Inferring Searcher Attention By Jointly Modeling User Interactions And Content Salience
IR(2015)
摘要
Modeling and predicting user attention is crucial for interpreting search behavior. Current applications include quantifying web search satisfaction, estimating search quality, and measuring and predicting online user engagement. The most direct way to measure attention is through eye gaze tracking, which is not yet widely available. While prior research has demonstrated the value of mouse cursor data and other interactions as a rough proxy of user attention, accurately predicting where a user is looking on a page remains a challenge. This problem is exacerbated when moving beyond the traditional search result pages to other domains, where high diversity of content and visual presentation often affect how users examine a page. We posit that in order to accurately model user attention online, interaction signals should be grounded to the underlying content. To this end, we introduce a principled model to connect interaction signals with page content features, which we call Mixture of Interactions and Content Salience (MICS). To our knowledge, our model is the first to effectively combine user interaction data with visual prominence, or salience, of the page content elements. Extensive experiments on multiple popular types of Web content demonstrate that our model significantly outperforms previous approaches to searcher gaze prediction, which use only the interaction information. Grounding the observed interactions to the underlying page content provides a general and robust approach to user attention modeling, which could enable more powerful tools for search behavior interpretation, and ultimately search quality improvements.
更多查看译文
关键词
searcher attention,search behavior,content salience
AI 理解论文
溯源树
样例
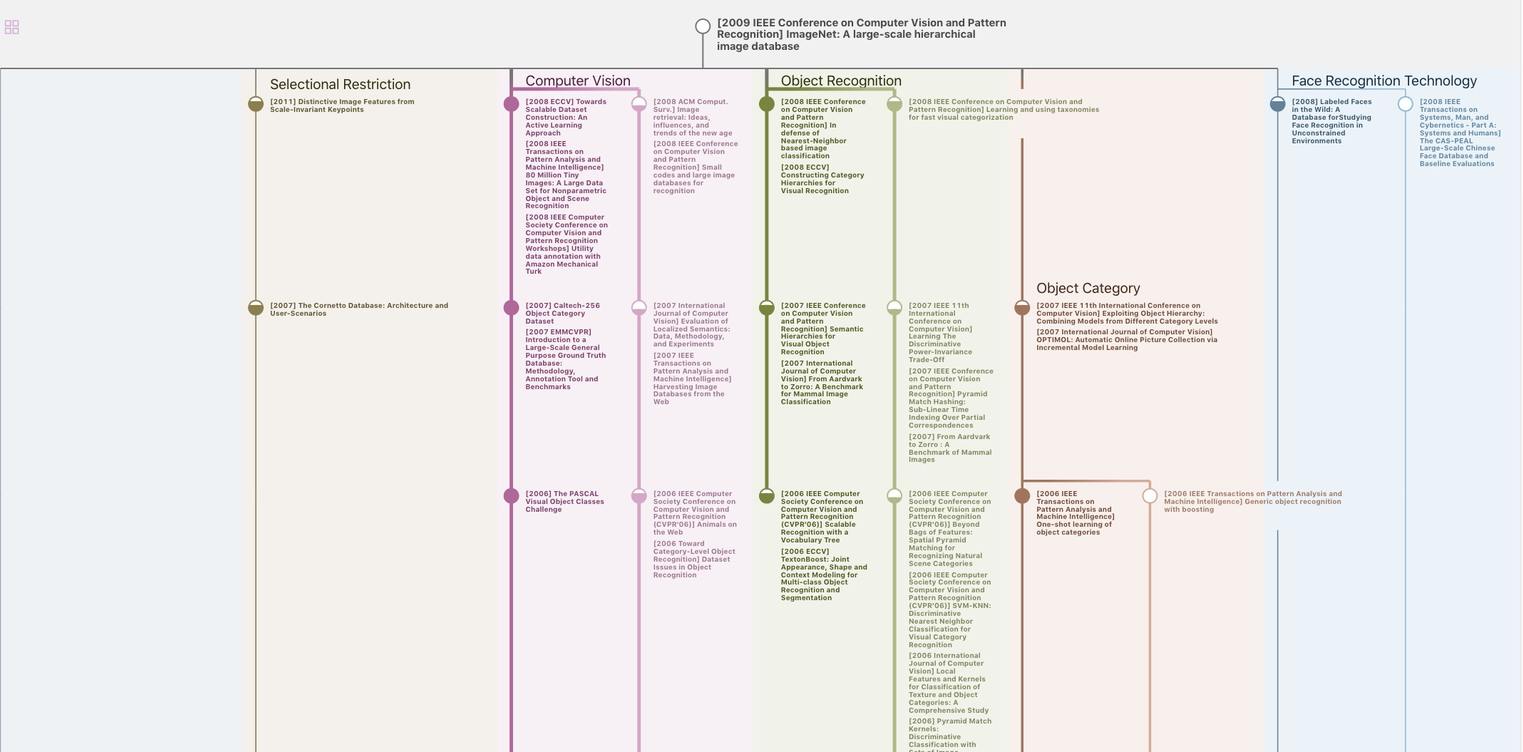
生成溯源树,研究论文发展脉络
Chat Paper
正在生成论文摘要