Weighted Multi-view Clustering with Feature Selection.
Pattern Recognition(2016)
摘要
In recent years, combining multiple sources or views of datasets for data clustering has been a popular practice for improving clustering accuracy. As different views are different representations of the same set of instances, we can simultaneously use information from multiple views to improve the clustering results generated by the limited information from a single view. Previous studies mainly focus on the relationships between distinct data views, which would get some improvement over the single-view clustering. However, in the case of high-dimensional data, where each view of data is of high dimensionality, feature selection is also a necessity for further improving the clustering results. To overcome this problem, this paper proposes a novel algorithm termed Weighted Multi-view Clustering with Feature Selection (WMCFS) that can simultaneously perform multi-view data clustering and feature selection. Two weighting schemes are designed that respectively weight the views of data points and feature representations in each view, such that the best view and the most representative feature space in each view can be selected for clustering. Experimental results conducted on real-world datasets have validated the effectiveness of the proposed method.
更多查看译文
关键词
Data clustering,Multi-view,Feature selection,Weighting
AI 理解论文
溯源树
样例
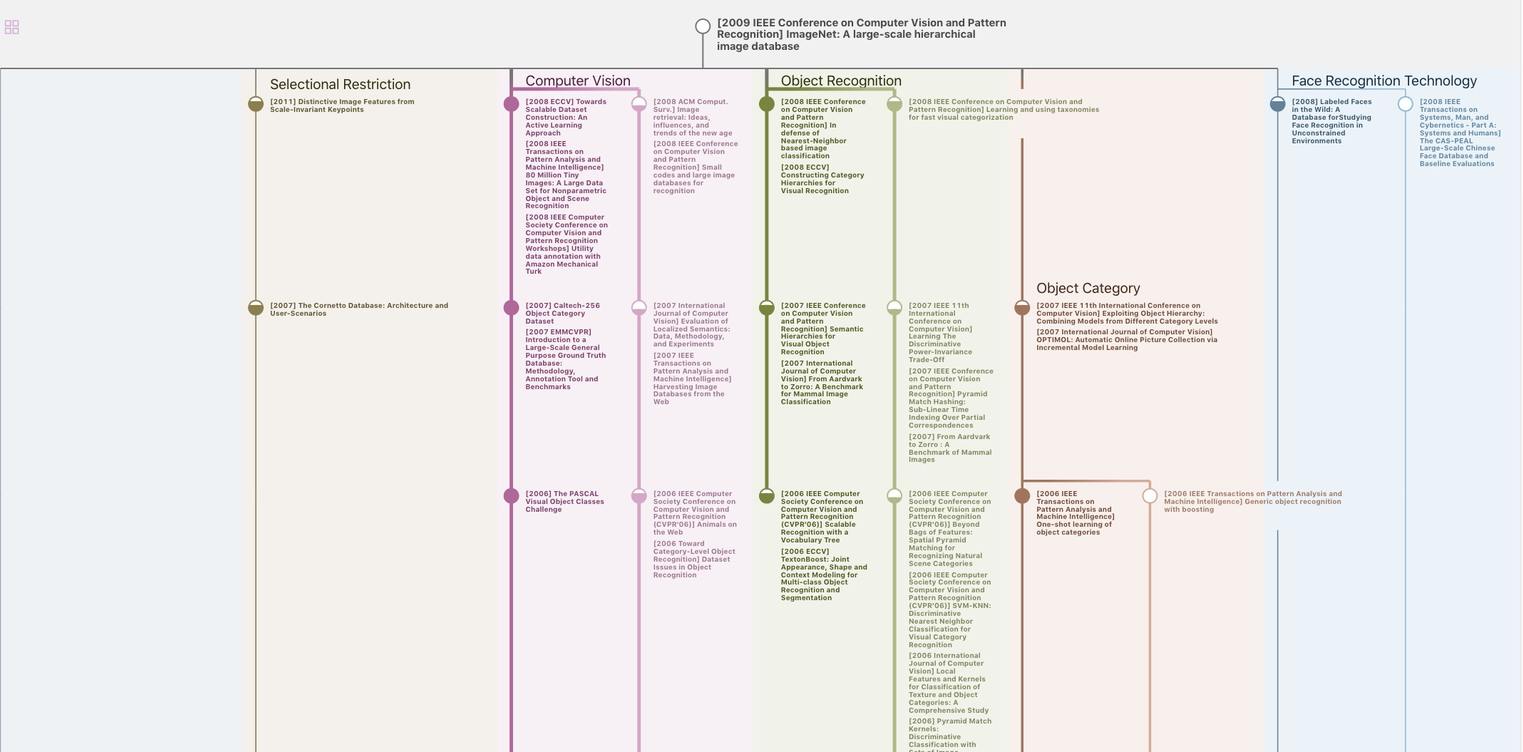
生成溯源树,研究论文发展脉络
Chat Paper
正在生成论文摘要