Can high-order dependencies improve mutual information based feature selection?
Pattern Recognition(2016)
摘要
Mutual information (MI) based approaches are a popular paradigm for feature selection. Most previous methods have made use of low-dimensional MI quantities that are only effective at detecting low-order dependencies between variables. Several works have considered the use of higher dimensional mutual information, but the theoretical underpinning of these approaches is not yet comprehensive. To fill this gap, in this paper, we systematically investigate the issues of employing high-order dependencies for mutual information based feature selection. We first identify a set of assumptions under which the original high-dimensional mutual information based criterion can be decomposed into a set of low-dimensional MI quantities. By relaxing these assumptions, we arrive at a principled approach for constructing higher dimensional MI based feature selection methods that takes into account higher order feature interactions. Our extensive experimental evaluation on real data sets provides concrete evidence that methodological inclusion of high-order dependencies improve MI based feature selection.
更多查看译文
关键词
Feature selection,Mutual information,High-order dependency
AI 理解论文
溯源树
样例
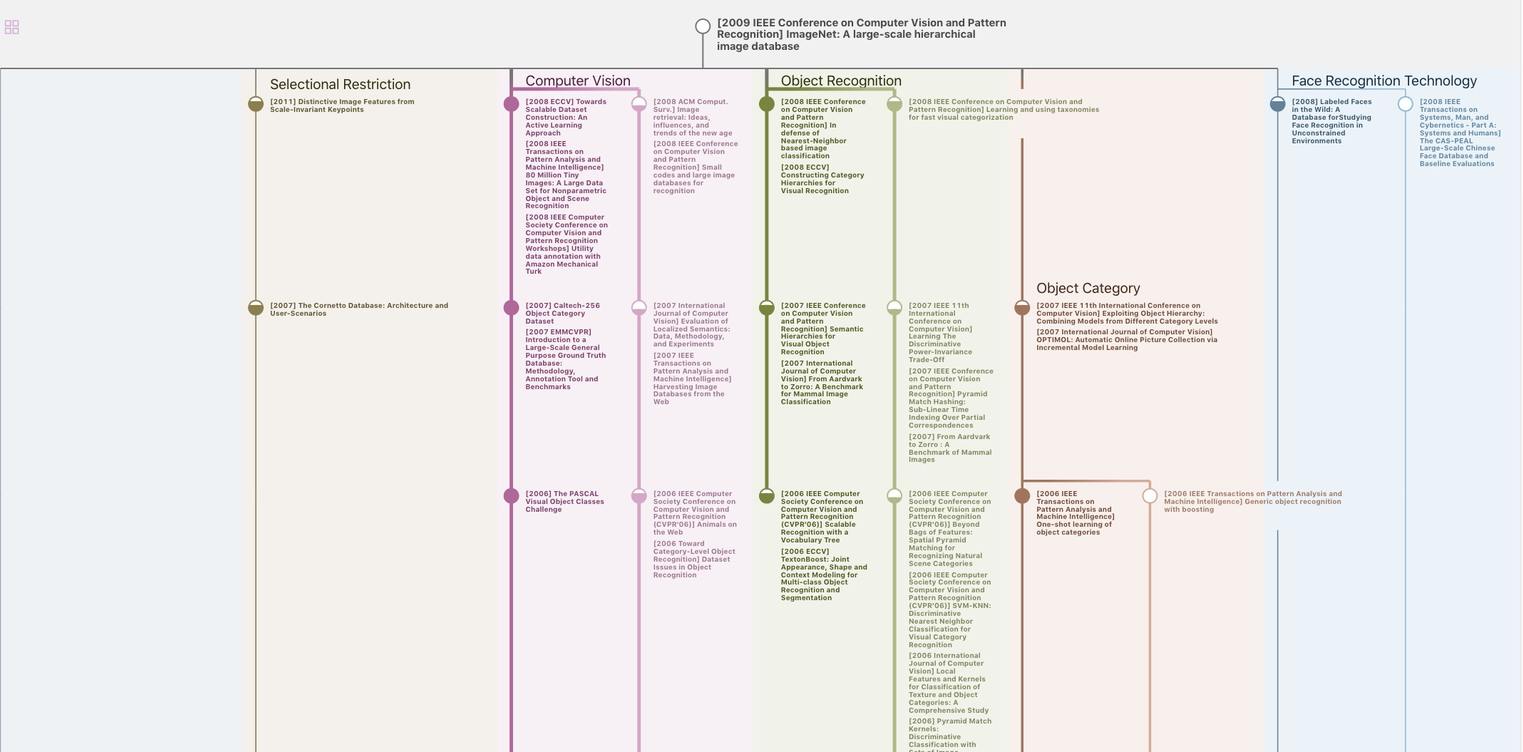
生成溯源树,研究论文发展脉络
Chat Paper
正在生成论文摘要