Predicting User Behavior In Display Advertising Via Dynamic Collective Matrix Factorization
SIGIR '15: The 38th International ACM SIGIR conference on research and development in Information Retrieval Santiago Chile August, 2015(2015)
摘要
Conversion prediction and click prediction are two important and intertwined problems in display advertising, but existing approaches usually look at them in isolation. In this paper, we aim to predict the conversion response of users by jointly examining the past purchase behavior and the click response behavior. Additionally, we model the temporal dynamics between the click response and purchase activity into a unified framework. In particular, a novel matrix factorization approach named dynamic collective matrix factorization (DCMF) is proposed to address this problem. Our model considers temporal dynamics of post-click conversions and also takes advantages of the side information of users, advertisements, and items. Experiments on a real-world marketing dataset show that our model achieves significant improvements over several baselines.
更多查看译文
关键词
Conversion prediction,matrix factorization,temporal dynamic
AI 理解论文
溯源树
样例
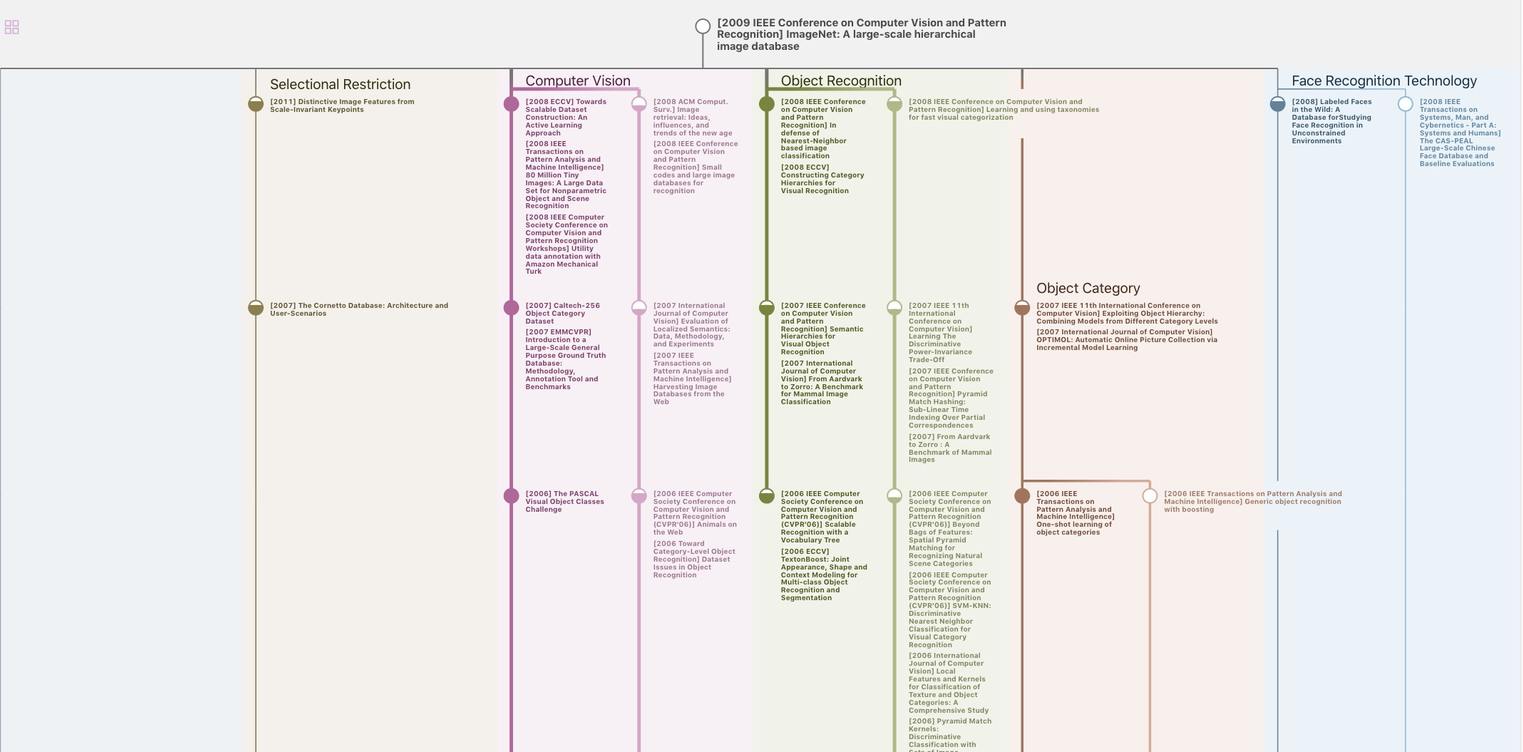
生成溯源树,研究论文发展脉络
Chat Paper
正在生成论文摘要