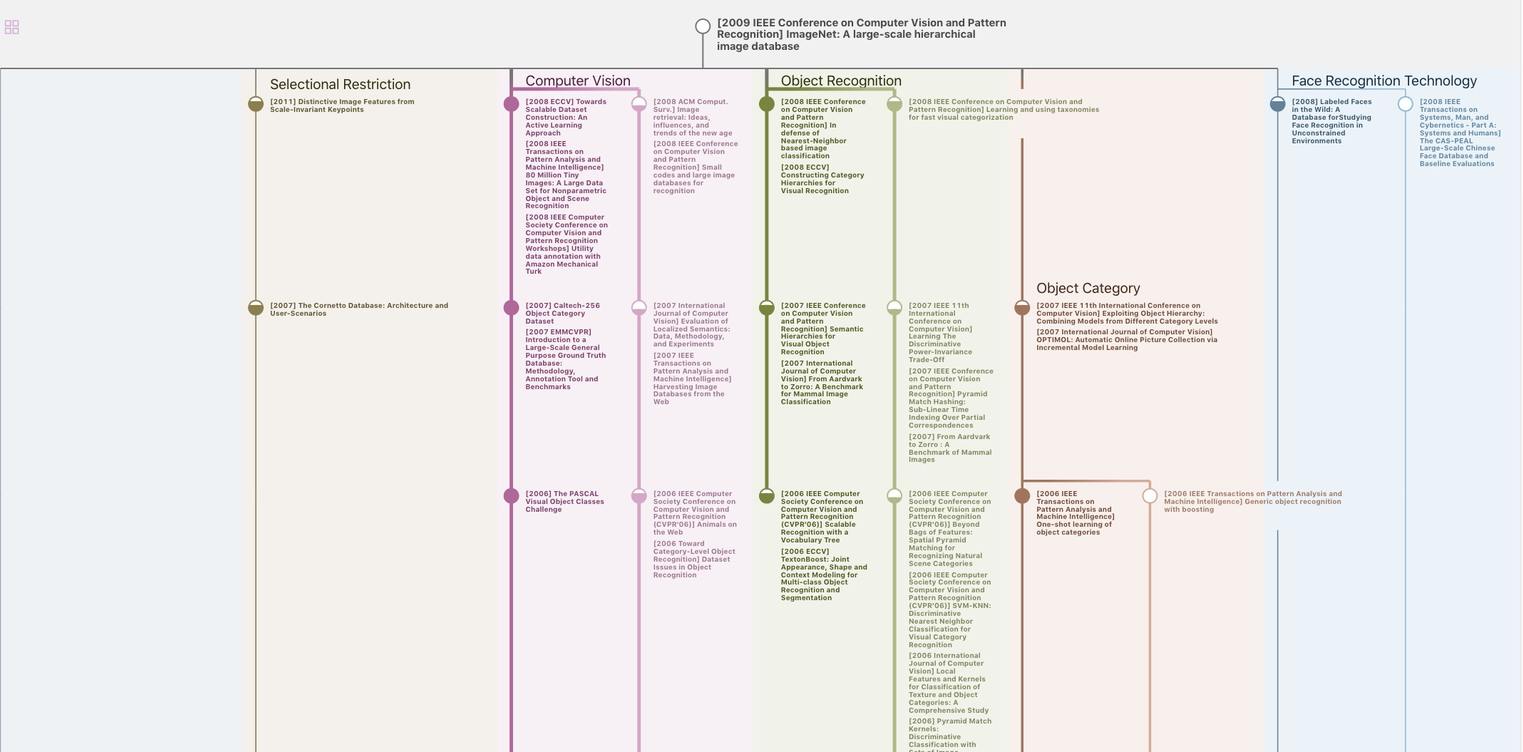
生成溯源树,研究论文发展脉络
Chat Paper
正在生成论文摘要