An Entity Class-Dependent Discriminative Mixture Model For Cumulative Citation Recommendation
SIGIR '15: The 38th International ACM SIGIR conference on research and development in Information Retrieval Santiago Chile August, 2015(2015)
摘要
This paper studies Cumulative Citation Recommendation (CCR) for Knowledge Base Acceleration (KBA). The CCR task aims to detect potential citations of a set of target entities with priorities from a volume of temporally-ordered stream corpus. Previous approaches for CCR that build an individual relevance model for each entity fail to handle unseen entities without annotation. A baseline solution is to build a global entity-unspecific model for all entities regardless of the relationship information among entities, which cannot guarantee to achieve satisfactory result for each entity. In this paper, we propose a novel entity class-dependent discriminative mixture model by introducing a latent entity class layer to model the correlations between entities and latent entity classes. The model can better adjust to different types of entities and achieve better performance when dealing with a broad range of entities. An extensive set of experiments has been conducted on TREC-KBA-2013 dataset, and the experimental results demonstrate that the proposed model can achieve the state-of-the-art performance.
更多查看译文
关键词
Cumulative Citation Recommendation,Knowledge Base Acceleration,Mixture Model,Information Filtering
AI 理解论文
溯源树
样例
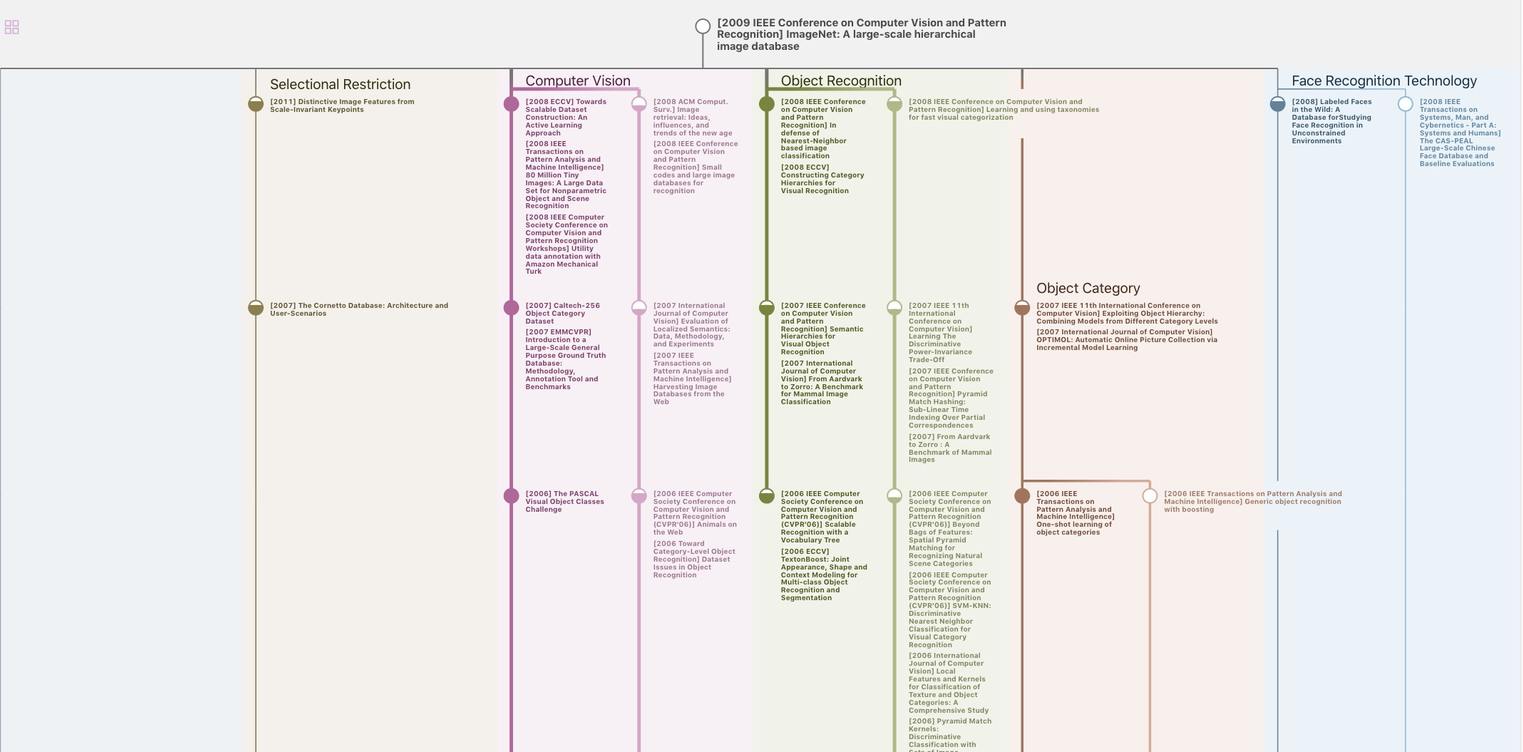
生成溯源树,研究论文发展脉络
Chat Paper
正在生成论文摘要